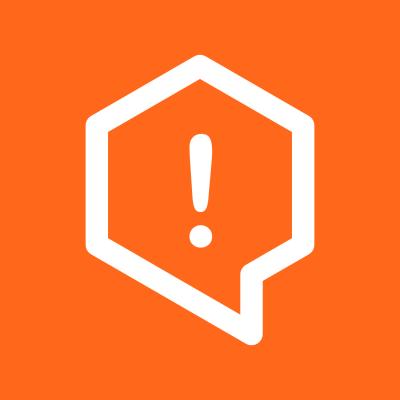
Security News
Fluent Assertions Faces Backlash After Abandoning Open Source Licensing
Fluent Assertions is facing backlash after dropping the Apache license for a commercial model, leaving users blindsided and questioning contributor rights.
llama-index-packs-raptor
Advanced tools
This LlamaPack shows how to use an implementation of RAPTOR with llama-index, leveraging the RAPTOR pack.
RAPTOR works by recursively clustering and summarizing clusters in layers for retrieval.
There two retrieval modes:
See the paper for full algorithm details.
You can download llamapacks directly using llamaindex-cli
, which comes installed with the llama-index
python package:
llamaindex-cli download-llamapack RaptorPack --download-dir ./raptor_pack
You can then inspect/modify the files at ./raptor_pack
and use them as a template for your own project.
You can alternaitvely install the package:
pip install llama-index-packs-raptor
Then, you can import and initialize the pack! This will perform clustering and summarization over your data.
from llama_index.packs.raptor import RaptorPack
pack = RaptorPack(documents, llm=llm, embed_model=embed_model)
The run()
function is a light wrapper around retriever.retrieve()
.
nodes = pack.run(
"query",
mode="collapsed", # or tree_traversal
)
You can also use modules individually.
# get the retriever
retriever = pack.retriever
The RaptorPack
comes with the RaptorRetriever
, which offers ways of saving/reloading!
If you are using a remote vector-db, just pass it in
# Pack usage
pack = RaptorPack(..., vector_store=vector_store)
# RaptorRetriever usage
retriever = RaptorRetriever(..., vector_store=vector_store)
Then, to re-connect, just pass in the vector store again and an empty list of documents
# Pack usage
pack = RaptorPack([], ..., vector_store=vector_store)
# RaptorRetriever usage
retriever = RaptorRetriever([], ..., vector_store=vector_store)
Check out the notebook here for complete details!.
Using the SummaryModule you can configure how the Raptor Pack does summaries and how many workers are applied to summaries.
You can configure the LLM.
You can configure summary_prompt. This will change the prompt sent to your LLM to summarize you docs.
You can configure num_workers, which will influence the number of workers or rather async semaphores allowing more summaries to process simulatneously. This might affect openai or other LLm provider API limits, be aware.
from llama_index.packs.raptor.base import SummaryModule
from llama_index.packs.raptor import RaptorRetriever
summary_prompt = "As a professional summarizer, create a concise and comprehensive summary of the provided text, be it an article, post, conversation, or passage with as much detail as possible."
# Adding SummaryModule you can configure the summary prompt and number of workers doing summaries.
summary_module = SummaryModule(
llm=llm, summary_prompt=summary_prompt, num_workers=16
)
pack = RaptorPack(
documents, llm=llm, embed_model=embed_model, summary_module=summary_module
)
FAQs
llama-index packs raptor integration
We found that llama-index-packs-raptor demonstrated a healthy version release cadence and project activity because the last version was released less than a year ago. It has 1 open source maintainer collaborating on the project.
Did you know?
Socket for GitHub automatically highlights issues in each pull request and monitors the health of all your open source dependencies. Discover the contents of your packages and block harmful activity before you install or update your dependencies.
Security News
Fluent Assertions is facing backlash after dropping the Apache license for a commercial model, leaving users blindsided and questioning contributor rights.
Research
Security News
Socket researchers uncover the risks of a malicious Python package targeting Discord developers.
Security News
The UK is proposing a bold ban on ransomware payments by public entities to disrupt cybercrime, protect critical services, and lead global cybersecurity efforts.