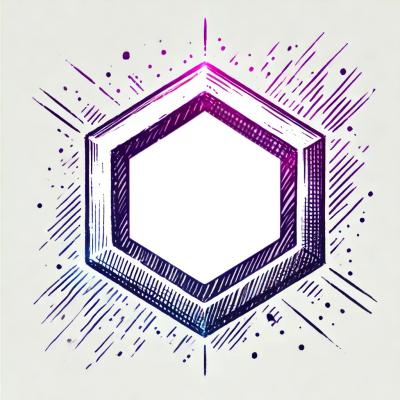
Security News
Maven Central Adds Sigstore Signature Validation
Maven Central now validates Sigstore signatures, making it easier for developers to verify the provenance of Java packages.
SQLite3-backed JSON document database with support for indices and advanced queries
SQLite3-backed JSON document database with support for indices and advanced queries.
JSONLiteDB leverages SQLite3 and JSON1 to create a fast JSON document store with easy persistence, indexing capability, and extensible use.
JSONLiteDB provides an easy API with no need to load the entire database into memory, nor dump it when inserting! JSONLiteDB SQLite files are easily usable in other tools with no proprietary formats or encoding. JSONLiteDB is a great replacement for reading a JSON or JSONLines file. Entries can be modified in place. Queries can be indexed for greatly improved query speed and optionally to enforce uniqueness.
Similar tools and inspiration:
TinyDB. The API and process of TinyDB heavily inspired JSONLiteDB. But TinyDB reads the entire JSON DB into memory and needs to dump the entire database upon insertion. Hardly efficient or scalable and still queries at O(N).
Dataset is promising but creates new columns for every key and is very "heavy" with its dependencies. As far as I can tell, there is no native way to support multi-column and/or unique indexes. But still, a very promising tool!
KenobiDB. Came out while JSONLiteDB was in development. Similar idea with different design decisions. Does not directly support advanced queries indexes (which can greatly accelerate queries!).
DictTable (also written by me) is nice but entirely in-memory and not always efficient for non-equality queries.
From PyPI:
$ pip install jsonlitedb
$ pip install jsonlitedb --upgrade
Or directly from Github
$ pip install git+https://github.com/Jwink3101/jsonlitedb.git
With some fake data.
>>> from jsonlitedb import JSONLiteDB
>>> db = JSONLiteDB(":memory:")
>>> # more generally:
>>> # db = JSONLiteDB('my_data.db')
Insert some data. Can use insert()
with any number of items or insertmany()
with an iterable (insertmany([...]) <--> insert(*[...])
).
Can also use a context manager (with db: ...
)to batch the insertions (or deletions).
>>> db.insert(
>>> {"first": "John", "last": "Lennon", "born": 1940, "role": "guitar"},
>>> {"first": "Paul", "last": "McCartney", "born": 1942, "role": "bass"},
>>> {"first": "George", "last": "Harrison", "born": 1943, "role": "guitar"},
>>> {"first": "Ringo", "last": "Starr", "born": 1940, "role": "drums"},
>>> {"first": "George", "last": "Martin", "born": 1926, "role": "producer"},
>>> )
>>> len(db)
5
>>> list(db)
[{'first': 'John', 'last': 'Lennon', 'born': 1940, 'role': 'guitar'},
{'first': 'Paul', 'last': 'McCartney', 'born': 1942, 'role': 'bass'},
{'first': 'George', 'last': 'Harrison', 'born': 1943, 'role': 'guitar'},
{'first': 'Ringo', 'last': 'Starr', 'born': 1940, 'role': 'drums'},
{'first': 'George', 'last': 'Martin', 'born': 1926, 'role': 'producer'}]
Let's do some simple queries. The default query()
returns an iterator so we wrap them in a list.
