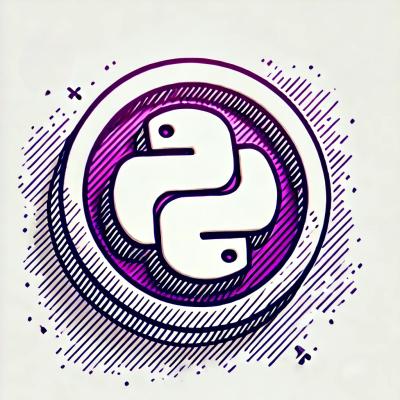
Security News
PyPI Now Supports iOS and Android Wheels for Mobile Python Development
PyPI now supports iOS and Android wheels, making it easier for Python developers to distribute mobile packages.
xverse short for X uniVerse is collection of transformers for feature engineering and feature selection
xverse short for X uniVerse is a Python module for machine learning in the space of feature engineering, feature transformation and feature selection.
Currently, xverse package handles only binary target.
The package requires numpy, pandas, scikit-learn, scipy
and statsmodels
. In addition, the package is tested on Python version 3.5 and above.
To install the package, download this folder and execute:
python setup.py install
or
pip install xverse
To install the development version. you can use
pip install --upgrade git+https://github.com/Sundar0989/XuniVerse
XVerse module is fully compatible with sklearn transformers, so they can be used in pipelines or in your existing scripts. Currently, it supports only Pandas dataframes.
from xverse.transformer import MonotonicBinning
clf = MonotonicBinning()
clf.fit(X, y)
print(clf.bins)
{'age': array([19., 35., 45., 87.]),
'balance': array([-3313. , 174. , 979.33333333, 71188. ]),
'campaign': array([ 1., 3., 50.]),
'day': array([ 1., 12., 20., 31.]),
'duration': array([ 4. , 128. , 261.33333333, 3025. ]),
'pdays': array([-1.00e+00, -5.00e-01, 1.00e+00, 8.71e+02]),
'previous': array([ 0., 1., 25.])}
from xverse.transformer import WOE
clf = WOE()
clf.fit(X, y)
print(clf.woe_df.head()) #Weight of Evidence transformation dataset
+---+---------------+--------------------+-------+-------+-----------+---------------------+--------------------+---------------------+------------------------+----------------------+---------------------+
| | Variable_Name | Category | Count | Event | Non_Event | Event_Rate | Non_Event_Rate | Event_Distribution | Non_Event_Distribution | WOE | Information_Value |
+---+---------------+--------------------+-------+-------+-----------+---------------------+--------------------+---------------------+------------------------+----------------------+---------------------+
| 0 | age | (18.999, 35.0] | 1652 | 197 | 1455 | 0.11924939467312348 | 0.8807506053268765 | 0.3781190019193858 | 0.36375 | 0.038742147481056366 | 0.02469286279236605 |
+---+---------------+--------------------+-------+-------+-----------+---------------------+--------------------+---------------------+------------------------+----------------------+---------------------+
| 1 | age | (35.0, 45.0] | 1388 | 129 | 1259 | 0.09293948126801153 | 0.9070605187319885 | 0.2476007677543186 | 0.31475 | -0.2399610313340142 | 0.02469286279236605 |
+---+---------------+--------------------+-------+-------+-----------+---------------------+--------------------+---------------------+------------------------+----------------------+---------------------+
| 2 | age | (45.0, 87.0] | 1481 | 195 | 1286 | 0.13166779203241052 | 0.8683322079675895 | 0.3742802303262956 | 0.3215 | 0.15200725211484276 | 0.02469286279236605 |
+---+---------------+--------------------+-------+-------+-----------+---------------------+--------------------+---------------------+------------------------+----------------------+---------------------+
| 3 | balance | (-3313.001, 174.0] | 1512 | 133 | 1379 | 0.08796296296296297 | 0.9120370370370371 | 0.255278310940499 | 0.34475 | -0.3004651512228873 | 0.06157421302850976 |
+---+---------------+--------------------+-------+-------+-----------+---------------------+--------------------+---------------------+------------------------+----------------------+---------------------+
| 4 | balance | (174.0, 979.333] | 1502 | 163 | 1339 | 0.1085219707057257 | 0.8914780292942743 | 0.31285988483685223 | 0.33475 | -0.06762854653574929 | 0.06157421302850976 |
+---+---------------+--------------------+-------+-------+-----------+---------------------+--------------------+---------------------+------------------------+----------------------+---------------------+
print(clf.iv_df) #Information value dataset
