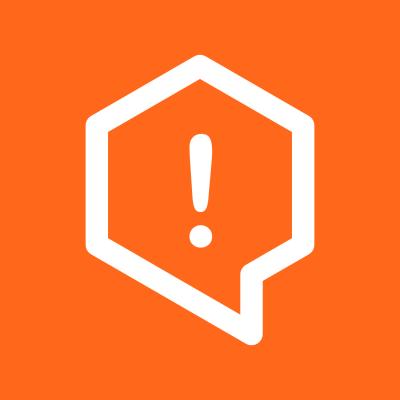
Security News
Fluent Assertions Faces Backlash After Abandoning Open Source Licensing
Fluent Assertions is facing backlash after dropping the Apache license for a commercial model, leaving users blindsided and questioning contributor rights.
torchextractor
: PyTorch Intermediate Feature ExtractionToo many times some model definitions get remorselessly copy-pasted just because the
forward
function does not return what the person expects. You provide module names
and torchextractor
takes care of the extraction for you.It's never been easier to
extract feature, add an extra loss or plug another head to a network.
Ler us know what amazing things you build with torchextractor
!
pip install torchextractor # stable
pip install git+https://github.com/antoinebrl/torchextractor.git # latest
Requirements:
import torch
import torchvision
import torchextractor as tx
model = torchvision.models.resnet18(pretrained=True)
model = tx.Extractor(model, ["layer1", "layer2", "layer3", "layer4"])
dummy_input = torch.rand(7, 3, 224, 224)
model_output, features = model(dummy_input)
feature_shapes = {name: f.shape for name, f in features.items()}
print(feature_shapes)
# {
# 'layer1': torch.Size([1, 64, 56, 56]),
# 'layer2': torch.Size([1, 128, 28, 28]),
# 'layer3': torch.Size([1, 256, 14, 14]),
# 'layer4': torch.Size([1, 512, 7, 7]),
# }
• How do I know the names of the modules?
You can print all module names like this:
tx.list_module_names(model)
# OR
for name, module in model.named_modules():
print(name)
• Why do some operations not get listed?
It is not possible to add hooks if operations are not defined as modules.
Therefore, F.relu
cannot be captured but nn.Relu()
can.
• How can I avoid listing all relevant modules?
You can specify a custom filtering function to hook the relevant modules:
# Hook everything !
module_filter_fn = lambda module, name: True
# Capture of all modules inside first layer
module_filter_fn = lambda module, name: name.startswith("layer1")
# Focus on all convolutions
module_filter_fn = lambda module, name: isinstance(module, torch.nn.Conv2d)
model = tx.Extractor(model, module_filter_fn=module_filter_fn)
• Is it compatible with ONNX?
tx.Extractor
is compatible with ONNX! This means you can also access intermediate features maps after the export.
Pro-tip: name the output nodes by using output_names
when calling torch.onnx.export
.
• Is it compatible with TorchScript?
Bad news, TorchScript cannot take variable number of arguments and keyword-only arguments.
Good news, there is a workaround! The solution is to overwrite the forward
function
of tx.Extractor
to replicate the interface of the model.
import torch
import torchvision
import torchextractor as tx
class MyExtractor(tx.Extractor):
def forward(self, x1, x2, x3):
# Assuming the model takes x1, x2 and x3 as input
output = self.model(x1, x2, x3)
return output, self.feature_maps
model = torchvision.models.resnet18(pretrained=True)
model = MyExtractor(model, ["layer1", "layer2", "layer3", "layer4"])
model_traced = torch.jit.script(model)
• "One more thing!" :wink: By default we capture the latest output of the relevant modules, but you can specify your own custom operations.
For example, to accumulate features over 10 forward passes you can do the following:
import torch
import torchvision
import torchextractor as tx
model = torchvision.models.resnet18(pretrained=True)
def capture_fn(module, input, output, module_name, feature_maps):
if module_name not in feature_maps:
feature_maps[module_name] = []
feature_maps[module_name].append(output)
extractor = tx.Extractor(model, ["layer3", "layer4"], capture_fn=capture_fn)
for i in range(20):
for i in range(10):
x = torch.rand(7, 3, 224, 224)
model(x)
feature_maps = extractor.collect()
# Do your stuffs here
# Discard collected elements
extractor.clear_placeholder()
All feedbacks and contributions are welcomed. Feel free to report an issue or to create a pull request!
If you want to get hands-on:
virtualenv -p python3 .venv && source .venv/bin/activate
pip install -r requirements.txt && pip install -r requirements-dev.txt
pre-commit install
python -m unittest discover -vs ./tests/
To Build documentation:
cd docs
pip install requirements.txt
make html
FAQs
Pytorch feature extraction made simple
We found that torchextractor demonstrated a healthy version release cadence and project activity because the last version was released less than a year ago. It has 1 open source maintainer collaborating on the project.
Did you know?
Socket for GitHub automatically highlights issues in each pull request and monitors the health of all your open source dependencies. Discover the contents of your packages and block harmful activity before you install or update your dependencies.
Security News
Fluent Assertions is facing backlash after dropping the Apache license for a commercial model, leaving users blindsided and questioning contributor rights.
Research
Security News
Socket researchers uncover the risks of a malicious Python package targeting Discord developers.
Security News
The UK is proposing a bold ban on ransomware payments by public entities to disrupt cybercrime, protect critical services, and lead global cybersecurity efforts.