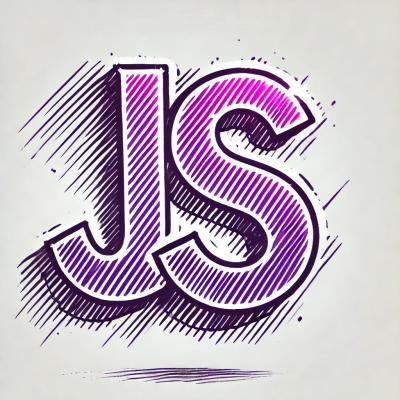
Security News
Oracle Drags Its Feet in the JavaScript Trademark Dispute
Oracle seeks to dismiss fraud claims in the JavaScript trademark dispute, delaying the case and avoiding questions about its right to the name.
Pytorch-based library to initialize any neural network with the gradient descent and your dataset. This implementation is the simple way to use the method described in the paper Chen Zhu et al. GradInit: Learning to Initialize Neural Networks for Stable and Efficient Training
Gradinit may be used as the alternative to the warmup mechanism. It is more useful for deep or unusual architectures.
pip install gradinit
or
pip install --upgrade git+https://github.com/johngull/gradinit.git
This implementation uses simplified loss in the case of the small gradients.
in this case, gradinit
uses the user's loss instead of the complex 2-stage procedure described in the paper.
To make gradinit you need to wrap your model with the GradInitWrapper
and then you can use the usual training loop with some differences:
GradInitWrapper.grad_loss
functionGradInitWrapper.clamp_scales
functionHere is a simplified example of the full gradinit process:
model: torch.nn.Module = ...
with GradInitWrapper(model) as ginit:
#it is important to create optimizer after wraping your model
optimizer = torch.optim.Adam(model.parameters())
norm = 1 # use 2 for the SGD optimizer
model.train()
for x, y in data_loader:
pred = model(x)
loss = criterion(pred, y)
# recalculate gradinit loss based on your loss
loss = ginit.grad_loss(loss, norm)
optimizer.zero_grad()
loss.backward()
optimizer.step()
# clip minimum values for the init scales
ginit.clamp_scales()
# on exit of with statement model is recovered to its normal way
# here you should create your main optimizer and start training
optimizer = torch.optim.Adam(model.parameters())
...
Alternatively to the with
-notation
you may use detach
or delete the wrapper object after finishing the initialization process.
ginit = GradInitWrapper(model)
# full gradinit loop
...
ginit.detach() # or del ginit
# here you should create your main optimizer and start training
optimizer = torch.optim.Adam(model.parameters())
...
From our experience gradinit works worse in the mixed-precision mode. And we recommend running gradinit in the full-precision mode and then starting the main training loop in mixed-precision.
But if you really want to try, gradinit supports torch mixed-precision. In such a case gradinit need to use your scaler object. Here is an example of how to use gradinit with the torch amp.
import torch.cuda.amp
model: torch.nn.Module = ...
scaler: torch.cuda.amp.GradScaler = ...
with GradInitWrapper(model) as ginit:
# it is important to create optimizer after wraping your model
optimizer = torch.optim.Adam(model.parameters())
norm = 1 # use 2 for the SGD optimizer
model.train()
for x, y in data_loader:
with autocast():
pred = model(x)
loss = criterion(pred, y)
# recalculate gradinit loss based on your loss
loss = ginit.grad_loss(loss, norm, scaler=scaler)
optimizer.zero_grad()
scaler.scale(loss).backward()
scaler.step(optimizer)
scaler.update()
# clip minimum values for the init scales
ginit.clamp_scales()
# on exit of with statement model is recovered to its normal way
# here you should create your main optimizer and start training
optimizer = torch.optim.Adam(model.parameters())
...
For the experiment results see this page
FAQs
Pytorch implementation of the gradient-based initialization
We found that gradinit demonstrated a healthy version release cadence and project activity because the last version was released less than a year ago. It has 1 open source maintainer collaborating on the project.
Did you know?
Socket for GitHub automatically highlights issues in each pull request and monitors the health of all your open source dependencies. Discover the contents of your packages and block harmful activity before you install or update your dependencies.
Security News
Oracle seeks to dismiss fraud claims in the JavaScript trademark dispute, delaying the case and avoiding questions about its right to the name.
Security News
The Linux Foundation is warning open source developers that compliance with global sanctions is mandatory, highlighting legal risks and restrictions on contributions.
Security News
Maven Central now validates Sigstore signatures, making it easier for developers to verify the provenance of Java packages.