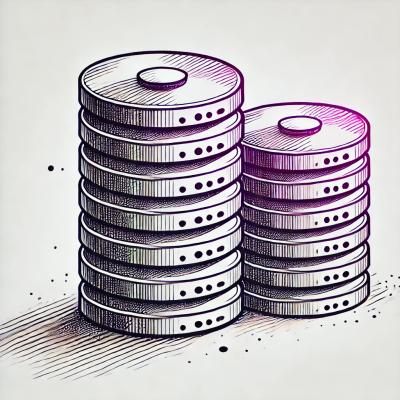
Security News
MCP Community Begins Work on Official MCP Metaregistry
The MCP community is launching an official registry to standardize AI tool discovery and let agents dynamically find and install MCP servers.
NVIDIA NeMo Framework is a scalable and cloud-native generative AI framework built for researchers and PyTorch developers working on Large Language Models (LLMs), Multimodal Models (MMs), Automatic Speech Recognition (ASR), Text to Speech (TTS), and Computer Vision (CV) domains. It is designed to help you efficiently create, customize, and deploy new generative AI models by leveraging existing code and pre-trained model checkpoints.
For technical documentation, please see the NeMo Framework User Guide.
NVIDIA NeMo 2.0 introduces several significant improvements over its predecessor, NeMo 1.0, enhancing flexibility, performance, and scalability.
Python-Based Configuration - NeMo 2.0 transitions from YAML files to a Python-based configuration, providing more flexibility and control. This shift makes it easier to extend and customize configurations programmatically.
Modular Abstractions - By adopting PyTorch Lightning’s modular abstractions, NeMo 2.0 simplifies adaptation and experimentation. This modular approach allows developers to more easily modify and experiment with different components of their models.
Scalability - NeMo 2.0 seamlessly scaling large-scale experiments across thousands of GPUs using NeMo-Run, a powerful tool designed to streamline the configuration, execution, and management of machine learning experiments across computing environments.
Overall, these enhancements make NeMo 2.0 a powerful, scalable, and user-friendly framework for AI model development.
[!IMPORTANT]
NeMo 2.0 is currently supported by the LLM (large language model) and VLM (vision language model) collections.
NeMo Curator and NeMo Framework support video curation and post-training of the Cosmos World Foundation Models, which are open and available on NGC and Hugging Face. For more information on video datasets, refer to NeMo Curator. To post-train World Foundation Models using the NeMo Framework for your custom physical AI tasks, see the Cosmos Diffusion models and the Cosmos Autoregressive models.
All NeMo models are trained with Lightning. Training is automatically scalable to 1000s of GPUs. You can check the performance benchmarks using the latest NeMo Framework container here.
When applicable, NeMo models leverage cutting-edge distributed training techniques, incorporating parallelism strategies to enable efficient training of very large models. These techniques include Tensor Parallelism (TP), Pipeline Parallelism (PP), Fully Sharded Data Parallelism (FSDP), Mixture-of-Experts (MoE), and Mixed Precision Training with BFloat16 and FP8, as well as others.
NeMo Transformer-based LLMs and MMs utilize NVIDIA Transformer Engine for FP8 training on NVIDIA Hopper GPUs, while leveraging NVIDIA Megatron Core for scaling Transformer model training.
NeMo LLMs can be aligned with state-of-the-art methods such as SteerLM, Direct Preference Optimization (DPO), and Reinforcement Learning from Human Feedback (RLHF). See NVIDIA NeMo Aligner for more information.
In addition to supervised fine-tuning (SFT), NeMo also supports the latest parameter efficient fine-tuning (PEFT) techniques such as LoRA, P-Tuning, Adapters, and IA3. Refer to the NeMo Framework User Guide for the full list of supported models and techniques.
NeMo LLMs and MMs can be deployed and optimized with NVIDIA NeMo Microservices.
NeMo ASR and TTS models can be optimized for inference and deployed for production use cases with NVIDIA Riva.
[!IMPORTANT]
NeMo Framework Launcher is compatible with NeMo version 1.0 only. NeMo-Run is recommended for launching experiments using NeMo 2.0.
NeMo Framework Launcher is a cloud-native tool that streamlines the NeMo Framework experience. It is used for launching end-to-end NeMo Framework training jobs on CSPs and Slurm clusters.
The NeMo Framework Launcher includes extensive recipes, scripts, utilities, and documentation for training NeMo LLMs. It also includes the NeMo Framework Autoconfigurator, which is designed to find the optimal model parallel configuration for training on a specific cluster.
To get started quickly with the NeMo Framework Launcher, please see the NeMo Framework Playbooks. The NeMo Framework Launcher does not currently support ASR and TTS training, but it will soon.
Getting started with NeMo Framework is easy. State-of-the-art pretrained NeMo models are freely available on Hugging Face Hub and NVIDIA NGC. These models can be used to generate text or images, transcribe audio, and synthesize speech in just a few lines of code.
We have extensive tutorials that can be run on Google Colab or with our NGC NeMo Framework Container. We also have playbooks for users who want to train NeMo models with the NeMo Framework Launcher.
For advanced users who want to train NeMo models from scratch or fine-tune existing NeMo models, we have a full suite of example scripts that support multi-GPU/multi-node training.
