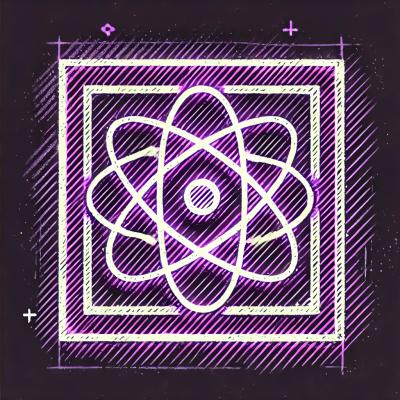
Security News
Create React App Officially Deprecated Amid React 19 Compatibility Issues
Create React App is officially deprecated due to React 19 issues and lack of maintenance—developers should switch to Vite or other modern alternatives.
AntiNex publisher-subscriber core for processing training and prediction requests for deep neural networks to detect network exploits using Keras and Tensorflow in near real-time.
Automating network exploit detection using highly accurate pre-trained deep neural networks.
As of 2018-03-12, the core can repeatedly predict attacks on Django, Flask, React + Redux, Vue, and Spring application servers by training using the pre-recorded AntiNex datasets
_ with cross validation scores above ~99.8% with automated scaler normalization.
.. image:: https://travis-ci.org/jay-johnson/antinex-core.svg?branch=master :target: https://travis-ci.org/jay-johnson/antinex-core
.. _AntiNex datasets: https://github.com/jay-johnson/antinex-datasets
The core is a Celery worker pool for processing training and prediction requests for deep neural networks to detect network exploits (Nex) using Keras and Tensorflow in near real-time. Internally each worker manages a buffer of pre-trained models identified by the label
from the initial training request. Once trained, a model can be used for rapid prediction testing provided the same label
name is used on the prediction request. Models can also be re-trained by using the training api with the same label
. While the initial focus is on network exploits, the repository also includes mock stock data for demonstrating running a worker pool to quickly predict regression data (like stock prices) with many, pre-trained deep neural networks.
This repository is a standalone training and prediction worker pool that is decoupled from the AntiNex REST API:
https://github.com/jay-johnson/train-ai-with-django-swagger-jwt
AntiNex Core Worker is part of the AntiNex stack:
.. list-table:: :header-rows: 1
REST API <https://github.com/jay-johnson/train-ai-with-django-swagger-jwt>
__Docs <http://antinex.readthedocs.io/en/latest/>
__Core Worker <https://github.com/jay-johnson/antinex-core>
__Docs <http://antinex-core-worker.readthedocs.io/en/latest/>
__Network Pipeline <https://github.com/jay-johnson/network-pipeline>
__Docs <http://antinex-network-pipeline.readthedocs.io/en/latest/>
__AI Utils <https://github.com/jay-johnson/antinex-utils>
__Docs <http://antinex-ai-utilities.readthedocs.io/en/latest/>
__Client <https://github.com/jay-johnson/antinex-client>
__Docs <http://antinex-client.readthedocs.io/en/latest/>
__pip install antinex-core
If you want to generate images please install python3-tk
on Ubuntu.
::
sudo apt-get install python3-tk
Start the container for browsing with Jupyter:
::
# if you do not have docker compose installed, you can try installing it with:
# pip install docker-compose
cd docker
./start-stack.sh
Default password is: admin
http://localhost:8888/notebooks/AntiNex-Protecting-Django.ipynb
#. Use Alt + r
inside the notebook
#. Use the non-vertical scolling url: http://localhost:8889/Slides-AntiNex-Protecting-Django.slides.html
#. Use the non-vertical scolling url: http://localhost:8890/Slides-AntiNex-Using-Pre-Trained-Deep-Neural-Networks-For-Defense.slides.html
Please make sure redis is running and accessible before starting the core:
::
redis-cli
127.0.0.1:6379>
With redis running and the antinex-core pip installed in the python 3 runtime, use this command to start the core:
::
./run-antinex-core.sh
Or with celery:
::
celery worker -A antinex_core.antinex_worker -l DEBUG
To train and predict with the new automated scaler-normalized dataset with a 99.8% prediction accuracy for detecting attacks using a wide, two-layer deep neural network with the AntiNex datasets
_ run the following steps.
