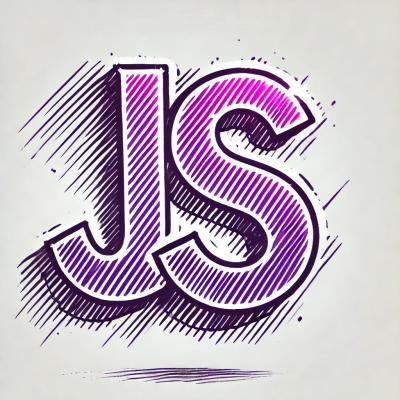
Security News
Oracle Drags Its Feet in the JavaScript Trademark Dispute
Oracle seeks to dismiss fraud claims in the JavaScript trademark dispute, delaying the case and avoiding questions about its right to the name.
The Seismology Benchmark collection (SeisBench) is an open-source python toolbox for machine learning in seismology. It provides a unified API for accessing seismic datasets and both training and applying machine learning algorithms to seismic data. SeisBench has been built to reduce the overhead when applying or developing machine learning techniques for seismological tasks.
SeisBench offers three core modules, data
, models
, and generate
.
data
provides access to benchmark datasets and offers functionality for loading datasets.
models
offers a collection of machine learning models for seismology.
You can easily create models, load pretrained models or train models on any dataset.
generate
contains tools for building data generation pipelines.
They bridge the gap between data
and models
.
The easiest way of getting started is through our colab notebooks.
Alternatively, you can clone the repository and run the same examples locally.
For more detailed information on Seisbench check out the SeisBench documentation.
SeisBench can be installed in two ways. In both cases, you might consider installing SeisBench in a virtual environment, for example using conda.
The recommended way is installation through pip. Simply run:
pip install seisbench
Alternatively, you can install the latest version from source. For this approach, clone the repository, switch to the repository root and run:
pip install .
which will install SeisBench in your current python environment.
SeisBench is built on pytorch, which in turn runs on CUDA for GPU acceleration. Sometimes, it might be preferable to install pytorch without CUDA, for example, because CUDA will not be used and the CUDA binaries are rather large. To install such a pure CPU version, the easiest way is to follow a two-step installation. First, install pytorch in a pure CPU version as explained here. Second, install SeisBench the regular way through pip. Example instructions would be:
pip install torch torchvision torchaudio --index-url https://download.pytorch.org/whl/cpu
pip install seisbench
There are many ways to contribute to SeisBench and we are always looking forward to your contributions. Check out the contribution guidelines for details on how to contribute.
seisbench.use_backup_repository()
.
Please note that the backup repository will usually show lower download speeds.
We recommend contacting your network administrator to allow outgoing access to TCP port 2880 on our server as a higher performance solution.import seisbench
from urllib.parse import urljoin
seisbench.remote_root = "https://hifis-storage.desy.de:2880/Helmholtz/HelmholtzAI/SeisBench/"
seisbench.remote_data_root = urljoin(seisbench.remote_root, "datasets/")
seisbench.remote_model_root = urljoin(seisbench.remote_root, "models/v3/")
pip install seisbench
.
As a workaround, follow the instructions at (https://pytorch.org/) to install pytorch and then install SeisBench as usual through pip.from_pretrained("original", version="3")
or from_pretrained("original", update=True)
. The weights will not differ in their predictions.Reference publications for SeisBench:
SeisBench - A Toolbox for Machine Learning in Seismology
Reference publication for software.
Which picker fits my data? A quantitative evaluation of deep learning based seismic pickers
Example of in-depth bencharking study of deep learning-based picking routines using the SeisBench framework.
The initial version of SeisBench has been developed at GFZ Potsdam and KIT with funding from Helmholtz AI. The SeisBench repository is hosted by HIFIS - Helmholtz Federated IT Services.
FAQs
The seismological machine learning benchmark collection
We found that seisbench demonstrated a healthy version release cadence and project activity because the last version was released less than a year ago. It has 2 open source maintainers collaborating on the project.
Did you know?
Socket for GitHub automatically highlights issues in each pull request and monitors the health of all your open source dependencies. Discover the contents of your packages and block harmful activity before you install or update your dependencies.
Security News
Oracle seeks to dismiss fraud claims in the JavaScript trademark dispute, delaying the case and avoiding questions about its right to the name.
Security News
The Linux Foundation is warning open source developers that compliance with global sanctions is mandatory, highlighting legal risks and restrictions on contributions.
Security News
Maven Central now validates Sigstore signatures, making it easier for developers to verify the provenance of Java packages.