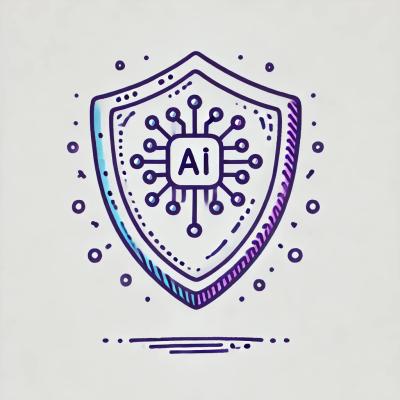
Security News
38% of CISOs Fear They’re Not Moving Fast Enough on AI
CISOs are racing to adopt AI for cybersecurity, but hurdles in budgets and governance may leave some falling behind in the fight against cyber threats.
A python machine learning library to use and visualize gradient descent for linear regression and logistic regression optimization.
Mlektic is a Python library built on top of TensorFlow, designed to simplify the implementation and experimentation with univariate/multivariate linear and logistic regression models. By providing a variety of gradient descent algorithms and regularization techniques, Mlektic enables both beginners and experienced practitioners to efficiently build, train, and evaluate regression models with minimal code.
You can install Mlektic using pip:
pip install mlektic
To train a model using linear regression with standard gradient descent and L1 regularization:
from mlektic.linear_reg import LinearRegressionArcht
from mlektic import preprocessing
from mlektic import methods
import pandas as pd
import numpy as np
# Generate random data.
np.random.seed(42)
n_samples = 100
feature1 = np.random.rand(n_samples)
feature2 = np.random.rand(n_samples)
target = 3 * feature1 + 5 * feature2 + np.random.randn(n_samples) * 0.5
# Create pandas dataframe from the data.
df = pd.DataFrame({
'feature1': feature1,
'feature2': feature2,
'target': target
})
# Create train and test sets.
train_set, test_set = preprocessing.pd_dataset(df, ['feature1', 'feature2'], 'target', 0.8)
# Define regulizer and optimizer.
regularizer = methods.regularizer_archt('l1', lambda_value=0.01)
optimizer = methods.optimizer_archt('sgd-standard')
# Configure the model.
lin_reg = LinearRegressionArcht(iterations=50, optimizer=optimizer, regularizer=regularizer)
# Train the model.
lin_reg.train(train_set)
Epoch 5, Loss: 15.191523551940918
Epoch 10, Loss: 11.642797470092773
Epoch 15, Loss: 9.021803855895996
Epoch 20, Loss: 7.08500862121582
Epoch 25, Loss: 5.652813911437988
Epoch 30, Loss: 4.592779636383057
Epoch 35, Loss: 3.807236909866333
Epoch 40, Loss: 3.2241621017456055
Epoch 45, Loss: 2.790440320968628
Epoch 50, Loss: 2.4669017791748047
The cost evolution can be plotted with:
from mlektic.plot_utils import plot_cost
cost_history = lin_reg.get_cost_history()
plot_cost(cost_history, dim = (7, 5))
You can replace LinearRegressionArcht
with LogisticRegressionArcht
, and try different types of optimizers and regularizers.
For more detailed information, including API references and advanced usage, please refer to the full documentation.
Contributions are welcome! If you have suggestions for improvements, feel free to open an issue or send me an email to contacto@dialektico.com.
Mlektic is licensed under the Apache 2.0 License. See the LICENSE file for more details.
FAQs
A python machine learning library to use and visualize gradient descent for linear regression and logistic regression optimization.
We found that mlektic demonstrated a healthy version release cadence and project activity because the last version was released less than a year ago. It has 1 open source maintainer collaborating on the project.
Did you know?
Socket for GitHub automatically highlights issues in each pull request and monitors the health of all your open source dependencies. Discover the contents of your packages and block harmful activity before you install or update your dependencies.
Security News
CISOs are racing to adopt AI for cybersecurity, but hurdles in budgets and governance may leave some falling behind in the fight against cyber threats.
Research
Security News
Socket researchers uncovered a backdoored typosquat of BoltDB in the Go ecosystem, exploiting Go Module Proxy caching to persist undetected for years.
Security News
Company News
Socket is joining TC54 to help develop standards for software supply chain security, contributing to the evolution of SBOMs, CycloneDX, and Package URL specifications.