+----+---------------+------------------------+
| | Variable_Name | Information_Value |
+----+---------------+------------------------+
| 6 | duration | 1.1606798895024775 |
+----+---------------+------------------------+
| 14 | poutcome | 0.4618899274360784 |
+----+---------------+------------------------+
| 12 | month | 0.37953277364723703 |
+----+---------------+------------------------+
| 3 | contact | 0.2477624664660033 |
+----+---------------+------------------------+
| 13 | pdays | 0.20326698063078097 |
+----+---------------+------------------------+
| 15 | previous | 0.1770811514357682 |
+----+---------------+------------------------+
| 9 | job | 0.13251854742728092 |
+----+---------------+------------------------+
| 8 | housing | 0.10655553101753026 |
+----+---------------+------------------------+
| 1 | balance | 0.06157421302850976 |
+----+---------------+------------------------+
| 10 | loan | 0.06079091829519839 |
+----+---------------+------------------------+
| 11 | marital | 0.04009032555607127 |
+----+---------------+------------------------+
| 7 | education | 0.03181211694236827 |
+----+---------------+------------------------+
| 0 | age | 0.02469286279236605 |
+----+---------------+------------------------+
| 2 | campaign | 0.019350877455830695 |
+----+---------------+------------------------+
| 4 | day | 0.0028156288525541884 |
+----+---------------+------------------------+
| 5 | default | 1.6450124824351054e-05 |
+----+---------------+------------------------+
+-------------------+-----------------------------+
| Information Value | Variable Predictiveness |
+-------------------+-----------------------------+
| Less than 0.02 | Not useful for prediction |
+-------------------+-----------------------------+
| 0.02 to 0.1 | Weak predictive Power |
+-------------------+-----------------------------+
| 0.1 to 0.3 | Medium predictive Power |
+-------------------+-----------------------------+
| 0.3 to 0.5 | Strong predictive Power |
+-------------------+-----------------------------+
| >0.5 | Suspicious Predictive Power |
+-------------------+-----------------------------+
clf.transform(X) #apply WOE transformation on the dataset
from xverse.ensemble import VotingSelector
clf = VotingSelector()
clf.fit(X, y)
print(clf.available_techniques)
['WOE', 'RF', 'RFE', 'ETC', 'CS', 'L_ONE']
clf.feature_importances_
+----+---------------+------------------------+-----------------------+-------------------------------+----------------------+----------------------+-------------------------+
| | Variable_Name | Information_Value | Random_Forest | Recursive_Feature_Elimination | Extra_Trees | Chi_Square | L_One |
+----+---------------+------------------------+-----------------------+-------------------------------+----------------------+----------------------+-------------------------+
| 0 | duration | 1.1606798895024775 | 0.29100016518065835 | 0.0 | 0.24336032789230097 | 62.53045588382914 | 0.0009834060765907017 |
+----+---------------+------------------------+-----------------------+-------------------------------+----------------------+----------------------+-------------------------+
| 1 | poutcome | 0.4618899274360784 | 0.05975563617541324 | 0.8149539108454378 | 0.07291945099022576 | 209.1788690088815 | 0.27884071686005385 |
+----+---------------+------------------------+-----------------------+-------------------------------+----------------------+----------------------+-------------------------+
| 2 | month | 0.37953277364723703 | 0.09472524644853274 | 0.6270707318033509 | 0.10303345973615481 | 54.81011477300214 | 0.18763733424335785 |
+----+---------------+------------------------+-----------------------+-------------------------------+----------------------+----------------------+-------------------------+
| 3 | contact | 0.2477624664660033 | 0.018358265986906014 | 0.45594899004325673 | 0.029325952072445132 | 25.357947712611868 | 0.04876094100065351 |
+----+---------------+------------------------+-----------------------+-------------------------------+----------------------+----------------------+-------------------------+
