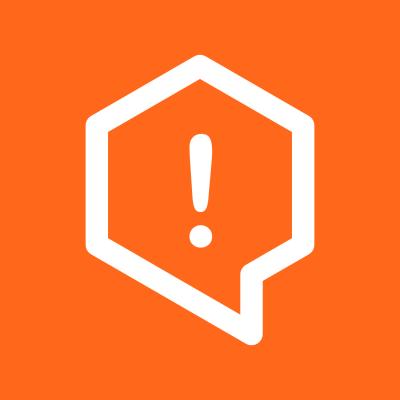
Security News
Fluent Assertions Faces Backlash After Abandoning Open Source Licensing
Fluent Assertions is facing backlash after dropping the Apache license for a commercial model, leaving users blindsided and questioning contributor rights.
TabPFN is a foundation model for tabular data that outperforms traditional methods while being dramatically faster. This repository contains the core PyTorch implementation with CUDA optimization.
⚠️ Major Update: Version 2.0: Complete codebase overhaul with new architecture and
features. Previous version available at v1.0.0 and
pip install tabpfn<2
.
📚 For detailed usage examples and best practices, check out Interactive Colab Tutorial
Choose the right TabPFN implementation for your needs:
Try our Interactive Colab Tutorial to get started quickly.
# Simple installation
pip install tabpfn
# Local development installation
git clone https://github.com/PriorLabs/TabPFN.git
pip install -e "TabPFN[dev]"
from sklearn.datasets import load_breast_cancer
from sklearn.metrics import accuracy_score, roc_auc_score
from sklearn.model_selection import train_test_split
from tabpfn import TabPFNClassifier
# Load data
X, y = load_breast_cancer(return_X_y=True)
X_train, X_test, y_train, y_test = train_test_split(X, y, test_size=0.33, random_state=42)
# Initialize a classifier
clf = TabPFNClassifier()
clf.fit(X_train, y_train)
# Predict probabilities
prediction_probabilities = clf.predict_proba(X_test)
print("ROC AUC:", roc_auc_score(y_test, prediction_probabilities[:, 1]))
# Predict labels
predictions = clf.predict(X_test)
print("Accuracy", accuracy_score(y_test, predictions))
For optimal performance, use the AutoTabPFNClassifier
or AutoTabPFNRegressor
for post-hoc ensembling. These can be found in the TabPFN Extensions repository. Post-hoc ensembling combines multiple TabPFN models into an ensemble.
Steps for Best Results:
Install the extensions:
git clone https://github.com/priorlabs/tabpfn-extensions.git
pip install -e tabpfn-extensions
from tabpfn_extensions.post_hoc_ensembles.sklearn_interface import AutoTabPFNClassifier
clf = AutoTabPFNClassifier(max_time=120) # 120 seconds tuning time
clf.fit(X_train, y_train)
predictions = clf.predict(X_test)
Prior Labs License (Apache 2.0 with additional attribution requirement)
@article{hollmann2025tabpfn,
title={Accurate predictions on small data with a tabular foundation model},
author={Hollmann, Noah and M{\"u}ller, Samuel and Purucker, Lennart and
Krishnakumar, Arjun and K{\"o}rfer, Max and Hoo, Shi Bin and
Schirrmeister, Robin Tibor and Hutter, Frank},
journal={Nature},
year={2025},
month={01},
day={09},
doi={10.1038/s41586-024-08328-6},
publisher={Springer Nature},
url={https://www.nature.com/articles/s41586-024-08328-6},
}
We're building the future of tabular machine learning and would love your involvement:
Connect & Learn:
Contribute:
Stay Updated: Star the repo and join Discord for the latest updates
python -m venv venv
source venv/bin/activate # On Windows: venv\Scripts\activate
git clone https://github.com/PriorLabs/TabPFN.git
cd tabpfn
pip install -e ".[dev]"
pre-commit install
pre-commit run --all-files
pytest tests/
Built with ❤️ by Prior Labs - Copyright (c) 2025 Prior Labs GmbH
FAQs
TabPFN: Foundation model for tabular data
We found that tabpfn demonstrated a healthy version release cadence and project activity because the last version was released less than a year ago. It has 2 open source maintainers collaborating on the project.
Did you know?
Socket for GitHub automatically highlights issues in each pull request and monitors the health of all your open source dependencies. Discover the contents of your packages and block harmful activity before you install or update your dependencies.
Security News
Fluent Assertions is facing backlash after dropping the Apache license for a commercial model, leaving users blindsided and questioning contributor rights.
Research
Security News
Socket researchers uncover the risks of a malicious Python package targeting Discord developers.
Security News
The UK is proposing a bold ban on ransomware payments by public entities to disrupt cybercrime, protect critical services, and lead global cybersecurity efforts.