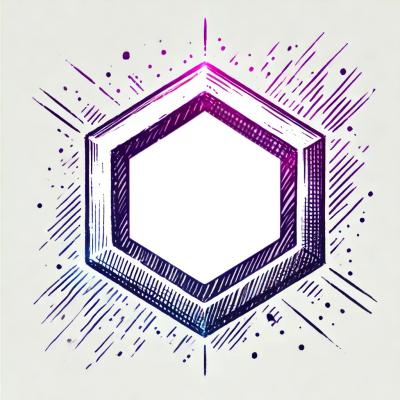
Security News
Maven Central Adds Sigstore Signature Validation
Maven Central now validates Sigstore signatures, making it easier for developers to verify the provenance of Java packages.
Those dict flavours that you have probably thought of at some point. Zero dependencies.
pip install those-dicts
Below you may find examples of behavior under normal dist-style usage of those_dicts. Essentially those are dicts but with a twist.
from those_dicts import BatchedDict, GraphDict, TwoWayDict, OOMDict
my_batched_dict = BatchedDict(nested=True)
client1 = dict(name='Lieutenant', surname='Kowalski',
address=dict(street='Funny Avenue', city='Elsewhere'))
client2 = dict(name='Thomas', surname='Dison',
address=dict(street='Lightbulb St.', city='Elsewhere'))
my_batched_dict.update(client1)
my_batched_dict.update(client2)
# >>> my_batched_dict['name']
# ['Lieutenant', 'Thomas']
# >>> my_batched_dict['address']
# {'street': ['Funny Avenue', 'Lightbulb St.'], 'city': ['Elsewhere', 'Elsewhere']}
my_graph_dict = GraphDict(Warsaw='Katowice', Katowice='Gdansk', Gdansk='Warsaw')
flights_to_germany = dict(Warsaw='Berlin', Katowice='Frankfurt')
flights_from_germany = dict(Berlin='Warsaw', Frankfurt='Katowice')
my_graph_dict.update(flights_to_germany)
my_graph_dict.update(flights_from_germany)
# >>> my_graph_dict['Warsaw']
# {'Berlin', 'Katowice'}
# >>> my_graph_dict['Berlin']
# 'Warsaw'
my_twoway_dict = TwoWayDict({('Eric', 'Doe'): ('Ella', 'Moon')})
# >>> my_twoway_dict[('Ella', 'Moon')] == ('Eric', 'Doe')
# True
# >>> my_twoway_dict[('Eric', 'Doe')] == ('Ella', 'Moon')
# True
new_marriage_after_divorce = {('Ella', 'Moon'): ('Benny', 'Hills')}
my_twoway_dict.update(new_marriage_after_divorce)
# >>> my_twoway_dict[('Ella', 'Moon')] == ('Benny', 'Hills')
# True
# >>> my_twoway_dict[('Eric', 'Doe')] is None
# True
from some_lib import ObjWithDefinedSize
my_oom_dict = OOMDict(max_ram_entries=10)
my_oom_dict.update([str(k): ObjWithDefinedSize(mb_size=k) for k in range(1000)])
# first 10 objects are in RAM, the rest is on the disk
del my_oom_dict # clears the disk also
When you want to aggregate multiple dicts:
from those_dicts import BatchedDict
my_batched_dict = BatchedDict()
my_batched_nested = BatchedDict(nested=True)
client1 = dict(name='Lieutenant', surname='Kowalski',
address=dict(street='Funny Avenue', city='Elsewhere'))
client2 = dict(name='Thomas', surname='Dison',
address=dict(street='Lightbulb St.', city='Elsewhere'))
my_batched_dict.update(client1)
my_batched_dict.update(client2)
my_batched_nested.update(client1)
my_batched_nested.update(client2)
# or equivalently, because it is a dict
my_batched_dict = BatchedDict(name='Lieutenant', surname='Kowalski',
address=dict(street='Funny Avenue', city='Elsewhere'))
my_batched_nested = BatchedDict(nested=True, name='Lieutenant', surname='Kowalski',
address=dict(street='Funny Avenue', city='Elsewhere'))
my_batched_dict.update(client2)
my_batched_nested.update(client2)
# >>> my_batched_dict
# {'name': ['Lieutenant', 'Thomas'], 'surname': ['Kowalski', 'Dison'], 'address': [{'street': 'Funny Avenue', 'city': 'Elsewhere'}, {'street': 'Lightbulb St.', 'city': 'Elsewhere'}]}
# >>> my_batched_nested
# {'name': ['Lieutenant', 'Thomas'], 'surname': ['Kowalski', 'Dison'], 'address': {'street': ['Funny Avenue', 'Lightbulb St.'], 'city': ['Elsewhere', 'Elsewhere']}}
# straightforward aggregation use case
my_batched_dict = BatchedDict()
my_batched_dict['john_properties'] = 'car'
