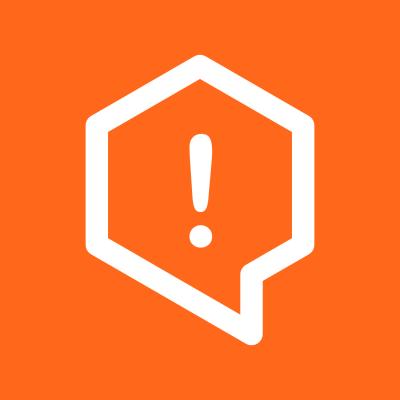
Security News
Fluent Assertions Faces Backlash After Abandoning Open Source Licensing
Fluent Assertions is facing backlash after dropping the Apache license for a commercial model, leaving users blindsided and questioning contributor rights.
The nanoarrow Python package provides bindings to the nanoarrow C library. Like the nanoarrow C library, it provides tools to facilitate the use of the Arrow C Data and Arrow C Stream interfaces.
The nanoarrow Python bindings are available from PyPI and conda-forge:
pip install nanoarrow
conda install nanoarrow -c conda-forge
Development versions (based on the main
branch) are also available:
pip install --extra-index-url https://pypi.fury.io/arrow-nightlies/ \
--prefer-binary --pre nanoarrow
If you can import the namespace, you're good to go!
import nanoarrow as na
The Arrow C Data and Arrow C Stream interfaces are comprised of three structures: the ArrowSchema
which represents a data type of an array, the ArrowArray
which represents the values of an array, and an ArrowArrayStream
, which represents zero or more ArrowArray
s with a common ArrowSchema
. These concepts map to the nanoarrow.Schema
, nanoarrow.Array
, and nanoarrow.ArrayStream
in the Python package.
na.int32()
<Schema> int32
na.Array([1, 2, 3], na.int32())
nanoarrow.Array<int32>[3]
1
2
3
The nanoarrow.Array
can accommodate arrays with any number of chunks, reflecting the reality that many array containers (e.g., pyarrow.ChunkedArray
, polars.Series
) support this.
chunked = na.Array.from_chunks([[1, 2, 3], [4, 5, 6]], na.int32())
chunked
nanoarrow.Array<int32>[6]
1
2
3
4
5
6
Whereas chunks of an Array
are always fully materialized when the object is constructed, the chunks of an ArrayStream
have not necessarily been resolved yet.
stream = na.ArrayStream(chunked)
stream
nanoarrow.ArrayStream<int32>
with stream:
for chunk in stream:
print(chunk)
nanoarrow.Array<int32>[3]
1
2
3
nanoarrow.Array<int32>[3]
4
5
6
The nanoarrow.ArrayStream
also provides an interface to nanoarrow's Arrow IPC reader:
url = "https://github.com/apache/arrow-experiments/raw/main/data/arrow-commits/arrow-commits.arrows"
na.ArrayStream.from_url(url)
nanoarrow.ArrayStream<non-nullable struct<commit: string, time: timestamp('us', 'UTC'), files: int3...>
These objects implement the Arrow PyCapsule interface for both producing and consuming and are interchangeable with pyarrow
objects in many cases:
import pyarrow as pa
pa.field(na.int32())
pyarrow.Field<: int32>
pa.chunked_array(chunked)
<pyarrow.lib.ChunkedArray object at 0x12a49a250>
[
[
1,
2,
3
],
[
4,
5,
6
]
]
pa.array(chunked.chunk(1))
<pyarrow.lib.Int32Array object at 0x11b552500>
[
4,
5,
6
]
na.Array(pa.array([10, 11, 12]))
nanoarrow.Array<int64>[3]
10
11
12
na.Schema(pa.string())
<Schema> string
The nanoarrow Python package also provides lower level wrappers around Arrow C interface structures. You can create these using nanoarrow.c_schema()
, nanoarrow.c_array()
, and nanoarrow.c_array_stream()
.
Use nanoarrow.c_schema()
to convert an object to an ArrowSchema
and wrap it as a Python object. This works for any object implementing the Arrow PyCapsule Interface (e.g., pyarrow.Schema
, pyarrow.DataType
, and pyarrow.Field
).
na.c_schema(pa.decimal128(10, 3))
<nanoarrow.c_schema.CSchema decimal128(10, 3)>
- format: 'd:10,3'
- name: ''
- flags: 2
- metadata: NULL
- dictionary: NULL
- children[0]:
Using c_schema()
is a good fit for testing and for ephemeral schema objects that are being passed from one library to another. To extract the fields of a schema in a more convenient form, use Schema()
:
schema = na.Schema(pa.decimal128(10, 3))
schema.precision, schema.scale
(10, 3)
The CSchema
object cleans up after itself: when the object is deleted, the underlying ArrowSchema
is released.