.. _AntiNex datasets: https://github.com/jay-johnson/antinex-datasets
Please make sure to clone the dataset repo to the pre-configured location:
::
mkdir -p -m 777 /opt/antinex
git clone https://github.com/jay-johnson/antinex-datasets.git /opt/antinex/antinex-datasets
::
./antinex_core/scripts/publish_predict_request.py -f training/scaler-full-django-antinex-simple.json
::
./antinex_core/scripts/publish_predict_request.py -f training/scaler-full-flask-antinex-simple.json
::
./antinex_core/scripts/publish_predict_request.py -f training/scaler-full-react-redux-antinex-simple.json
::
./antinex_core/scripts/publish_predict_request.py -f training/scaler-full-vue-antinex-simple.json
::
./antinex_core/scripts/publish_predict_request.py -f training/scaler-full-spring-antinex-simple.json
After a few minutes the final report will be printed out like:
::
2018-03-11 23:35:00,944 - antinex-prc - INFO - sample=30178 - label_value=1.0 predicted=1 label=attack
2018-03-11 23:35:00,944 - antinex-prc - INFO - sample=30179 - label_value=-1.0 predicted=-1 label=not_attack
2018-03-11 23:35:00,944 - antinex-prc - INFO - sample=30180 - label_value=-1.0 predicted=-1 label=not_attack
2018-03-11 23:35:00,944 - antinex-prc - INFO - sample=30181 - label_value=-1.0 predicted=-1 label=not_attack
2018-03-11 23:35:00,944 - antinex-prc - INFO - sample=30182 - label_value=-1.0 predicted=-1 label=not_attack
2018-03-11 23:35:00,945 - antinex-prc - INFO - sample=30183 - label_value=-1.0 predicted=-1 label=not_attack
2018-03-11 23:35:00,945 - antinex-prc - INFO - sample=30184 - label_value=-1.0 predicted=-1 label=not_attack
2018-03-11 23:35:00,945 - antinex-prc - INFO - sample=30185 - label_value=-1.0 predicted=-1 label=not_attack
2018-03-11 23:35:00,945 - antinex-prc - INFO - sample=30186 - label_value=-1.0 predicted=-1 label=not_attack
2018-03-11 23:35:00,945 - antinex-prc - INFO - sample=30187 - label_value=-1.0 predicted=-1 label=not_attack
2018-03-11 23:35:00,945 - antinex-prc - INFO - sample=30188 - label_value=-1.0 predicted=-1 label=not_attack
2018-03-11 23:35:00,945 - antinex-prc - INFO - sample=30189 - label_value=1.0 predicted=1 label=attack
2018-03-11 23:35:00,945 - antinex-prc - INFO - sample=30190 - label_value=-1.0 predicted=-1 label=not_attack
2018-03-11 23:35:00,945 - antinex-prc - INFO - sample=30191 - label_value=-1.0 predicted=-1 label=not_attack
2018-03-11 23:35:00,946 - antinex-prc - INFO - sample=30192 - label_value=-1.0 predicted=-1 label=not_attack
2018-03-11 23:35:00,946 - antinex-prc - INFO - sample=30193 - label_value=-1.0 predicted=-1 label=not_attack
2018-03-11 23:35:00,946 - antinex-prc - INFO - sample=30194 - label_value=-1.0 predicted=-1 label=not_attack
2018-03-11 23:35:00,946 - antinex-prc - INFO - sample=30195 - label_value=-1.0 predicted=-1 label=not_attack
2018-03-11 23:35:00,946 - antinex-prc - INFO - sample=30196 - label_value=-1.0 predicted=-1 label=not_attack
2018-03-11 23:35:00,946 - antinex-prc - INFO - sample=30197 - label_value=-1.0 predicted=-1 label=not_attack
2018-03-11 23:35:00,946 - antinex-prc - INFO - sample=30198 - label_value=-1.0 predicted=-1 label=not_attack
2018-03-11 23:35:00,946 - antinex-prc - INFO - sample=30199 - label_value=-1.0 predicted=-1 label=not_attack
2018-03-11 23:35:00,947 - antinex-prc - INFO - Full-Django-AntiNex-Simple-Scaler-DNN made predictions=30200 found=30200 accuracy=99.84685430463577
2018-03-11 23:35:00,947 - antinex-prc - INFO - Full-Django-AntiNex-Simple-Scaler-DNN - saving model=full-django-antinex-simple-scaler-dnn
If you do not have the datasets cloned locally, you can use the included minimized dataset from the repo:
::
./antinex_core/scripts/publish_predict_request.py -f training/scaler-django-antinex-simple.json
::
./antinex_core/scripts/publish_train_request.py
::
./antinex_core/scripts/publish_regression_predict.py
The AntiNex core manages a pool of workers that are subscribed to process tasks found in two queues (webapp.train.requests
and webapp.predict.requests
). Tasks are defined as JSON dictionaries and must have the following structure:
::
{
"label": "Django-AntiNex-Simple-Scaler-DNN",
"dataset": "./tests/datasets/classification/cleaned_attack_scans.csv",
