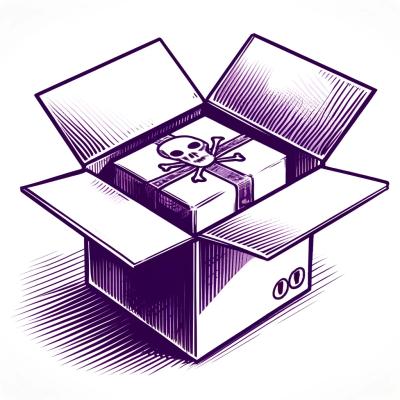
Security News
Research
Data Theft Repackaged: A Case Study in Malicious Wrapper Packages on npm
The Socket Research Team breaks down a malicious wrapper package that uses obfuscation to harvest credentials and exfiltrate sensitive data.
polars-coord-transforms
Advanced tools
Tools for coordinate transforms and S2-indexing via Polars Expression Plugins
This Polars plugin provides functionality which can be loosely described as tranformation of coordinates and extraction of features from them.
It contains functions which were needed in personal and work projects, therefore its set of features might appear a bit random. Nevertheless one can find it useful in projects related to robotics, geospatial science, spatial analytics etc.
The functions are divided among three namespaces: transform
, s2
, distance
:
transform
namespace contains functions for converting coordinates from\to map, ecef, lla, utm reference frames.
s2
namespace contains functions which allow to work with S2 Cells
distance
namespace allows to calculate distances between coordinates.
This plugin presupposes that coordianates represent points in space and that they are expressed with struct
datatype in Polars.
pip install polars-coord-transforms
import polars_coord_transforms
In order to use plugin, coordinates should be represented as struct
with fields x
, y
, z
(or, in case of LLA-points: lon
, lat
, alt
)!
For instance, if coordinates are in separate columns, one can make a valid struct
with pl.struct
native Polars function:
import polars as pl
df = pl.DataFrame(
dict(
lon=[31.409197919000064,],
lat=[58.860667429000046,],
alt=[57.309668855211015,],
)
)
df.with_columns(
point=pl.struct("lon", "lat", "alt")
)
Suppose we have the following DataFrame with some coordinates (column "pose"), rotation quaternion (column "rotation") and offset vector (column "offset"):
import polars as pl
df = pl.DataFrame(
[
pl.Series("pose", [{'x': 4190.66735544079, 'y': 14338.862844330957, 'z': 10.96391354687512}], dtype=pl.Struct({'x': pl.Float64, 'y': pl.Float64, 'z': pl.Float64})),
pl.Series("rotation", [{'x': 0.13007119, 'y': 0.26472049, 'z': 0.85758219, 'w': 0.42137553}], dtype=pl.Struct({'x': pl.Float64, 'y': pl.Float64, 'z': pl.Float64, 'w': pl.Float64})),
pl.Series("offset", [{'x': 2852423.40536658, 'y': 2201848.41975346, 'z': 5245234.74365368}], dtype=pl.Struct({'x': pl.Float64, 'y': pl.Float64, 'z': pl.Float64})),
]
)
print(df)
shape: (1, 3)
┌─────────────────────────────┬───────────────────────────┬───────────────────────────────────┐
│ pose ┆ rotation ┆ offset │
│ --- ┆ --- ┆ --- │
│ struct[3] ┆ struct[4] ┆ struct[3] │
╞═════════════════════════════╪═══════════════════════════╪═══════════════════════════════════╡
│ {4190.667,14338.863,10.964} ┆ {0.130,0.265,0.858,0.421} ┆ {2852423.405,2201848.420,5245234… │
└─────────────────────────────┴───────────────────────────┴───────────────────────────────────┘
transform
df.with_columns(
ecef=pl.col("pose").transform.map_to_ecef(
pl.col("rotation"), pl.col("offset")
)
)
shape: (1, 4)
┌────────────────────────┬────────────────────────┬────────────────────────┬───────────────────────┐