>>> list(db.query(first="George"))
[{'first': 'George', 'last': 'Harrison', 'born': 1943, 'role': 'guitar'},
{'first': 'George', 'last': 'Martin', 'born': 1926, 'role': 'producer'}]
>>> list(db.query(first="George", last="Martin"))
[{'first': 'George', 'last': 'Martin', 'born': 1926, 'role': 'producer'}]
Now let's query with a dictionary to match
>>> list(db.query({"first": "George"}))
[{'first': 'George', 'last': 'Harrison', 'born': 1943, 'role': 'guitar'},
{'first': 'George', 'last': 'Martin', 'born': 1926, 'role': 'producer'}]
Multiples are always an AND query
>>> list(db.query({"first": "George", "last": "Martin"}))
[{'first': 'George', 'last': 'Martin', 'born': 1926, 'role': 'producer'}]
Can do seperate items but it makes no difference.
>>> list(db.query({"first": "George"}, {"last": "Martin"}))
[{'first': 'George', 'last': 'Martin', 'born': 1926, 'role': 'producer'}]
Query objects enable more complex combinations and inequalities. Query objects can be from the database (db.Query
or db.Q
) or created on thier own (Query()
or Q()
). They are all the same.
>>> list(db.query(db.Q.first == "George"))
[{'first': 'George', 'last': 'Harrison', 'born': 1943, 'role': 'guitar'},
{'first': 'George', 'last': 'Martin', 'born': 1926, 'role': 'producer'}]
Note that you need to be careful with parentheses as the operator precedance for the &
and |
are very high
>>> list(db.query((db.Q.first == "George") & (db.Q.last == "Martin")))
[{'first': 'George', 'last': 'Martin', 'born': 1926, 'role': 'producer'}]
Can do inequalities too
>>> list(db.query(db.Q.born < 1930))
[{'first': 'George', 'last': 'Martin', 'born': 1926, 'role': 'producer'}]
Queries support: ==
, !=
, <
, <=
, >
, >=
for normal comparisons.
In addition they support
%
: LIKE
*
: GLOB
@
: REGEXP
using Python's regex module>>> # This will all be the same
>>> db.query(db.Q.role % "prod%").all() # LIKE
>>> db.query(db.Q.role * "prod*").all() # GLOB
>>> db.query(db.Q.role @ "prod").all() # REGEXP -- Python based
[{'first': 'George', 'last': 'Martin', 'born': 1926, 'role': 'producer'}]
Queries can be greatly accelerated with an index. Note that SQLite is extremely picky about how you write the index! For the most part, if you the same method to query as write the index, you will be fine. (This is more of an issue with nested queries and advanced formulating of the query).
The name of the index is imaterial. It is based on the fields. It will look different
>>> db.create_index("last")
>>> db.indexes
{'ix_items_1bd45eb5': ['$."last"']}
>>> # of course, with four items, this makes little difference
>>> list(db.query(last="Martin"))
[{'first': 'George', 'last': 'Martin', 'born': 1926, 'role': 'producer'}]
And an index can also be used to enforce uniqueness amongst one or more fields
>>> db.create_index("first", "last", unique=True)
>>> db.indexes
{'ix_items_1bd45eb5': ['$."last"'],
'ix_items_250e4243_UNIQUE': ['$."first"', '$."last"']}
>>> # db.insert({'first': 'George', 'last': 'Martin', 'type':'FAKE ENTRY'})
>>> # Causes: IntegrityError: UNIQUE constraint failed: index 'ix_items_250e4243_UNIQUE'
See Advanced Usage for more examples including nested queries
>>>
Queries are detailed in the db.query()
method. All queries and paths can take four basic forms, but query objects are, by far, the most versatile.
