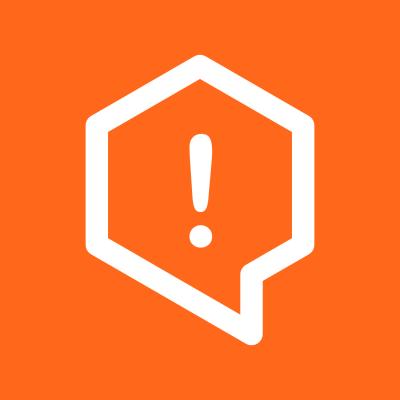
Security News
Fluent Assertions Faces Backlash After Abandoning Open Source Licensing
Fluent Assertions is facing backlash after dropping the Apache license for a commercial model, leaving users blindsided and questioning contributor rights.
当前版本完全兼容huggingface接口,支持计算优化、TP/PP/EP混合并行优化,不需要额外的模型转换操作
Dependencies: pytorch >= 2.0.0
支持显卡:Ampere, Ada, or Hopper GPUs (e.g., A100, A800, H100, H800, H20)
当前版本完全兼容huggingface接口,只需要2行代码即可开启计算优化
### TILEARN.LLM monkey patch
from tilearn.llm.transformers.models import patch_models
patch_models()
### 通过Auto factory初始化模型,接口与标准huggingface一致
model = AutoModelForCausalLM.from_pretrained(...)
### or直接初始化模型
model = LlamaForCausalLM.from_pretrained(...)
目前Monkey-Patch支持的模型列表:
LlamaForCausalLM
Qwen2ForCausalLM
### TILEARN.LLM
from tilearn.llm.transformers import LlamaForCausalLM
### 模型接口与标准huggingface一致
model = LlamaForCausalLM.from_pretrained(...)
或者使用AutoModelForCausalLM接口
### TILEARN.LLM
from tilearn.llm.transformers import AutoModelForCausalLM
### 模型接口与标准huggingface一致
model = AutoModelForCausalLM.from_pretrained(...)
特殊说明:
### TILEARN_LLM_BAICHUAN_13B open baichuan2 model
export TILEARN_LLM_BAICHUAN_13B=2
# llama
from tilearn.llm.transformers.models.llama.modeling_llama import LlamaForCausalLM
# bloom
from tilearn.llm.transformers.models.bloom.modeling_bloom import BloomForCausalLM
# baichuan1
from tilearn.llm.transformers.models.baichuan.baichuan1_13B.modeling_baichuan import BaichuanForCausalLM
from tilearn.llm.transformers.models.baichuan.baichuan1_7B.modeling_baichuan import BaiChuanForCausalLM
# baichuan2
# 默认使用TILEARN.LLM且无需任何设置
# 单独使用xformer,需安装xformer且设置环境变量TIACC_TRAINING_CUDA_KERNEL=2
from tilearn.llm.transformers.models.baichuan.baichuan2_7B.modeling_baichuan import BaichuanForCausalLM
from tilearn.llm.transformers.models.baichuan.baichuan2_13B.modeling_baichuan import BaichuanForCausalLM
# aquila2
from tilearn.llm.transformers.models.aquila.aquila2.modeling_aquila import AquilaForCausalLM
当前版本完全兼容huggingface trainer生态,只需要2行代码即可开启TP、PP、EP混合并行。无需进行模型转换
示例1(Llama/Qwen等非MoE结构)
### 混合并行
export TILEARN_DEBUG=1
export TILEARN_HYBRID_TP_SIZE=2
export TILEARN_HYBRID_PP_SIZE=2
#export TILEARN_HYBRID_OFFLOAD=0
#export TILEARN_HYBRID_ZERO_STAGE=1
示例2(MixtralMoE)
### 混合并行
export TILEARN_DEBUG=1
export TILEARN_HYBRID_MODE='MixtralMoe'
export TILEARN_HYBRID_TP_SIZE=1
export TILEARN_HYBRID_PP_SIZE=2
export TILEARN_HYBRID_EP_SIZE=2
export TILEARN_HYBRID_OFFLOAD=0
export TILEARN_HYBRID_ZERO_STAGE=1
示例3(HunYuanMoE)
### 混合并行
export TILEARN_DEBUG=1
export TILEARN_HYBRID_MODE='HunyuanMoe'
export TILEARN_HYBRID_TP_SIZE=1
export TILEARN_HYBRID_PP_SIZE=4
export TILEARN_HYBRID_EP_SIZE=2 # 专家并行
export TILEARN_HYBRID_OFFLOAD=0
export TILEARN_HYBRID_ZERO_STAGE=1
import tilearn.llm.hybrid_parallel
示例
### (可选)开启混合并行
import tilearn.llm.hybrid_parallel
### (可选)导入计算优化
from tilearn.llm.transformers.models import patch_models
### (可选)cpu 内存优化
# from tilearn.llm.memory.cpu.memory_optimize import memory_optimize
def main():
### (可选)导入计算优化
patch_models()
### (可选)cpu 内存优化
# memory_optimize()
run_exp()
if __name__ == "__main__":
main()
Qwen2.5 32B | GPU num | mbs | global bs | seqlength | GPU Mem | tokens / sec / gpu |