my_batched_dict['john_properties'] = 'bike'
my_batched_dict['john_properties'] = 'grill'
my_batched_dict['john_properties'] = 'gaming pc'
# >>> my_batched_dict['john_properties']
# ['car', 'bike', 'grill', 'gaming pc']
# >>> my_batched_dict['john_properties'].remove('grill')
# >>> my_batched_dict['john_properties']
# ['car', 'bike', 'gaming pc']
my_batched_dict['ella_properties'] = 'house'
my_batched_dict['ella_properties'] = 'garage'
# >>> my_batched_dict['ella_properties']
# ['house', 'garage']
Essentially it is a dict, so usage is intuitive.
When you want to create a mapping from one hashable to another hashable that may traverse further.
from dataclasses import dataclass
from those_dicts import GraphDict
@dataclass(frozen=True)
class Building:
coordinates: tuple[float, float]
address: str
elevation: float
purpose: str
history: str
# some big, hashable data structure
@dataclass(frozen=True)
class City:
name: str
country: str
area: float
population: int
top_10_buildings: frozenset[Building]
warsaw = City('Warsaw', ...)
katowice = ... # you get the point
gdansk = ...
berlin = ...
frankfurt = ...
my_graph_dict = GraphDict({warsaw: katowice, katowice: gdansk, gdansk: warsaw})
flights_to_germany = {warsaw: berlin, katowice: frankfurt}
flights_from_germany = {berlin: warsaw, frankfurt: katowice}
my_graph_dict.update(flights_to_germany)
my_graph_dict.update(flights_from_germany)
# >>> my_graph_dict[warsaw]
# {berlin, katowice}
# >>> my_graph_dict[berlin]
# warsaw
# >>> my_graph_dict
# {katowice: {2, 4}, warsaw: {0, 3}, gdansk: {1}, berlin: {1}, frankfurt: {0}}
GraphDict stores each hashable object only once - here everything is a key. Values are just index-wise references. This means a lot of memory savings for storing big objects.
GraphDict is compatible with dict, but with a twist(s) enlisted below:
It is a subclass of GraphDict that is restricted to have only exclusive two-way connections.
You can access value through its key and other way around.
Compared to GraphDict, .merge() and .make_loops() are raising NotImplementedError as those doesn't make sense for this class.
When you want to limit impact on RAM.
from those_dicts import OOMDict
my_oom_dict = OOMDict(max_ram_entries=10000) # the default
for name, big_obj in big_obj_generator(num_obj=1000000):
my_oom_dict[name] = big_obj
# everything above 10000 objects will be stored on the disk
Even if storage is split between RAM and disk, it is just a dict, so use it as usual.
FAQs
Those missing flavors of dict
We found that those-dicts demonstrated a healthy version release cadence and project activity because the last version was released less than a year ago. It has 1 open source maintainer collaborating on the project.
Did you know?
Socket for GitHub automatically highlights issues in each pull request and monitors the health of all your open source dependencies. Discover the contents of your packages and block harmful activity before you install or update your dependencies.
Security News
Maven Central now validates Sigstore signatures, making it easier for developers to verify the provenance of Java packages.
Security News
CISOs are racing to adopt AI for cybersecurity, but hurdles in budgets and governance may leave some falling behind in the fight against cyber threats.
Research
Security News
Socket researchers uncovered a backdoored typosquat of BoltDB in the Go ecosystem, exploiting Go Module Proxy caching to persist undetected for years.