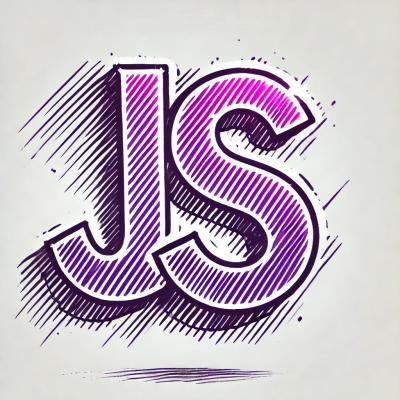
Security News
Oracle Drags Its Feet in the JavaScript Trademark Dispute
Oracle seeks to dismiss fraud claims in the JavaScript trademark dispute, delaying the case and avoiding questions about its right to the name.
AI-based Audio Watermarking Tool
pip install wavmark
The following code adds 16-bit watermark into the input file example.wav
and subsequently performs decoding:
import numpy as np
import soundfile
import torch
import wavmark
# 1.load model
device = torch.device('cuda:0' if torch.cuda.is_available() else 'cpu')
model = wavmark.load_model().to(device)
# 2.create 16-bit payload
payload = np.random.choice([0, 1], size=16)
print("Payload:", payload)
# 3.read host audio
# the audio should be a single-channel 16kHz wav, you can read it using soundfile:
signal, sample_rate = soundfile.read("example.wav")
# Otherwise, you can use the following function to convert the host audio to single-channel 16kHz format:
# from wavmark.utils import file_reader
# signal = file_reader.read_as_single_channel("example.wav", aim_sr=16000)
# 4.encode watermark
watermarked_signal, _ = wavmark.encode_watermark(model, signal, payload, show_progress=True)
# you can save it as a new wav:
# soundfile.write("output.wav", watermarked_signal, 16000)
# 5.decode watermark
payload_decoded, _ = wavmark.decode_watermark(model, watermarked_signal, show_progress=True)
BER = (payload != payload_decoded).mean() * 100
print("Decode BER:%.1f" % BER)
In paper WavMark: Watermarking for Audio Generation we proposed the WavMark model,
which enables encoding 32 bits of information into 1-second audio.
In this tool, we take the first 16 bits as a fixed pattern for watermark identification and the remaining 16 bits as a custom payload.
The same watermark is added repetitively to ensure full-time region protection:
Since the pattern length is 16, the probability of "mistakenly identifying an unwatermarked audio as watermarked" is only 1/(2^16)=0.000015
.
For a specific watermarking algorithm, there exists a trade-off among capacity, robustness, and imperceptibility. Therefore, a watermarking system often needs customization according to application requirements. The good news is that WavMark is entirely implemented with PyTorch. Here is an example of directly calling the PyTorch model:
# 1.load model
device = torch.device('cuda:0' if torch.cuda.is_available() else 'cpu')
model = wavmark.load_model().to(device)
# 2. take 16,000 samples
signal, sample_rate = soundfile.read("example.wav")
trunck = signal[0:16000]
message_npy = np.random.choice([0, 1], size=32)
# 3. do encode:
with torch.no_grad():
signal = torch.FloatTensor(trunck).to(device)[None]
message_tensor = torch.FloatTensor(message_npy).to(device)[None]
signal_wmd_tensor = model.encode(signal, message_tensor)
signal_wmd_npy = signal_wmd_tensor.detach().cpu().numpy().squeeze()
# 4.do decode:
with torch.no_grad():
signal = torch.FloatTensor(signal_wmd_npy).to(device).unsqueeze(0)
message_decoded_npy = (model.decode(signal) >= 0.5).int().detach().cpu().numpy().squeeze()
BER = (message_npy != message_decoded_npy).mean() * 100
print("BER:", BER)
The "Audiowmark" developed by Stefan Westerfeld has provided valuable ideas for the design of this project.
@misc{chen2023wavmark,
title={WavMark: Watermarking for Audio Generation},
author={Guangyu Chen and Yu Wu and Shujie Liu and Tao Liu and Xiaoyong Du and Furu Wei},
year={2023},
eprint={2308.12770},
archivePrefix={arXiv},
primaryClass={cs.SD}
}
FAQs
AI-Based Audio Watermarking Tool
We found that wavmark demonstrated a healthy version release cadence and project activity because the last version was released less than a year ago. It has 1 open source maintainer collaborating on the project.
Did you know?
Socket for GitHub automatically highlights issues in each pull request and monitors the health of all your open source dependencies. Discover the contents of your packages and block harmful activity before you install or update your dependencies.
Security News
Oracle seeks to dismiss fraud claims in the JavaScript trademark dispute, delaying the case and avoiding questions about its right to the name.
Security News
The Linux Foundation is warning open source developers that compliance with global sanctions is mandatory, highlighting legal risks and restrictions on contributions.
Security News
Maven Central now validates Sigstore signatures, making it easier for developers to verify the provenance of Java packages.