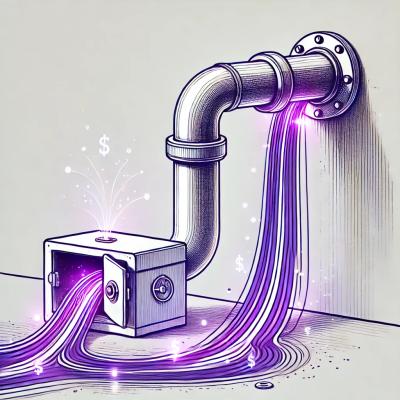
Research
Security News
Malicious npm Package Targets Solana Developers and Hijacks Funds
A malicious npm package targets Solana developers, rerouting funds in 2% of transactions to a hardcoded address.
Vision-algorithms Requests Processing Wrappers for deep-learning Computer Vision algorithms on the cloud.
VRPWRP (Vision-algorithm Requests Processing Wrappers) is a package that wraps an API-REST for Computer Vision deep-learning algorithms. Currently, it supports state-of-the-art face-detection and face-recognition algorithms out-of-the-box.
.. image:: https://badge.fury.io/py/vrpwrp.svg :target: https://badge.fury.io/py/vrpwrp .. image:: https://travis-ci.org/ipazc/vrpwrp.svg?branch=master :target: https://travis-ci.org/ipazc/vrpwrp .. image:: https://coveralls.io/repos/github/ipazc/vrpwrp/badge.svg?branch=master :target: https://coveralls.io/github/ipazc/vrpwrp?branch=master .. image:: https://landscape.io/github/ipazc/vrpwrp/master/landscape.svg?style=flat :target: https://landscape.io/github/ipazc/vrpwrp/master :alt: Code Health
Currently it is only supported Python 3.4.1 onwards:
.. code:: bash
sudo pip3 install vrpwrp
Face detection allows you to retrieve the location of faces inside images in the form of bounding boxes (left, top, width, height). The algorihm is a deep-learning based algorithm, composed by a cascade of Convolutional Neural Networks. It is based on the paper Zhang et al. (2016) [ZHANG2016]. The backend runs a Caffe-based MTCNN influenced by this python MTCNN version <https://github.com/DuinoDu/mtcnn>
.
A simple example for retrieving the bounding boxes of faces from an image:
.. code:: python
>>> from vrpwrp.wrappers.face_detection import FaceDetection
>>> face_detection = FaceDetection()
>>> bounding_boxes = face_detection.analyze_file("route/to/image.jpg")
>>> for bb in bounding_boxes: print(bb)
...
[162, 79, 114, 146]
FaceDetection has methods for analyzing images also from bytes, URLs and pillow images directly:
.. code:: python
>>> bounding_boxes = face_detection.analyze_bytes(image_bytes)
>>> bounding_boxes = face_detection.analyze_url(image_url)
>>> bounding_boxes = face_detection.analyze_pil(pillow_image)
...
Face recognition allows extracting the identity of a face within a given image of the face. The identity is a set of float numbers (since it is deep-learning-based, it is the output of the last convolution layer of a Convolutional Neural Network). The algorithm is based on the papers Schroff et al. (2015) [SCHROFF2015], Wen et al. (2016) [WEN2016]. and Parkhi et al. (2015) [PARKHI2015]. The backend is influenced by Facenet <https://github.com/davidsandberg/facenet>
, using TensorFlow.
In vrpwrp, the identity of a face is also known as embeddings.
A simple example for retrieving the embeddings of a face is:
.. code:: python
>>> from vrpwrp.wrappers.face_recognition import FaceRecognition
>>> face_recognition = FaceRecognition()
>>> face_embeddings = face_recognition.get_embeddings_from_file("route/to/image_of_face.jpg")
>>> print(face_embeddings)
[-0.05258641 -0.14807236 0.21828972 0.00097196 0.08881456 0.01356898 -0.01393933 -0.09459263 -0.07305822 0.00354048 0.1649337 -0.05636634 0.03599492 -0.02649886 ...]
Like in FaceDetection, it allows to analyze images from different sources:
.. code:: python
>>> embeddings = face_recognition.get_embeddings_from_bytes(image_bytes)
>>> embeddings = face_recognition.get_embeddings_from_url(image_url)
>>> embeddings = face_recognition.get_embeddings_from_pil(pillow_image)
...
The embeddings of two faces can be easily compared to see how close they are:
.. code:: python
>>> face1_embeddings = face_recognition.get_embeddings_from_file("route/to/image_of_face1.jpg")
>>> face2_embeddings = face_recognition.get_embeddings_from_file("route/to/image_of_face2.jpg")
>>> print(face1_embeddings - face2_embeddings)
0.5634614628831894
A value close to 0 indicates that two faces might be of the same person. In this example, image_of_face1.jpg and image_of_face2.jpg are likely to be of the same person. Otherwise, a value over 1.0 might indicate that two faces are not likely to be of the same person.
This might lead to a scenario where you store lot of embeddings and want to compare a single one with each of them, resulting in a loop like the following:
.. code:: python
faces_embeddings = [emb1, emb2, ..., embN]
new_embedding = face_recognition.get_embeddings_from_file("route/to/image_of_face1.jpg")
for embedding in faces_embeddings:
distance = embedding - new_embedding
Rather than using a loop (even if it is a list-comprehension), there is an optimized and preferred way of performing such a comparison that can be used instead:
.. code:: python
faces_embeddings = [emb1, emb2, ..., embN]
new_embedding = face_recognition.get_embedding_from_file("route/to/image_of_face1.jpg")
distances = face_recognition.get_embeddings_distances(new_embedding, faces_embeddings)
.. [ZHANG2016] Zhang, K., Zhang, Z., Li, Z., and Qiao, Y. (2016). Joint face detection and alignment using multitask cascaded convolutional networks. IEEE Signal Processing Letters, 23(10):1499–1503.
.. [SCHROFF2015] Schroff, F., Kalenichenko, D., & Philbin, J. (2015). Facenet: A unified embedding for face recognition and clustering. In Proceedings of the IEEE Conference on CVPR (pp. 815-823).
.. [WEN2016] Wen, Y., Zhang, K., Li, Z., & Qiao, Y. (2016, October). A discriminative feature learning approach for deep face recognition. In ECCV (pp. 499-515). Springer International Publishing.
.. [PARKHI2015] Parkhi, O. M., Vedaldi, A., & Zisserman, A. (2015, September). Deep Face Recognition. In BMVC (Vol. 1, No. 3, p. 6).
FAQs
Vision-algorithms Requests Processing Wrappers for deep-learning Computer Vision algorithms on the cloud.
We found that vrpwrp demonstrated a healthy version release cadence and project activity because the last version was released less than a year ago. It has 1 open source maintainer collaborating on the project.
Did you know?
Socket for GitHub automatically highlights issues in each pull request and monitors the health of all your open source dependencies. Discover the contents of your packages and block harmful activity before you install or update your dependencies.
Research
Security News
A malicious npm package targets Solana developers, rerouting funds in 2% of transactions to a hardcoded address.
Security News
Research
Socket researchers have discovered malicious npm packages targeting crypto developers, stealing credentials and wallet data using spyware delivered through typosquats of popular cryptographic libraries.
Security News
Socket's package search now displays weekly downloads for npm packages, helping developers quickly assess popularity and make more informed decisions.