Type | Path (e.g. create_index() ) | Query (e.g. query() ) | Comments |
---|---|---|---|
Plain string | 'itemkey'
| {'itemkey':'query_val'} | Limited to a single item |
JSON Path string |
'$.itemkey'
'$.itemkey.subkey'
'$.itemkey[4]'
'$.itemkey.subkey[4]'
|
{'$.itemkey':'query_val'}
{'$.itemkey.subkey':'query_val'}
{'$.itemkey[4]':'query_val'}
{'$.itemkey.subkey[4]':'query_val'}
| Be careful about indices on JSON path strings. See more below |
Tuples (or lists) |
('itemkey',)
('itemkey','subkey')
('itemkey',4)
('itemkey','subkey',4)
|
{('itemkey',):'query_val'}
{('itemkey','subkey'):'query_val'}
{('itemkey',4):'query_val'}
{('itemkey','subkey',4):'query_val'}
| |
Query Objects. (Let db be your database) |
db.Q.itemkey
db.Q.itemkey.subkey
db.Q.itemkey[4]
db.Q.itemkey.subkey[4]
|
db.Q.itemkey == 'query_val'
db.Q.itemkey.subkey == 'query_val'
db.Q.itemkey[4] == 'query_val'
db.Q.itemkey.subkey[4] == 'query_val'
| See below. Can also do many more types of comparisons beyond equality |
Note that JSON Path strings presented here are unquoted, but all other methods will quote them. For example, '$.itemkey.subkey'
and ('itemkey','subkey')
are functionally identical; the latter becomes '$."itemkey"."subkey"'
. While they are functionally the same, an index created on one will not be used on the other.
Query Objects provide a great deal more flexibility than other forms.
They can handle normal equality ==
but can handle inequalities, including !=
, <
, <=
, >
, >=
.
db.Q.item < 10
db.Q.other_item > 'bla'
They can also handle logic. Note that you must be very careful about parentheses.
(db.Q.item < 10) & (db.Q.other_item > 'bla') # AND
(db.Q.item < 10) | (db.Q.other_item > 'bla') # OR
Note that while something like 10 <= var <= 20
is valid Python, a query must be done like:
(10 <= db.Q.var) & (db.Q.var <= 20 )
And, as noted in "Basic Usage," they can do SQL LIKE
comparisons (db.Q.key % "%Val%"
), GLOB
comparisons (db.Q.key * "file*.txt"
), and REGEXP
comparisons (db.Q.key @ "\S+?\.[A-Z]"
).
You can mix and match index or attribute for keys. The following are all identical:
db.Q.itemkey.subkey
db.Q['itemkey'].subkey
db.Q['itemkey','subkey']
db.Q['itemkey']['subkey']
JSONLiteDB also installs a tool called "jsonlitedb" that makes it easy to read JSONL and JSON files into a database. This is useful for converting existing databases or appending data.
$ jsonlitedb insert mydb.db newfile.jsonl
$ cat newdata.jsonl | jsonlitedb insert mydb.db
It can also dump a database to JSONL.
$ jsonlitedb dump mydb.db # stdout
$ jsonlitedb dump mydb.db --output db.jsonl
_
. (Some of these may work but could have unexpected outcomes.)None
vs. not having the key. However, you can use query_by_path_exists()
to query items that have a certain path. There is no way still to mix this with other queries testing existence other than with None
.Yes and no. The idea is the complete lack of schema needed and as a notable improvement to a JSON file. Plus, if you index the field of interest, you get super-fast queries all the same!
FAQs
SQLite3-backed JSON document database with support for indices and advanced queries
We found that jsonlitedb demonstrated a healthy version release cadence and project activity because the last version was released less than a year ago. It has 1 open source maintainer collaborating on the project.
Did you know?
Socket for GitHub automatically highlights issues in each pull request and monitors the health of all your open source dependencies. Discover the contents of your packages and block harmful activity before you install or update your dependencies.
Security News
Maven Central now validates Sigstore signatures, making it easier for developers to verify the provenance of Java packages.
Security News
CISOs are racing to adopt AI for cybersecurity, but hurdles in budgets and governance may leave some falling behind in the fight against cyber threats.
Research
Security News
Socket researchers uncovered a backdoored typosquat of BoltDB in the Go ecosystem, exploiting Go Module Proxy caching to persist undetected for years.