"apply_scaler": true,
"ml_type": "classification",
"predict_feature": "label_value",
"features_to_process": [
"eth_type",
"idx",
"ip_ihl",
"ip_len",
"ip_tos",
"ip_version",
"tcp_dport",
"tcp_fields_options.MSS",
"tcp_fields_options.Timestamp",
"tcp_fields_options.WScale",
"tcp_seq",
"tcp_sport"
],
"ignore_features": [
],
"sort_values": [
],
"seed": 42,
"test_size": 0.2,
"batch_size": 32,
"epochs": 10,
"num_splits": 2,
"loss": "binary_crossentropy",
"optimizer": "adam",
"metrics": [
"accuracy"
],
"histories": [
"val_loss",
"val_acc",
"loss",
"acc"
],
"model_desc": {
"layers": [
{
"num_neurons": 250,
"init": "uniform",
"activation": "relu"
},
{
"num_neurons": 1,
"init": "uniform",
"activation": "sigmoid"
}
]
},
"label_rules": {
"labels": [
"not_attack",
"not_attack",
"attack"
],
"label_values": [
-1,
0,
1
]
},
"version": 1
}
Regression prediction tasks are also supported, and here is an example from an included dataset with mock stock prices:
::
{
"label": "Scaler-Close-Regression",
"dataset": "./tests/datasets/regression/stock.csv",
"apply_scaler": true,
"ml_type": "regression",
"predict_feature": "close",
"features_to_process": [
"high",
"low",
"open",
"volume"
],
"ignore_features": [
],
"sort_values": [
],
"seed": 7,
"test_size": 0.2,
"batch_size": 32,
"epochs": 50,
"num_splits": 2,
"loss": "mse",
"optimizer": "adam",
"metrics": [
"accuracy"
],
"model_desc": {
"layers": [
{
"activation": "relu",
"init": "uniform",
"num_neurons": 200
},
{
"activation": null,
"init": "uniform",
"num_neurons": 1
}
]
}
}
This repository uses the Spylunking <https://github.com/jay-johnson/spylunking>
__ logger that supports publishing logs to Splunk over the authenticated HEC REST API. You can set these environment variables to publish to Splunk:
::
export SPLUNK_ADDRESS="<splunk address host:port>"
export SPLUNK_API_ADDRESS="<splunk api address host:port>"
export SPLUNK_USER="<splunk username for login>"
export SPLUNK_PASSWORD="<splunk password for login>"
export SPLUNK_TOKEN="<Optional - username and password will login or you can use a pre-existing splunk token>"
export SPLUNK_INDEX="<splunk index>"
export SPLUNK_QUEUE_SIZE="<num msgs allowed in queue - 0=infinite>"
export SPLUNK_RETRY_COUNT="<attempts per log to retry publishing>"
export SPLUNK_RETRY_BACKOFF="<cooldown in seconds per failed POST>"
export SPLUNK_SLEEP_INTERVAL="<sleep in seconds per batch>"
export SPLUNK_SOURCE="<splunk source>"
export SPLUNK_SOURCETYPE="<splunk sourcetype>"
export SPLUNK_TIMEOUT="<timeout in seconds>"
export SPLUNK_DEBUG="<1 enable debug|0 off - very verbose logging in the Splunk Publishers>"
::
virtualenv -p python3 ~/.venvs/antinexcore && source ~/.venvs/antinexcore/bin/activate && pip install -e .
Run all
::
python setup.py test
Run a test case
::
python -m unittest tests.test_train.TestTrain.test_train_antinex_simple_success_retrain
flake8 .
pycodestyle .
Apache 2.0 - Please refer to the LICENSE_ for more details
.. _License: https://github.com/jay-johnson/antinex-core/blob/master/LICENSE
FAQs
AntiNex publisher-subscriber core for processing training and prediction requests for deep neural networks to detect network exploits using Keras and Tensorflow in near real-time.
We found that antinex-core demonstrated a healthy version release cadence and project activity because the last version was released less than a year ago. It has 1 open source maintainer collaborating on the project.
Did you know?
Socket for GitHub automatically highlights issues in each pull request and monitors the health of all your open source dependencies. Discover the contents of your packages and block harmful activity before you install or update your dependencies.
Security News
Create React App is officially deprecated due to React 19 issues and lack of maintenance—developers should switch to Vite or other modern alternatives.
Security News
Oracle seeks to dismiss fraud claims in the JavaScript trademark dispute, delaying the case and avoiding questions about its right to the name.
Security News
The Linux Foundation is warning open source developers that compliance with global sanctions is mandatory, highlighting legal risks and restrictions on contributions.