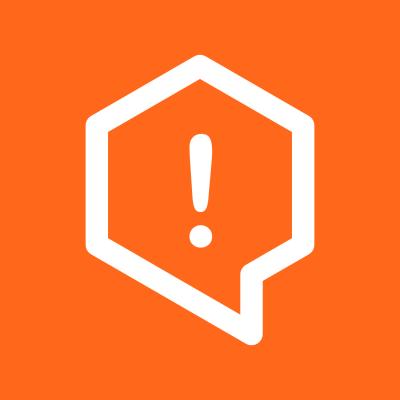
Security News
Fluent Assertions Faces Backlash After Abandoning Open Source Licensing
Fluent Assertions is facing backlash after dropping the Apache license for a commercial model, leaving users blindsided and questioning contributor rights.
This repository contains code for the LLMeBench framework (described in this paper). The framework currently supports evaluation of a variety of NLP tasks using three model providers: OpenAI (e.g., GPT), HuggingFace Inference API, and Petals (e.g., BLOOMZ); it can be seamlessly customized for any NLP task, LLM model and dataset, regardless of language.
Developing LLMeBench is an ongoing effort and it will be continuously expanded. Currently, the framework features the following:
Install LLMeBench: pip install 'llmebench[fewshot]'
Download the current assets: python -m llmebench assets download
. This will fetch assets and place them in the current working directory.
Download one of the dataset, e.g. ArSAS. python -m llmebench data download ArSAS
. This will download the data to the current working directory inside the data
folder.
Evaluate!
For example, to evaluate the performance of a random baseline for Sentiment analysis on ArSAS dataset, you can run:
python -m llmebench --filter 'sentiment/ArSAS_Random*' assets/ results/
which uses the ArSAS_random "asset": a file that specifies the dataset, model and task to evaluate. Here, ArSAS_Random
is the asset name referring to the ArSAS
dataset name and the Random
model, and assets/ar/sentiment_emotion_others/sentiment/
is the directory where the benchmarking asset for the sentiment analysis task on Arabic ArSAS dataset can be found. Results will be saved in a directory called results
.
In addition to supporting the user to implement their own LLM evaluation and benchmarking experiments, the framework comes equipped with benchmarking assets over a large variety of datasets and NLP tasks. To benchmark models on the same datasets, the framework automatically downloads the datasets when possible. Manually downloading them (for example to explore the data before running any assets) can be done as follows:
python -m llmebench data download <DatasetName>
Voilà! all ready to start evaluation...
Note: Some datasets and associated assets are implemented in LLMeBench but the dataset files can't be re-distributed, it is the responsibility of the framework user to acquire them from their original sources. The metadata for each Dataset
includes a link to the primary page for the dataset, which can be used to obtain the data. The data should be downloaded and present in a folder under data/<DatasetName>
, where <DatasetName>
is the same as implementation under llmebench.datasets
. For instance, the ADIDataset
should have it's data under data/ADI/
.
Disclaimer: The datasets associated with the current version of LLMeBench are either existing datasets or processed versions of them. We refer users to the original license accompanying each dataset as provided in the metadata for each dataset script. It is our understanding that these licenses allow for datasets use and redistribution for research or non-commercial purposes .
To run the benchmark,
python -m llmebench --filter '*benchmarking_asset*' --limit <k> --n_shots <n> --ignore_cache <benchmark-dir> <results-dir>
--filter '*benchmarking_asset*'
: (Optional) This flag indicates specific tasks in the benchmark to run. The framework will run a wildcard search using 'benchmarking_asset' in the assets directory specified by <benchmark-dir>
. If not set, the framework will run the entire benchmark.--limit <k>
: (Optional) Specify the number of samples from input data to run through the pipeline, to allow efficient testing. If not set, all the samples in a dataset will be evaluated.--n_shots <n>
: (Optional) If defined, the framework will expect a few-shot asset and will run the few-shot learning paradigm, with n
as the number of shots. If not set, zero-shot will be assumed.--ignore_cache
: (Optional) A flag to ignore loading and saving intermediate model responses from/to cache.<benchmark-dir>
: Path of the directory where the benchmarking assets can be found.<results-dir>
: Path of the directory where to save output results, along with intermediate cached values.AZURE_API_URL
and AZURE_API_KEY
) depending on the benchmark you are running. This can be done by either:
export AZURE_API_KEY="..."
