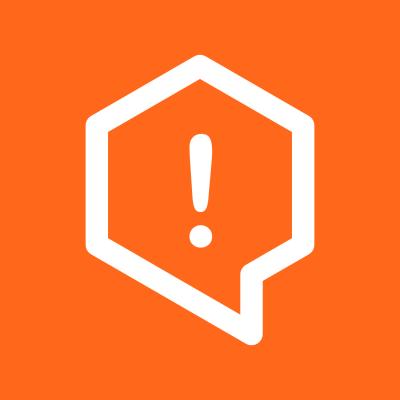
Security News
Fluent Assertions Faces Backlash After Abandoning Open Source Licensing
Fluent Assertions is facing backlash after dropping the Apache license for a commercial model, leaving users blindsided and questioning contributor rights.
Bagua is a deep learning training acceleration framework for PyTorch. It provides a one-stop training acceleration solution, including faster distributed training compared to PyTorch DDP, faster dataloader, kernel fusion, and more.
WARNING: THIS PROJECT IS CURRENTLY IN MAINTENANCE MODE, DUE TO COMPANY REORGANIZATION.
Bagua is a deep learning training acceleration framework for PyTorch developed by AI platform@Kuaishou Technology and DS3 Lab@ETH Zürich. Bagua currently supports:
.step()
operation on multiple layers. It can be applied to arbitrary PyTorch optimizer, in contrast to NVIDIA Apex's approach, where only some specific optimizers are implemented.strategy=BaguaStrategy
in your Trainer. This enables you to take advantage of a range of advanced training algorithms, including decentralized methods, asynchronous methods, communication compression, and their combinations!Its effectiveness has been evaluated in various scenarios, including VGG and ResNet on ImageNet, BERT Large and many industrial applications at Kuaishou.
The performance of different systems and algorithms on VGG16 with 128 GPUs under different network bandwidth.
Epoch time of BERT-Large Finetune under different network conditions for different systems.
For more comprehensive and up to date results, refer to Bagua benchmark page.
Wheels (precompiled binary packages) are available for Linux (x86_64). Package names are different depending on your CUDA Toolkit version (CUDA Toolkit version is shown in nvcc --version
).
CUDA Toolkit version | Installation command |
---|---|
>= v10.2 | pip install bagua-cuda102 |
>= v11.1 | pip install bagua-cuda111 |
>= v11.3 | pip install bagua-cuda113 |
>= v11.5 | pip install bagua-cuda115 |
>= v11.6 | pip install bagua-cuda116 |
Add --pre
to pip install
commands to install pre-release (development) versions. See Bagua tutorials for quick start guide and more installation options.
Thanks to the Amazon Machine Images (AMI), we can provide users an easy way to deploy and run Bagua on AWS EC2 clusters with flexible size of machines and a wide range of GPU types. Users can find our pre-installed Bagua image on EC2 by the unique AMI-ID that we publish here. Note that AMI is a regional resource, so please make sure you are using the machines in same reginon as our AMI.
Bagua version | AMI ID | Region |
---|---|---|
0.6.3 | ami-0e719d0e3e42b397e | us-east-1 |
0.9.0 | ami-0f01fd14e9a742624 | us-east-1 |
To manage the EC2 cluster more efficiently, we use Starcluster as a toolkit to manipulate the cluster. In the config
file of Starcluster, there are a few configurations that need to be set up by users, including AWS credentials, cluster settings, etc. More information regarding the Starcluster configuration can be found in this tutorial.
For example, we create a EC2 cluster with 4 machines, each of which has 8 V100 GPUs (p3.16xlarge
). The cluster is based on the Bagua AMI we pre-installed in us-east-1
region. Then the config
file of Starcluster would be:
# region of EC2 instances, here we choose us_east_1
AWS_REGION_NAME = us-east-1
AWS_REGION_HOST = ec2.us-east-1.amazonaws.com
# AMI ID of Bagua
NODE_IMAGE_ID = ami-0e719d0e3e42b397e
# number of instances
CLUSTER_SIZE = 4
# instance type
NODE_INSTANCE_TYPE = p3.16xlarge
With above setup, we created two identical clusters to benchmark a synthesized image classification task over Bagua and Horovod, respectively. Here is the screen recording video of this experiment.
% System Overview
@misc{gan2021bagua,
title={BAGUA: Scaling up Distributed Learning with System Relaxations},
author={Shaoduo Gan and Xiangru Lian and Rui Wang and Jianbin Chang and Chengjun Liu and Hongmei Shi and Shengzhuo Zhang and Xianghong Li and Tengxu Sun and Jiawei Jiang and Binhang Yuan and Sen Yang and Ji Liu and Ce Zhang},
year={2021},
eprint={2107.01499},
archivePrefix={arXiv},
primaryClass={cs.LG}
}
% Theory on System Relaxation Techniques
@book{liu2020distributed,
title={Distributed Learning Systems with First-Order Methods: An Introduction},
author={Liu, J. and Zhang, C.},
isbn={9781680837018},
series={Foundations and trends in databases},
url={https://books.google.com/books?id=vzQmzgEACAAJ},
year={2020},
publisher={now publishers}
}
FAQs
Bagua is a deep learning training acceleration framework for PyTorch. It provides a one-stop training acceleration solution, including faster distributed training compared to PyTorch DDP, faster dataloader, kernel fusion, and more.
We found that bagua-cuda113 demonstrated a healthy version release cadence and project activity because the last version was released less than a year ago. It has 1 open source maintainer collaborating on the project.
Did you know?
Socket for GitHub automatically highlights issues in each pull request and monitors the health of all your open source dependencies. Discover the contents of your packages and block harmful activity before you install or update your dependencies.
Security News
Fluent Assertions is facing backlash after dropping the Apache license for a commercial model, leaving users blindsided and questioning contributor rights.
Research
Security News
Socket researchers uncover the risks of a malicious Python package targeting Discord developers.
Security News
The UK is proposing a bold ban on ransomware payments by public entities to disrupt cybercrime, protect critical services, and lead global cybersecurity efforts.