---|---|---|---|---|---|---|
deepspeed zero3 g-ckpt | 16 | 1 | 64 | 4096 | 86 GB | 427 |
tilearn.llm TP4 PP2 zero1 | 16 | 1 | 64 | 4096 | 76 GB | 515 |
其他数据见1.5节
适用于显存有限,zero3+offload场景显存优化
### AutoZero
export TILEARN_DEBUG=1
export TILEARN_HYBRID_MODE='AutoZero'
export TILEARN_HYBRID_AUTOZERO_SHARD_PARAM=1
export TILEARN_HYBRID_AUTOZERO_OFFLOAD_OPTIM=1
export TILEARN_HYBRID_AUTOZERO_OFFLOAD_PARAM=1
Qwen2 72B | GPU num | mbs | global bs | seqlength | GPU Mem | tokens / sec / gpu |
---|---|---|---|---|---|---|
deepspeed zero3 offload | 8 | 1 | 16 | 4096 | 42 GB | 136 |
tilearn.llm autozero offload | 8 | 1 | 16 | 4096 | 22 GB | 135 |
适用场景:huggingface transformers + trainer模型
自动编译优化,在main.py添加如下代码即可开启,目前还在实验阶段
import tilearn.llm.compile
目前已支持手工CUDA算子+自动编译优化,若要关闭手工CUDA算子,则添加以下环境变量
export TILEARN_COMPILE_MODELPATCH=0
适用范围单机8卡、多机多卡。用法上相对通用与具体模型无关,但加速收益和模型相关
from tilearn.llm.cpu_affinity import cpu_affinity
def main():
cpu_affinity()
main()
某自动驾驶场景模型加速效果
Method | GPU num | mbs | sec / step | speedup |
---|---|---|---|---|
baseline | 48 | 2 | 2.55 | 1 |
tilearn.llm | 48 | 2 | 1.91 | 1.34 |
适用范围单机8卡、多机多卡。用法上相对通用与具体模型无关,但加速收益和模型相关
from tilearn.llm.gc_optimize import gc_optimize
def main():
gc_optimize()
main()
某自动驾驶场景模型加速效果
Method | GPU num | mbs | sec / step | speedup |
---|---|---|---|---|
baseline | 32 | 64 | 1.74 | 1 |
tilearn.llm | 32 | 64 | 1.13 | 1.54 |
训练加速中的通信加速能力通过兼容原生的DDP工具提供,用户无需修改原生的使用代码可直接进行使用,数据IO优化、自适应FP16都通过封装好的简单函数/类进行提供,用户仅需增加几行代码便可使用。
适用范围:多机多卡 以兼容原生DDP的方式启动训练脚本,无需进行训练代码的修改,启动命令参考示例如下: 在启动脚本start.sh内使用tiaccrun替换torchrun,接口与pytorch torchrun完全一致
export NODE_NUM=1
export INDEX=0
export GPU_NUM_PER_NODE=1
export MASTER_ADDR=127.0.0.1
export MASTER_PORT=23458
tiaccrun \
--nnodes $NODE_NUM \
--node_rank $INDEX \
--nproc_per_node $GPU_NUM_PER_NODE \
--master_addr $MASTER_ADDR \
--master_port $MASTER_PORT \
xxx.py
tilearnrun \
--nnodes $NODE_NUM \
--node_rank $INDEX \
--nproc_per_node $GPU_NUM_PER_NODE \
--master_addr $MASTER_ADDR \
--master_port $MASTER_PORT \
xxx.py
DDP分布式训练通信优化实测效果: (加速效果在多机多卡场景方有体现,单机多卡场景与原生DDP性能无异。)
硬件环境 | 模型 | GPU卡数 | 原生DDP(examples/sec per V100) | TI-ACC通信优化(examples/sec per V100) |
---|---|---|---|---|
腾讯云GN10Xp.20XLARGE320 | resnext50_32x4d | 1(单机) | 227 | 227 |
腾讯云GN10Xp.20XLARGE320 | resnext50_32x4d | 8(单机) | 215 | 215 |
腾讯云GN10Xp.20XLARGE320 | resnext50_32x4d | 16(双机) | 116 | 158.6 |
适用范围:单机单卡、单机多卡、多级多卡
import torch
from tilearn.llm.torch.optimizers import FusedSGD
from tilearn.llm.torch.optimizers import FusedAdam
from tilearn.llm.torch.optimizers import FusedLAMB
from tilearn.llm.torch.optimizers import FusedAdagrad
nelem = 1
tensor = torch.rand(nelem, dtype=torch.float, device="cuda")
param = []
param.append(torch.nn.Parameter(tensor.clone()))
sgd_options = {"lr": .25, "momentum": .125}
optimizer =FusedSGD(param, **sgd_options)
optimizer =FusedAdam(param)
optimizer =FusedLAMB(param)
optimizer =FusedAdagrad(param)
FusedSGD接口
class FusedSGD(Optimizer):
def __init__(self, params, lr=required, momentum=0,
dampening=0, weight_decay=0, nesterov=False)
FusedAdam接口
class FusedAdam(Optimizer):
def __init__(self, params, lr=1e-3, bias_correction=True,
betas=(0.9, 0.999), eps=1e-8, adam_w_mode=True,
weight_decay=0., amsgrad=False)
