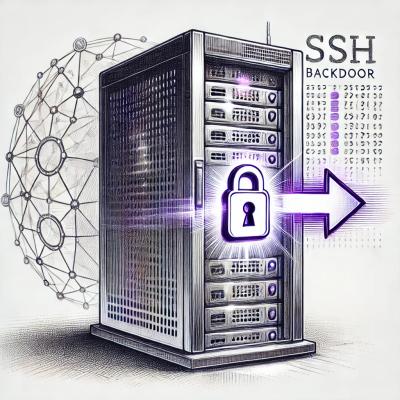
Research
Security News
Malicious npm Packages Inject SSH Backdoors via Typosquatted Libraries
Socket’s threat research team has detected six malicious npm packages typosquatting popular libraries to insert SSH backdoors.
Python package for parsing configurations from YAML and TOML and command-line interface.
Python package for parsing configurations from YAML and TOML files with the command-line interface.
You can install omniconfig using pip:
pip install omniconfig
git clone https://github.com/synxlin/omniconfig
cd omniconfig
conda env create -f environment.yml
poetry install
Decorator configclass
in omniconfig
package can help build argument parser from dataclass
in dataclasses
. Here is an example:
from dataclasses import dataclass, field
from typing import Any, Callable
import omniconfig
@omniconfig.configclass
@dataclass
class EvalConfig:
"""Evaluation Config class.
Attributes:
num_gpus (int): the number of GPUs. Defaults to ``8``.
"""
num_gpus: int = 8
@omniconfig.configclass
@dataclass
class QuantConfig:
"""Quantization config class.
Attributes:
dtype (str): Quantization data type. Defaults to ``"torch.float16"``.
group_shape (list[int]): Quantization group shape. Defaults to ``(1, -1)``.
"""
dtype: str = "torch.float16"
group_shape: list[int] = (1, -1)
@classmethod
def update_get_arguments(
cls: type["QuantConfig"],
*,
overwrites: dict[str, Callable | None] | None = None,
defaults: dict[str, Any] | None = None,
) -> tuple[dict[str, Callable | None], dict[str, Any]]:
"""Get the arguments for the quantization configuration."""
overwrites = overwrites or {}
defaults = defaults or {}
overwrites.setdefault(
"group_shape",
lambda parser: parser.add_argument(
"--group-shape",
nargs="+",
type=int,
default=defaults.get("group_shape", (1, -1)),
help="Quantization group shape",
),
)
return overwrites, defaults
@omniconfig.configclass
@dataclass
class ModelConfig:
"""Model Config class.
Attributes:
name (str): the model name.
group (str): the model family.
"""
name: str
family: str = field(init=False, default="")
def __post_init__(self):
self.family = self.name.split("-")[0]
@omniconfig.configclass
@dataclass
class QuantizedModelConfig(ModelConfig):
"""Quantized Model Config class.
Attributes:
name (str): the model name.
group (str): the model group.
quant (QuantConfig): quantization configuration. Defaults to ``None``.
"""
quant: QuantConfig | None = None
@omniconfig.configclass
@dataclass
class Config:
"""Config class.
Attributes:
model (QuantizedModelConfig): the quantized model config.
eval (EvalConfig): the evaluation config.
"""
model: QuantizedModelConfig
eval: EvalConfig
@staticmethod
def parse_args(args: Any = None) -> tuple["Config", dict[str, dict], list[str]]:
"""Parse arguments.
Args:
args (list[str], optional): Arguments to parse. Defaults to ``None``.
Returns:
tuple[Config, dict[str, dict], list[str]]: Configs from the parsed arguments,
parsed yaml configs, and unknown arguments.
"""
parser = omniconfig.ConfigParser("Evaluate Quantized Model")
parser.add_config(Config)
config, parsed_args, unknown_args = parser.parse_known_args(args)
assert isinstance(config, Config)
return config, parsed_args, unknown_args
@staticmethod
def dump_default(path: str = "default.yaml") -> None:
"""Dump default configuration to a yaml file.
Args:
path (str, optional): The path to save the default configuration. Defaults to ``"default.yaml"``.
"""
parser = omniconfig.ConfigParser("Evaluate Quantized Model")
parser.add_config(Config)
parser.dump_default(path)
def main(args: Any = None) -> None: # noqa: C901
"""Evaluate Quantized Model with the given arguments.
Args:
args (list[str], optional): Arguments to parse. Defaults to ``None``.
"""
config, parsed_args, unknown_args = Config.parse_args(args)
...
In the example above, configclass
will automatically generate the following flags for command-line argument parser:
usage: Evaluate Quantized Model [-h] [--model-name MODEL_NAME] [--model-enable-quant] [--model-quant-dtype MODEL_QUANT_DTYPE] [--model-quant-group-shape MODEL_QUANT_GROUP_SHAPE [MODEL_QUANT_GROUP_SHAPE ...]]
[--eval-num-gpus EVAL_NUM_GPUS]
[cfgs ...]
positional arguments:
cfgs config file(s)
options:
-h, --help show this help message and exit
--model-name MODEL_NAME
the model name for model.
--model-enable-quant Enable quant for model. Default: False.
--model-quant-dtype MODEL_QUANT_DTYPE
Quantization data type for model_quant. Default: torch.float16.
--model-quant-group-shape MODEL_QUANT_GROUP_SHAPE [MODEL_QUANT_GROUP_SHAPE ...]
Quantization group shape for model_quant. Default: (1, -1).
--eval-num-gpus EVAL_NUM_GPUS
the number of GPUs for eval. Default: 8.
Note that
configclass
will automatically extract help message from the docstring of the class.
configclass
will always set the first positional argument as paths to config files. Current supported config file type is YAML and TOML. An example YAML config should be like:
model:
name: llama2-7b
enable_quant: true
quant:
dtype: sint8
group_shape:
- 1
- -1
eval:
num_gpus: 8
in
QuantConfig
, we overwrite the command-line parser for fieldgroup_shape
by overriding the classmethodupdate_get_arguments
. We can also add any other field inside the classmethodupdate_get_arguments
and override the classmethodupdate_from_dict
to handle these extra fields.
in
ModelConfig
, the fieldfamily
is set withinit=False
, and thusconfigclass
will not generate parser forfamily
field.
class
QuantizedModelConfig
inherited fromModelConfig
, thus it will also inherited all fields fromModelConfig
.
in
QuantizedModelConfig
, the fieldquant
is a type ofOptional[QuantConfig]
, and thus, the parser will automatically add a--model-enable-quant
to help set fieldquant
toNone
.
FAQs
Python package for parsing configurations from YAML and TOML and command-line interface.
We found that omniconfig demonstrated a healthy version release cadence and project activity because the last version was released less than a year ago. It has 1 open source maintainer collaborating on the project.
Did you know?
Socket for GitHub automatically highlights issues in each pull request and monitors the health of all your open source dependencies. Discover the contents of your packages and block harmful activity before you install or update your dependencies.
Research
Security News
Socket’s threat research team has detected six malicious npm packages typosquatting popular libraries to insert SSH backdoors.
Security News
MITRE's 2024 CWE Top 25 highlights critical software vulnerabilities like XSS, SQL Injection, and CSRF, reflecting shifts due to a refined ranking methodology.
Security News
In this segment of the Risky Business podcast, Feross Aboukhadijeh and Patrick Gray discuss the challenges of tracking malware discovered in open source softare.