Version | Status | Description |
---|---|---|
Latest | Documentation of the latest (i.e. main) branch. | |
Stable | Documentation of the stable (i.e. most recent release) |
The NeMo Framework can be installed in a variety of ways, depending on your needs. Depending on the domain, you may find one of the following installation methods more suitable.
NeMo-Framework provides tiers of support based on OS / Platform and mode of installation. Please refer the following overview of support levels:
Please refer to the following table for current support levels:
OS / Platform | Install from PyPi | Source into NGC container |
---|---|---|
linux - amd64/x84_64 | Limited support | Full support |
linux - arm64 | Limited support | Limited support |
darwin - amd64/x64_64 | Deprecated | Deprecated |
darwin - arm64 | Limited support | Limited support |
windows - amd64/x64_64 | No support yet | No support yet |
windows - arm64 | No support yet | No support yet |
Install NeMo in a fresh Conda environment:
conda create --name nemo python==3.10.12
conda activate nemo
NeMo-Framework publishes pre-built wheels with each release. To install nemo_toolkit from such a wheel, use the following installation method:
pip install "nemo_toolkit[all]"
If a more specific version is desired, we recommend a Pip-VCS install. From NVIDIA/NeMo, fetch the commit, branch, or tag that you would like to install.
To install nemo_toolkit from this Git reference $REF
, use the following installation method:
git clone https://github.com/NVIDIA/NeMo
cd NeMo
git checkout @${REF:-'main'}
pip install '.[all]'
To install a specific domain of NeMo, you must first install the nemo_toolkit using the instructions listed above. Then, you run the following domain-specific commands:
pip install nemo_toolkit['all'] # or pip install "nemo_toolkit['all']@git+https://github.com/NVIDIA/NeMo@${REF:-'main'}"
pip install nemo_toolkit['asr'] # or pip install "nemo_toolkit['asr']@git+https://github.com/NVIDIA/NeMo@$REF:-'main'}"
pip install nemo_toolkit['nlp'] # or pip install "nemo_toolkit['nlp']@git+https://github.com/NVIDIA/NeMo@${REF:-'main'}"
pip install nemo_toolkit['tts'] # or pip install "nemo_toolkit['tts']@git+https://github.com/NVIDIA/NeMo@${REF:-'main'}"
pip install nemo_toolkit['vision'] # or pip install "nemo_toolkit['vision']@git+https://github.com/NVIDIA/NeMo@${REF:-'main'}"
pip install nemo_toolkit['multimodal'] # or pip install "nemo_toolkit['multimodal']@git+https://github.com/NVIDIA/NeMo@${REF:-'main'}"
NOTE: The following steps are supported beginning with 24.04 (NeMo-Toolkit 2.3.0)
We recommended that you start with a base NVIDIA PyTorch container: nvcr.io/nvidia/pytorch:25.01-py3.
If starting with a base NVIDIA PyTorch container, you must first launch the container:
docker run \
--gpus all \
-it \
--rm \
--shm-size=16g \
--ulimit memlock=-1 \
--ulimit stack=67108864 \
nvcr.io/nvidia/pytorch:${NV_PYTORCH_TAG:-'nvcr.io/nvidia/pytorch:25.01-py3'}
From NVIDIA/NeMo, fetch the commit/branch/tag that you want to install.
To install nemo_toolkit including all of its dependencies from this Git reference $REF
, use the following installation method:
cd /opt
git clone https://github.com/NVIDIA/NeMo
cd NeMo
git checkout ${REF:-'main'}
bash reinstall.sh --library all
NeMo containers are launched concurrently with NeMo version updates. NeMo Framework now supports LLMs, MMs, ASR, and TTS in a single consolidated Docker container. You can find additional information about released containers on the NeMo releases page.
To use a pre-built container, run the following code:
docker run \
--gpus all \
-it \
--rm \
--shm-size=16g \
--ulimit memlock=-1 \
--ulimit stack=67108864 \
nvcr.io/nvidia/pytorch:${NV_PYTORCH_TAG:-'nvcr.io/nvidia/nemo:25.02'}
The NeMo Framework Launcher does not currently support ASR and TTS training, but it will soon.
FAQ can be found on the NeMo Discussions board. You are welcome to ask questions or start discussions on the board.
We welcome community contributions! Please refer to CONTRIBUTING.md for the process.
We provide an ever-growing list of publications that utilize the NeMo Framework.
To contribute an article to the collection, please submit a pull request
to the gh-pages-src
branch of this repository. For detailed
information, please consult the README located at the gh-pages-src
branch.
FAQs
NeMo - a toolkit for Conversational AI
We found that nemo-toolkit demonstrated a healthy version release cadence and project activity because the last version was released less than a year ago. It has 3 open source maintainers collaborating on the project.
Did you know?
Socket for GitHub automatically highlights issues in each pull request and monitors the health of all your open source dependencies. Discover the contents of your packages and block harmful activity before you install or update your dependencies.
Security News
The MCP community is launching an official registry to standardize AI tool discovery and let agents dynamically find and install MCP servers.
Research
Security News
Socket uncovers an npm Trojan stealing crypto wallets and BullX credentials via obfuscated code and Telegram exfiltration.
Research
Security News
Malicious npm packages posing as developer tools target macOS Cursor IDE users, stealing credentials and modifying files to gain persistent backdoor access.