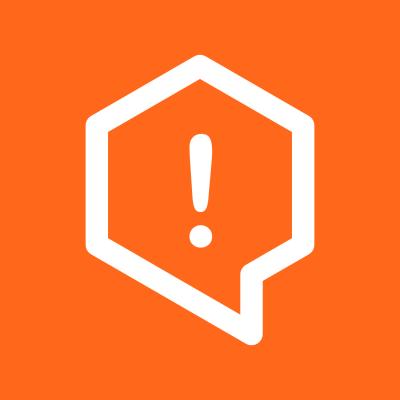
Security News
Fluent Assertions Faces Backlash After Abandoning Open Source Licensing
Fluent Assertions is facing backlash after dropping the Apache license for a commercial model, leaving users blindsided and questioning contributor rights.
A python library for timeseries smoothing and outlier detection in a vectorized way.
A python library for time-series smoothing and outlier detection in a vectorized way.
tsmoothie computes, in a fast and efficient way, the smoothing of single or multiple time-series.
The smoothing techniques available are:
tsmoothie provides the calculation of intervals as result of the smoothing process. This can be useful to identify outliers and anomalies in time-series.
In relation to the smoothing method used, the interval types available are:
tsmoothie can carry out a sliding smoothing approach to simulate an online usage. This is possible splitting the time-series into equal sized pieces and smoothing them independently. As always, this functionality is implemented in a vectorized way through the WindowWrapper class.
tsmoothie can operate time-series bootstrap through the BootstrappingWrapper class.
The supported bootstrap algorithms are:
Blog Posts:
pip install --upgrade tsmoothie
The module depends only on NumPy, SciPy and simdkalman. Python 3.6 or above is supported.
Below a couple of examples of how tsmoothie works. Full examples are available in the notebooks folder.
# import libraries
import numpy as np
import matplotlib.pyplot as plt
from tsmoothie.utils_func import sim_randomwalk
from tsmoothie.smoother import LowessSmoother
# generate 3 randomwalks of lenght 200
np.random.seed(123)
data = sim_randomwalk(n_series=3, timesteps=200,
process_noise=10, measure_noise=30)
# operate smoothing
smoother = LowessSmoother(smooth_fraction=0.1, iterations=1)
smoother.smooth(data)
# generate intervals
low, up = smoother.get_intervals('prediction_interval')
# plot the smoothed timeseries with intervals
plt.figure(figsize=(18,5))
for i in range(3):
plt.subplot(1,3,i+1)
plt.plot(smoother.smooth_data[i], linewidth=3, color='blue')
plt.plot(smoother.data[i], '.k')
plt.title(f"timeseries {i+1}"); plt.xlabel('time')
plt.fill_between(range(len(smoother.data[i])), low[i], up[i], alpha=0.3)
# import libraries
import numpy as np
import matplotlib.pyplot as plt
from tsmoothie.utils_func import sim_seasonal_data
from tsmoothie.smoother import DecomposeSmoother
# generate 3 periodic timeseries of lenght 300
np.random.seed(123)
data = sim_seasonal_data(n_series=3, timesteps=300,
freq=24, measure_noise=30)
# operate smoothing
smoother = DecomposeSmoother(smooth_type='lowess', periods=24,
smooth_fraction=0.3)
smoother.smooth(data)
# generate intervals
low, up = smoother.get_intervals('sigma_interval')
# plot the smoothed timeseries with intervals
plt.figure(figsize=(18,5))
for i in range(3):
plt.subplot(1,3,i+1)
plt.plot(smoother.smooth_data[i], linewidth=3, color='blue')
plt.plot(smoother.data[i], '.k')
plt.title(f"timeseries {i+1}"); plt.xlabel('time')
plt.fill_between(range(len(smoother.data[i])), low[i], up[i], alpha=0.3)
All the available smoothers are fully integrable with sklearn (see here).
# import libraries
import numpy as np
import matplotlib.pyplot as plt
from tsmoothie.utils_func import sim_seasonal_data
from tsmoothie.smoother import ConvolutionSmoother
from tsmoothie.bootstrap import BootstrappingWrapper
# generate a periodic timeseries of lenght 300
np.random.seed(123)
data = sim_seasonal_data(n_series=1, timesteps=300,
freq=24, measure_noise=15)
# operate bootstrap
bts = BootstrappingWrapper(ConvolutionSmoother(window_len=8, window_type='ones'),
bootstrap_type='mbb', block_length=24)
bts_samples = bts.sample(data, n_samples=100)
# plot the bootstrapped timeseries
plt.figure(figsize=(13,5))
plt.plot(bts_samples.T, alpha=0.3, c='orange')
plt.plot(data[0], c='blue', linewidth=2)
FAQs
A python library for timeseries smoothing and outlier detection in a vectorized way.
We found that tsmoothie demonstrated a healthy version release cadence and project activity because the last version was released less than a year ago. It has 1 open source maintainer collaborating on the project.
Did you know?
Socket for GitHub automatically highlights issues in each pull request and monitors the health of all your open source dependencies. Discover the contents of your packages and block harmful activity before you install or update your dependencies.
Security News
Fluent Assertions is facing backlash after dropping the Apache license for a commercial model, leaving users blindsided and questioning contributor rights.
Research
Security News
Socket researchers uncover the risks of a malicious Python package targeting Discord developers.
Security News
The UK is proposing a bold ban on ransomware payments by public entities to disrupt cybercrime, protect critical services, and lead global cybersecurity efforts.