before running the above command, orAZURE_API_URL="..." AZURE_API_KEY="..."
to the above command.--env
flag. Sample dotenv files are provided in the env/
folder<results-dir>
: This folder will contain the outputs resulting from running assets. It follows this structure:
<results-dir>
was specified as the output directory.jq is a helpful command line utility to analyze the resulting json files. The simplest usage is jq . summary.jsonl
, which will print a summary of all samples and model responses in a readable form.
The framework provides caching (if --ignore_cache
isn't passed), to enable the following:
The framework has some preliminary support to automatically select n
examples per test sample based on a maximal marginal relevance-based approach (using langchain's implementation). This will be expanded in the future to have more few shot example selection mechanisms (e.g Random, Class based etc.).
To run few shot assets, supply the --n_shots <n>
option to the benchmarking script. This is set to 0 by default and will run only zero shot assets. If --n_shots
is > zero, only few shot assets are run.
The tutorials directory provides tutorials on the following: updating an existing asset, advanced usage commands to run different benchmarking use cases, and extending the framework by at least one of these components:
Please cite our papers when referring to this framework:
@inproceedings{abdelali-2024-larabench,
title = "{{LAraBench}: Benchmarking Arabic AI with Large Language Models}",
author ={Ahmed Abdelali and Hamdy Mubarak and Shammur Absar Chowdhury and Maram Hasanain and Basel Mousi and Sabri Boughorbel and Samir Abdaljalil and Yassine El Kheir and Daniel Izham and Fahim Dalvi and Majd Hawasly and Nizi Nazar and Yousseif Elshahawy and Ahmed Ali and Nadir Durrani and Natasa Milic-Frayling and Firoj Alam},
booktitle = {Proceedings of the 18th Conference of the European Chapter of the Association for Computational Linguistics: Volume 1, Long Papers},
month = mar,
year = {2024},
address = {Malta},
publisher = {Association for Computational Linguistics},
}
@article{dalvi2023llmebench,
title={{LLMeBench}: A Flexible Framework for Accelerating LLMs Benchmarking},
author={Fahim Dalvi and Maram Hasanain and Sabri Boughorbel and Basel Mousi and Samir Abdaljalil and Nizi Nazar and Ahmed Abdelali and Shammur Absar Chowdhury and Hamdy Mubarak and Ahmed Ali and Majd Hawasly and Nadir Durrani and Firoj Alam},
booktitle = {Proceedings of the 18th Conference of the European Chapter of the Association for Computational Linguistics: System Demonstrations},
month = mar,
year = {2024},
address = {Malta},
publisher = {Association for Computational Linguistics},
}
FAQs
A Flexible Framework for Accelerating LLMs Benchmarking
We found that llmebench demonstrated a healthy version release cadence and project activity because the last version was released less than a year ago. It has 1 open source maintainer collaborating on the project.
Did you know?
Socket for GitHub automatically highlights issues in each pull request and monitors the health of all your open source dependencies. Discover the contents of your packages and block harmful activity before you install or update your dependencies.
Security News
Fluent Assertions is facing backlash after dropping the Apache license for a commercial model, leaving users blindsided and questioning contributor rights.
Research
Security News
Socket researchers uncover the risks of a malicious Python package targeting Discord developers.
Security News
The UK is proposing a bold ban on ransomware payments by public entities to disrupt cybercrime, protect critical services, and lead global cybersecurity efforts.