You can use nanoarrow.c_array()
to convert an array-like object to an ArrowArray
, wrap it as a Python object, and attach a schema that can be used to interpret its contents. This works for any object implementing the Arrow PyCapsule Interface (e.g., pyarrow.Array
, pyarrow.RecordBatch
).
na.c_array(["one", "two", "three", None], na.string())
<nanoarrow.c_array.CArray string>
- length: 4
- offset: 0
- null_count: 1
- buffers: (4754305168, 4754307808, 4754310464)
- dictionary: NULL
- children[0]:
Using c_array()
is a good fit for testing and for ephemeral array objects that are being passed from one library to another. For a higher level interface, use Array()
:
array = na.Array(["one", "two", "three", None], na.string())
array.to_pylist()
['one', 'two', 'three', None]
array.buffers
(nanoarrow.c_lib.CBufferView(bool[1 b] 11100000),
nanoarrow.c_lib.CBufferView(int32[20 b] 0 3 6 11 11),
nanoarrow.c_lib.CBufferView(string[11 b] b'onetwothree'))
Advanced users can create arrays directly from buffers using c_array_from_buffers()
:
na.c_array_from_buffers(
na.string(),
2,
[None, na.c_buffer([0, 3, 6], na.int32()), b"abcdef"]
)
<nanoarrow.c_array.CArray string>
- length: 2
- offset: 0
- null_count: 0
- buffers: (0, 5002908320, 4999694624)
- dictionary: NULL
- children[0]:
You can use nanoarrow.c_array_stream()
to wrap an object representing a sequence of CArray
s with a common CSchema
to an ArrowArrayStream
and wrap it as a Python object. This works for any object implementing the Arrow PyCapsule Interface (e.g., pyarrow.RecordBatchReader
, pyarrow.ChunkedArray
).
pa_batch = pa.record_batch({"col1": [1, 2, 3]})
reader = pa.RecordBatchReader.from_batches(pa_batch.schema, [pa_batch])
array_stream = na.c_array_stream(reader)
array_stream
<nanoarrow.c_array_stream.CArrayStream>
- get_schema(): struct<col1: int64>
You can pull the next array from the stream using .get_next()
or use it like an iterator. The .get_next()
method will raise StopIteration
when there are no more arrays in the stream.
for array in array_stream:
print(array)
<nanoarrow.c_array.CArray struct<col1: int64>>
- length: 3
- offset: 0
- null_count: 0
- buffers: (0,)
- dictionary: NULL
- children[1]:
'col1': <nanoarrow.c_array.CArray int64>
- length: 3
- offset: 0
- null_count: 0
- buffers: (0, 2642948588352)
- dictionary: NULL
- children[0]:
Use ArrayStream()
for a higher level interface:
reader = pa.RecordBatchReader.from_batches(pa_batch.schema, [pa_batch])
na.ArrayStream(reader).read_all()
nanoarrow.Array<non-nullable struct<col1: int64>>[3]
{'col1': 1}
{'col1': 2}
{'col1': 3}
Python bindings for nanoarrow are managed with setuptools. This means you can build the project using:
git clone https://github.com/apache/arrow-nanoarrow.git
cd arrow-nanoarrow/python
pip install -e .
Tests use pytest:
# Install dependencies
pip install -e ".[test]"
# Run tests
pytest -vvx
CMake is currently required to ensure that the vendored copy of nanoarrow in the Python package stays in sync with the nanoarrow sources in the working tree.
FAQs
Python bindings to the nanoarrow C library
We found that nanoarrow demonstrated a healthy version release cadence and project activity because the last version was released less than a year ago. It has 1 open source maintainer collaborating on the project.
Did you know?
Socket for GitHub automatically highlights issues in each pull request and monitors the health of all your open source dependencies. Discover the contents of your packages and block harmful activity before you install or update your dependencies.
Security News
Fluent Assertions is facing backlash after dropping the Apache license for a commercial model, leaving users blindsided and questioning contributor rights.
Research
Security News
Socket researchers uncover the risks of a malicious Python package targeting Discord developers.
Security News
The UK is proposing a bold ban on ransomware payments by public entities to disrupt cybercrime, protect critical services, and lead global cybersecurity efforts.