FusedLAMB接口
class FusedLAMB(Optimizer):
def __init__(self, params, lr=1e-3, bias_correction=True,
betas=(0.9, 0.999), eps=1e-6, weight_decay=0.01,
amsgrad=False, adam_w_mode=True,
max_grad_norm=1.0):
FusedAdagrad接口
class FusedAdagrad(Optimizer):
def __init__(self, params, lr=1e-2, eps=1e-10,
weight_decay=0., adagrad_w_mode=False):
适用范围:开启torch amp后,loss不收敛或模型效果下降时,使用tiacc_training amp接口提升模型效果
import torch
from tilearn.llm.torch.adapt_amp import MixedPrecision_TrainingPolicy
def main():
#使用tiacc自适应混合精度
scaler = torch.cuda.amp.GradScaler()
#实例化tiacc自适应混合精度策略类的对象
schedulePolicy = "TimeSchedulePolicy"
policy = MixedPrecision_TrainingPolicy(
policy=schedulePolicy,
start_time=0, end_time=40)
#根据输入的参数得到当前epoch是否需要开启混合精度
for epoch in range(0, 51):
mixed_precision = policy.enable_mixed_precision(epoch,
scaler=scaler)
print(mixed_precision)
#with amp.autocast(enabled=mixed_precision):
# outputs = model(inputs)
# loss = criterion(outputs, targets)
#scaler.scale(loss).backward()
#scaler.step(optimizer)
#scaler.update()
main()
实现对训练过程中自动混合精度自适应策略的实例化,自适应策略包括时间混合精度、时间学习率混合精度策略、损失函数混合精度策略。
初始化参数:
是否必填 | 参数说明 | 示例 | 默认值 |
---|---|---|---|
是 | 自适应混合精度策略,0:时间混合精度,适用于通用自适应情况; 1:时间学习率混合精度策略,适用于训练过程中某一阶段loss波动出现异常的情况; 2:损失函数混合精度策略,适用于训练过程中loss下降过快或过慢情况。 | 0 | 无 |
否 | 开启自适应混合精度的开始时间,一般建议设为10。策略为0和1时必填,为2时非必填。 | 10 | 10 |
否 | 开启自适应混合精度的结束时间,一般建议设为最后一个epoch时间。策略为0和1时必填,为2时非必填。 | 1000 None | |
否 | 开启策略1时的保持时间,在保持时间内采用统一策略:开启或者不开启。一般建议为训练过程中loss异常波动的持续时间。策略为1时必填,为0和2时非必填。 | 20 | None |
否 | 开启策略2的间隔时间,默认值为1000,即每间隔1000轮epoch开启策略2。策略为2时需要填写,为0和1时无需必填。 | 1000 | 1000 |
否 | 在interval_time间隔时间开启策略2后的保持时间,默认值为100,如interval_time为1000,即在1000-1100,2000-2100...开启策略2。策略为2时需要填写,为0和1时无需必填。 | 100 | 100 |
policy实例化对象:
对象 | 类型 | 对象说明 |
---|---|---|
policy | MixedPrecision_TrainingPolicy类 | 训练过程中自动混合精度自适应策略的实例化对象 |
属于MixedPrecision_TrainingPolicy类,根据输入的参数得到当前epoch是否需要开启自动混合精度。 输入参数:
参数 | 类型 | 是否必填 | 参数说明 | 示例 | 默认值 |
---|---|---|---|---|---|
epoch | INT | 是 | 当前的epoch | 20 | 无 |
scaler | torch.cuda.amp.GradScaler | 是 | 梯度缩放实例化对象 | scaler | 无 |
lr | float | 否 | lr是当前epoch的学习率 | 0.01 | None |
loss | float | 否 | loss是上一轮epoch的损失值 | 0.1 | None |
输出参数:
输出参数 | 类型 | 参数说明 |
---|---|---|
mixed_precision | BOOL | 输入的参数得到当前epoch是否需要开启自动混合精度,是返回TRUE,否则返回FLASE。 |
FAQs
TILEARN for LLM
We found that tilearn-llm demonstrated a healthy version release cadence and project activity because the last version was released less than a year ago. It has 1 open source maintainer collaborating on the project.
Did you know?
Socket for GitHub automatically highlights issues in each pull request and monitors the health of all your open source dependencies. Discover the contents of your packages and block harmful activity before you install or update your dependencies.
Security News
Fluent Assertions is facing backlash after dropping the Apache license for a commercial model, leaving users blindsided and questioning contributor rights.
Research
Security News
Socket researchers uncover the risks of a malicious Python package targeting Discord developers.
Security News
The UK is proposing a bold ban on ransomware payments by public entities to disrupt cybercrime, protect critical services, and lead global cybersecurity efforts.