│ pose ┆ rotation ┆ offset ┆ ecef │
│ --- ┆ --- ┆ --- ┆ --- │
│ struct[3] ┆ struct[4] ┆ struct[3] ┆ struct[3] │
╞════════════════════════╪════════════════════════╪════════════════════════╪═══════════════════════╡
│ {4190.667,14338.863,10 ┆ {0.130,0.265,0.858,0.4 ┆ {2852423.405,2201848.4 ┆ {2840491.941,2197932. │
│ .964} ┆ 21} ┆ 20,5245234… ┆ 225,5253325… │
└────────────────────────┴────────────────────────┴────────────────────────┴───────────────────────┘
df.with_columns(
pose_new=pl.col("ecef").transform.ecef_to_map("rotation", "offset")
).select(
"pose",
"pose_new"
)
shape: (1, 5)
┌───────────────────┬───────────────────┬───────────────────┬───────────────────┬──────────────────┐
│ pose ┆ rotation ┆ offset ┆ ecef ┆ pose_new │
│ --- ┆ --- ┆ --- ┆ --- ┆ --- │
│ struct[3] ┆ struct[4] ┆ struct[3] ┆ struct[3] ┆ struct[3] │
╞═══════════════════╪═══════════════════╪═══════════════════╪═══════════════════╪══════════════════╡
│ {4190.667,14338.8 ┆ {0.130,0.265,0.85 ┆ {2852423.405,2201 ┆ {2840491.941,2197 ┆ {4190.667,14338. │
│ 63,10.964} ┆ 8,0.421} ┆ 848.420,5245234… ┆ 932.225,5253325… ┆ 863,10.964} │
└───────────────────┴───────────────────┴───────────────────┴───────────────────┴──────────────────┘
df.with_columns(
lla=pl.col("ecef").transform.ecef_to_lla()
)
shape: (1, 3)
┌─────────────────────────────┬───────────────────────────────────┬─────────────────────────┐
│ pose ┆ ecef ┆ lla │
│ --- ┆ --- ┆ --- │
│ struct[3] ┆ struct[3] ┆ struct[3] │
╞═════════════════════════════╪═══════════════════════════════════╪═════════════════════════╡
│ {4190.667,14338.863,10.964} ┆ {2840491.941,2197932.225,5253325… ┆ {37.732,55.820,163.916} │
└─────────────────────────────┴───────────────────────────────────┴─────────────────────────┘
df.with_columns(
ecef_new=pl.col("lla").transform.lla_to_ecef()
)
shape: (1, 4)
┌────────────────────────┬────────────────────────┬────────────────────────┬───────────────────────┐
│ pose ┆ ecef ┆ lla ┆ ecef_new │
│ --- ┆ --- ┆ --- ┆ --- │
│ struct[3] ┆ struct[3] ┆ struct[3] ┆ struct[3] │
╞════════════════════════╪════════════════════════╪════════════════════════╪═══════════════════════╡
│ {4190.667,14338.863,10 ┆ {2840491.941,2197932.2 ┆ {37.732,55.820,163.916 ┆ {2840491.941,2197932. │
│ .964} ┆ 25,5253325… ┆ } ┆ 225,5253325… │
└────────────────────────┴────────────────────────┴────────────────────────┴───────────────────────┘
df.with_columns(
utm=pl.col("lla").transform.lla_to_utm()
)
shape: (1, 3)
┌─────────────────────────────┬─────────────────────────┬──────────────────────────────────┐
│ pose ┆ lla ┆ utm │
│ --- ┆ --- ┆ --- │
│ struct[3] ┆ struct[3] ┆ struct[3] │
╞═════════════════════════════╪═════════════════════════╪══════════════════════════════════╡
│ {4190.667,14338.863,10.964} ┆ {37.732,55.820,163.916} ┆ {420564.380,6186739.936,163.916} │
└─────────────────────────────┴─────────────────────────┴──────────────────────────────────┘
df.with_columns(
utm_zone_number=pl.col("lla").transform.lla_to_utm_zone_number()
)
shape: (1, 3)
┌─────────────────────────┬──────────────────────────────────┬─────────────────┐
│ lla ┆ utm ┆ utm_zone_number │
│ --- ┆ --- ┆ --- │
│ struct[3] ┆ struct[3] ┆ u8 │
