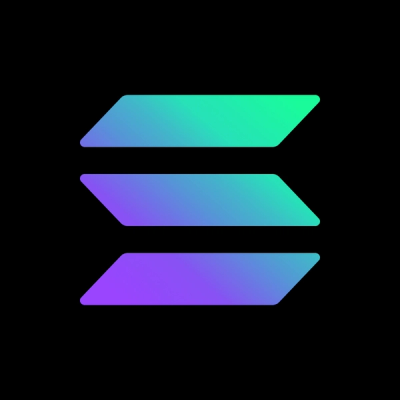
Security News
Supply Chain Attack Detected in Solana's web3.js Library
A supply chain attack has been detected in versions 1.95.6 and 1.95.7 of the popular @solana/web3.js library.
Machine learning for animal behavior.
Interprets pose-tracking files (currently only from DLC) and behavior annotations (currently only from BORIS) to train a behavior classifier, perform unsupervised learning, and other common analysis tasks.
pip install behaveml
Can install optional extras with:
pip install numpy, cython
pip install behaveml[all]
This includes matplotlib, keras, and Linderman lab's state-space model package, ssm. Note that installing ssm requires cython and numpy for the build, so must be already present in the environment.
Import
from glob import glob
from behaveml import VideosetDataFrame, clone_metadata
from behaveml import compute_dl_probability_features, compute_mars_features
from behaveml.io import get_sample_data_paths
Gather the DLC and BORIS tracking and annotation files
tracking_files, boris_files = get_sample_data_paths()
Setup some parameters
frame_width = 20 # (float) length of entire horizontal shot
frame_width_units = 'in' # (str) units frame_width is given in
fps = 30 # (int) frames per second
resolution = (1200, 1600) # (tuple) HxW in pixels
Create a parameter object and video dataset
metadata = clone_metadata(tracking_files,
label_files = boris_files,
frame_width = frame_width,
fps = fps,
frame_width_units = frame_width_units,
resolution = resolution)
animal_renamer = {'adult': 'resident', 'juvenile':'intruder'}
dataset = VideosetDataFrame(metadata, animal_renamer=animal_renamer)
Now create features on this dataset
dataset.add_features(compute_dl_probability_features,
featureset_name = '1dcnn',
add_to_features = True)
dataset.add_features(compute_mars_features,
featureset_name = 'MARS',
add_to_features = True)
Now access a features table, labels, and groups for learning with dataset.features, dataset.labels, dataset.groups
. From here it's easy to use some ML libraries to predict behavior. For example:
from sklearn.ensemble import ExtraTreesClassifier, RandomForestClassifier
from sklearn.model_selection import cross_val_predict
from sklearn.metrics import accuracy_score
model = RandomForestClassifier()
predictions = cross_val_predict(model, dataset.features, dataset.labels, dataset.groups)
score = accuracy_score(dataset.labels, predictions)
FAQs
Machine learning for animal behavior analysis
We found that behaveml demonstrated a healthy version release cadence and project activity because the last version was released less than a year ago. It has 1 open source maintainer collaborating on the project.
Did you know?
Socket for GitHub automatically highlights issues in each pull request and monitors the health of all your open source dependencies. Discover the contents of your packages and block harmful activity before you install or update your dependencies.
Security News
A supply chain attack has been detected in versions 1.95.6 and 1.95.7 of the popular @solana/web3.js library.
Research
Security News
A malicious npm package targets Solana developers, rerouting funds in 2% of transactions to a hardcoded address.
Security News
Research
Socket researchers have discovered malicious npm packages targeting crypto developers, stealing credentials and wallet data using spyware delivered through typosquats of popular cryptographic libraries.