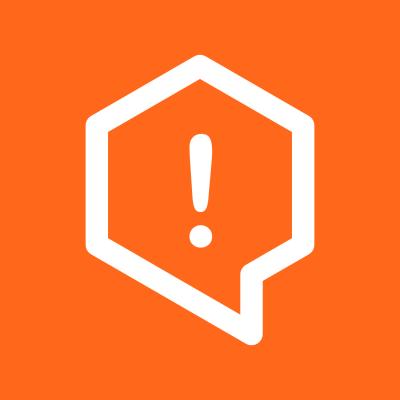
Security News
Fluent Assertions Faces Backlash After Abandoning Open Source Licensing
Fluent Assertions is facing backlash after dropping the Apache license for a commercial model, leaving users blindsided and questioning contributor rights.
EntityGym is a Python library that defines a novel entity-based abstraction for reinforcement learning environments which enables highly ergonomic and efficient training of deep reinforcement learning agents. This crate provides bindings that allows Rust programs to be used as EntityGym training environments, and to load and run neural networks agents trained with Entity Neural Network Trainer natively in pure Rust applications.
The core abstraction in entity-gym-rs is the Agent
trait.
It defines a high-level API for neural network agents which allows them to directly interact with Rust data structures.
To use any of the Agent
implementations provided by entity-gym-rs, you just need to derive the Action
and Featurizable
traits, which define what information the agent can observe and what actions it can take:
Action
trait allows a Rust type to be returned as an action by an Agent
. This trait can be derived automatically for enums with only unit variants.Featurizable
trait converts objects into a format that can be processed by neural networks. It can be derived for most fixed-size struct
s, and for enum
s with unit variants. Agent
s can observe collections containing any number of Featurizable
objects.Basic example that demonstrates how to construct an observation and sample a random action from an Agent
:
use entity_gym_rs::agent::{Agent, AgentOps, Obs, Action, Featurizable};
#[derive(Action, Debug)]
enum Move { Up, Down, Left, Right }
#[derive(Featurizable)]
struct Player { x: i32, y: i32 }
#[derive(Featurizable)]
struct Cake {
x: i32,
y: i32,
size: u32,
}
fn main() {
// Creates an agent that acts completely randomly.
let mut agent = Agent::random();
// Alternatively, load a trained neural network agent from a checkpoint.
// let mut agent = Agent::load("agent");
// Construct an observation with one `Player` entity and two `Cake entities.
let obs = Obs::new(0.0)
.entities([Player { x: 0, y: 0 }])
.entities([
Cake { x: 4, y: 0, size: 4 },
Cake { x: 10, y: 42, size: 12 },
]);
// To obtain an action from an agent, we simple call the `act` method
// with the observation we constructed.
let action = agent.act::<Move>(obs);
println!("{:?}", action);
}
For a more complete example that includes training a neural network to play Snake, see examples/bevy_snake.
FAQs
Rust bindings for the entity-gym library
We found that entity-gym-rs demonstrated a healthy version release cadence and project activity because the last version was released less than a year ago. It has 1 open source maintainer collaborating on the project.
Did you know?
Socket for GitHub automatically highlights issues in each pull request and monitors the health of all your open source dependencies. Discover the contents of your packages and block harmful activity before you install or update your dependencies.
Security News
Fluent Assertions is facing backlash after dropping the Apache license for a commercial model, leaving users blindsided and questioning contributor rights.
Research
Security News
Socket researchers uncover the risks of a malicious Python package targeting Discord developers.
Security News
The UK is proposing a bold ban on ransomware payments by public entities to disrupt cybercrime, protect critical services, and lead global cybersecurity efforts.