╞═════════════════════════╪══════════════════════════════════╪═════════════════╡
│ {37.732,55.820,163.916} ┆ {420564.380,6186739.936,163.916} ┆ 37 │
└─────────────────────────┴──────────────────────────────────┴─────────────────┘
the function returns a struct with 3 fields:"roll", "pitch", "yaw"
df.select(
euler_angles=pl.col("rotation").transform.quat_to_euler_angles()
)
┌──────────────────────────────┐
│ euler_angles │
│ --- │
│ struct[3] │
╞══════════════════════════════╡
│ {0.598806,0.000000,2.228181} │
└──────────────────────────────┘
s2
df.select(
cellid_30=pl.col("lla").s2.lonlat_to_cellid(level=30),
cellid_28=pl.col("lla").s2.lonlat_to_cellid(level=28),
cellid_5=pl.col("lla").s2.lonlat_to_cellid(level=5),
)
shape: (1, 3)
┌─────────────────────┬─────────────────────┬─────────────────────┐
│ cellid_30 ┆ cellid_28 ┆ cellid_5 │
│ --- ┆ --- ┆ --- │
│ u64 ┆ u64 ┆ u64 │
╞═════════════════════╪═════════════════════╪═════════════════════╡
│ 5095036114269810839 ┆ 5095036114269810832 ┆ 5094697078462873600 │
└─────────────────────┴─────────────────────┴─────────────────────┘
df.select(
lla_cell=pl.lit(5095036114269810839, dtype=pl.UInt64()).s2.cellid_to_lonlat()
)
shape: (1, 1)
┌─────────────────┐
│ lla_cell │
│ --- │
│ struct[2] │
╞═════════════════╡
│ {37.732,55.820} │
└─────────────────┘
df.select(
lla",
cellid=pl.lit(5095036114269810832, dtype=pl.UInt64()),
is_in_cell=pl.lit(5095036114269810832, dtype=pl.UInt64()).s2.cell_contains_point(pl.col("lla"))
)
shape: (1, 3)
┌─────────────────────────┬─────────────────────┬────────────┐
│ lla ┆ cellid ┆ is_in_cell │
│ --- ┆ --- ┆ --- │
│ struct[3] ┆ u64 ┆ bool │
╞═════════════════════════╪═════════════════════╪════════════╡
│ {37.732,55.820,163.916} ┆ 5095036114269810832 ┆ true │
└─────────────────────────┴─────────────────────┴────────────┘
df.with_columns(
cellid=pl.col("lla").s2.lonlat_to_cellid(level=5),
).with_columns(
vertices=pl.col("cellid").s2.cellid_to_vertices()
)
shape: (1, 4)
┌─────────────────────────┬─────────────────────────┬─────────────────────┬────────────────────────┐
│ pose ┆ lla ┆ cellid ┆ vertices │
│ --- ┆ --- ┆ --- ┆ --- │
│ struct[3] ┆ struct[3] ┆ u64 ┆ struct[8] │
╞═════════════════════════╪═════════════════════════╪═════════════════════╪════════════════════════╡
│ {4190.667,14338.863,10. ┆ {37.732,55.820,163.916} ┆ 5094697078462873600 ┆ {37.304,55.491,40.932, │
│ 964} ┆ ┆ ┆ 57.545,36.… │
└─────────────────────────┴─────────────────────────┴─────────────────────┴────────────────────────┘
df.select("vertices").unnest("vertices")
shape: (1, 8)
┌────────┬────────┬────────┬────────┬────────┬────────┬────────┬────────┐
│ v0_lon ┆ v0_lat ┆ v1_lon ┆ v1_lat ┆ v2_lon ┆ v2_lat ┆ v3_lon ┆ v3_lat │
│ --- ┆ --- ┆ --- ┆ --- ┆ --- ┆ --- ┆ --- ┆ --- │
│ f64 ┆ f64 ┆ f64 ┆ f64 ┆ f64 ┆ f64 ┆ f64 ┆ f64 │
╞════════╪════════╪════════╪════════╪════════╪════════╪════════╪════════╡
│ 37.304 ┆ 55.491 ┆ 40.932 ┆ 57.545 ┆ 36.495 ┆ 59.135 ┆ 33.024 ┆ 56.886 │
└────────┴────────┴────────┴────────┴────────┴────────┴────────┴────────┘
distance
df = pl.DataFrame(
[
pl.Series("point_1", [{'x': -8893.663914126577, 'y': 19116.178523519542, 'z': 14.98697863612324}], dtype=pl.Struct({'x': pl.Float64, 'y': pl.Float64, 'z': pl.Float64})),
