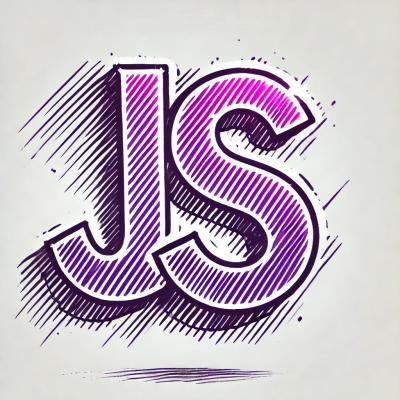
Security News
Oracle Drags Its Feet in the JavaScript Trademark Dispute
Oracle seeks to dismiss fraud claims in the JavaScript trademark dispute, delaying the case and avoiding questions about its right to the name.
Phoenix provides tooling to evaluate LLM applications, including tools to determine the relevance or irrelevance of documents retrieved by retrieval-augmented generation (RAG) application, whether or not the response is toxic, and much more.
Phoenix's approach to LLM evals is notable for the following reasons:
Install the arize-phoenix sub-package via pip
pip install arize-phoenix-evals
Note you will also have to install the LLM vendor SDK you would like to use with LLM Evals. For example, to use OpenAI's GPT-4, you will need to install the OpenAI Python SDK:
pip install 'openai>=1.0.0'
Here is an example of running the RAG relevance eval on a dataset of Wikipedia questions and answers:
import os
from phoenix.evals import (
RAG_RELEVANCY_PROMPT_TEMPLATE,
RAG_RELEVANCY_PROMPT_RAILS_MAP,
OpenAIModel,
download_benchmark_dataset,
llm_classify,
)
from sklearn.metrics import precision_recall_fscore_support, confusion_matrix, ConfusionMatrixDisplay
os.environ["OPENAI_API_KEY"] = "<your-openai-key>"
# Download the benchmark golden dataset
df = download_benchmark_dataset(
task="binary-relevance-classification", dataset_name="wiki_qa-train"
)
# Sample and re-name the columns to match the template
df = df.sample(100)
df = df.rename(
columns={
"query_text": "input",
"document_text": "reference",
},
)
model = OpenAIModel(
model="gpt-4",
temperature=0.0,
)
rails =list(RAG_RELEVANCY_PROMPT_RAILS_MAP.values())
df[["eval_relevance"]] = llm_classify(df, model, RAG_RELEVANCY_PROMPT_TEMPLATE, rails)
#Golden dataset has True/False map to -> "irrelevant" / "relevant"
#we can then scikit compare to output of template - same format
y_true = df["relevant"].map({True: "relevant", False: "irrelevant"})
y_pred = df["eval_relevance"]
# Compute Per-Class Precision, Recall, F1 Score, Support
precision, recall, f1, support = precision_recall_fscore_support(y_true, y_pred)
To learn more about LLM Evals, see the LLM Evals documentation.
FAQs
LLM Evaluations
We found that arize-phoenix-evals demonstrated a healthy version release cadence and project activity because the last version was released less than a year ago. It has 1 open source maintainer collaborating on the project.
Did you know?
Socket for GitHub automatically highlights issues in each pull request and monitors the health of all your open source dependencies. Discover the contents of your packages and block harmful activity before you install or update your dependencies.
Security News
Oracle seeks to dismiss fraud claims in the JavaScript trademark dispute, delaying the case and avoiding questions about its right to the name.
Security News
The Linux Foundation is warning open source developers that compliance with global sanctions is mandatory, highlighting legal risks and restrictions on contributions.
Security News
Maven Central now validates Sigstore signatures, making it easier for developers to verify the provenance of Java packages.