pl.Series("point_2", [{'x': 1553.3742543335538, 'y': 2916.118342842441, 'z': 15.580027717165649}], dtype=pl.Struct({'x': pl.Float64, 'y': pl.Float64, 'z': pl.Float64})),
]
)
df.with_columns(
distance=pl.col("point_1").distance.euclidean_3d(pl.col("point_2"))
)
shape: (1, 3)
┌──────────────────────────────┬────────────────────────────┬───────────┐
│ point_1 ┆ point_2 ┆ distance │
│ --- ┆ --- ┆ --- │
│ struct[3] ┆ struct[3] ┆ f64 │
╞══════════════════════════════╪════════════════════════════╪═══════════╡
│ {-8893.664,19116.179,14.987} ┆ {1553.374,2916.118,15.580} ┆ 19276.477 │
└──────────────────────────────┴────────────────────────────┴───────────┘
df.with_columns(
cosine_sim=pl.col("point_1").distance.cosine_similarity_3d(pl.col("point_2"))
)
shape: (1, 3)
┌──────────────────────────────┬────────────────────────────┬────────────┐
│ point_1 ┆ point_2 ┆ cosine_sim │
│ --- ┆ --- ┆ --- │
│ struct[3] ┆ struct[3] ┆ f64 │
╞══════════════════════════════╪════════════════════════════╪════════════╡
│ {-8893.664,19116.179,14.987} ┆ {1553.374,2916.118,15.580} ┆ 0.602 │
└──────────────────────────────┴────────────────────────────┴────────────┘
df.with_columns(
distance=pl.col("point_1").distance.euclidean_2d(pl.col("point_2"))
)
┌──────────────────────────────┬────────────────────────────┬───────────┐
│ point_1 ┆ point_2 ┆ distance │
│ --- ┆ --- ┆ --- │
│ struct[3] ┆ struct[3] ┆ f64 │
╞══════════════════════════════╪════════════════════════════╪═══════════╡
│ {-8893.664,19116.179,14.987} ┆ {1553.374,2916.118,15.580} ┆ 19276.477 │
└──────────────────────────────┴────────────────────────────┴───────────┘
shape: (1, 3)
┌──────────────────────────────┬────────────────────────────┬────────────┐
│ point_1 ┆ point_2 ┆ cosine_sim │
│ --- ┆ --- ┆ --- │
│ struct[3] ┆ struct[3] ┆ f64 │
╞══════════════════════════════╪════════════════════════════╪════════════╡
│ {-8893.664,19116.179,14.987} ┆ {1553.374,2916.118,15.580} ┆ 0.602 │
└──────────────────────────────┴────────────────────────────┴────────────┘
FAQs
Tools for coordinate transforms and S2-indexing via Polars Expression Plugins
We found that polars-coord-transforms demonstrated a healthy version release cadence and project activity because the last version was released less than a year ago. It has 1 open source maintainer collaborating on the project.
Did you know?
Socket for GitHub automatically highlights issues in each pull request and monitors the health of all your open source dependencies. Discover the contents of your packages and block harmful activity before you install or update your dependencies.
Security News
Research
The Socket Research Team breaks down a malicious wrapper package that uses obfuscation to harvest credentials and exfiltrate sensitive data.
Research
Security News
Attackers used a malicious npm package typosquatting a popular ESLint plugin to steal sensitive data, execute commands, and exploit developer systems.
Security News
The Ultralytics' PyPI Package was compromised four times in one weekend through GitHub Actions cache poisoning and failure to rotate previously compromised API tokens.