| 4 | pdays | 0.20326698063078097 | 0.04927368012222067 | 0.0 | 0.02738001362078519 | 13.808925800391403 | -0.00026932622581396677 |
+----+---------------+------------------------+-----------------------+-------------------------------+----------------------+----------------------+-------------------------+
| 5 | previous | 0.1770811514357682 | 0.02612886929056733 | 0.0 | 0.027197295919351088 | 13.019278420681164 | 0.0 |
+----+---------------+------------------------+-----------------------+-------------------------------+----------------------+----------------------+-------------------------+
| 6 | job | 0.13251854742728092 | 0.050024353325485646 | 0.5207956132479409 | 0.05775450997836301 | 13.043319831003855 | 0.11279310830899944 |
+----+---------------+------------------------+-----------------------+-------------------------------+----------------------+----------------------+-------------------------+
| 7 | housing | 0.10655553101753026 | 0.021126744587568032 | 0.28135643347861894 | 0.020830177741565564 | 28.043094016887064 | 0.0 |
+----+---------------+------------------------+-----------------------+-------------------------------+----------------------+----------------------+-------------------------+
| 8 | balance | 0.06157421302850976 | 0.0963543249575152 | 0.0 | 0.08429423739161768 | 0.03720300378031974 | -1.3553979494412002e-06 |
+----+---------------+------------------------+-----------------------+-------------------------------+----------------------+----------------------+-------------------------+
| 9 | loan | 0.06079091829519839 | 0.008783347837152861 | 0.6414812505459246 | 0.013652849211750306 | 3.4361027026756084 | 0.0 |
+----+---------------+------------------------+-----------------------+-------------------------------+----------------------+----------------------+-------------------------+
| 10 | marital | 0.04009032555607127 | 0.02648832289940045 | 0.9140684291962617 | 0.03929791951230852 | 10.889749514307464 | 0.0 |
+----+---------------+------------------------+-----------------------+-------------------------------+----------------------+----------------------+-------------------------+
| 11 | education | 0.03181211694236827 | 0.02757205345952717 | 0.21529148795958114 | 0.03980467391633981 | 4.70588768051867 | 0.0 |
+----+---------------+------------------------+-----------------------+-------------------------------+----------------------+----------------------+-------------------------+
| 12 | age | 0.02469286279236605 | 0.10164634631051869 | 0.0 | 0.08893247762137796 | 0.6818947945319156 | -0.004414426121909251 |
+----+---------------+------------------------+-----------------------+-------------------------------+----------------------+----------------------+-------------------------+
| 13 | campaign | 0.019350877455830695 | 0.04289312347011537 | 0.0 | 0.05716486374991612 | 1.8596566731099653 | -0.012650844735972498 |
+----+---------------+------------------------+-----------------------+-------------------------------+----------------------+----------------------+-------------------------+
| 14 | day | 0.0028156288525541884 | 0.083859807784465 | 0.0 | 0.09056623672332145 | 0.08687716739873641 | -0.00231307077371602 |
+----+---------------+------------------------+-----------------------+-------------------------------+----------------------+----------------------+-------------------------+
| 15 | default | 1.6450124824351054e-05 | 0.0020097121639531665 | 0.0 | 0.004485553922176626 | 0.007542737902818529 | 0.0 |
+----+---------------+------------------------+-----------------------+-------------------------------+----------------------+----------------------+-------------------------+
clf.feature_votes_
+----+---------------+-------------------+---------------+-------------------------------+-------------+------------+-------+-------+
| | Variable_Name | Information_Value | Random_Forest | Recursive_Feature_Elimination | Extra_Trees | Chi_Square | L_One | Votes |
+----+---------------+-------------------+---------------+-------------------------------+-------------+------------+-------+-------+
| 1 | poutcome | 1 | 1 | 1 | 1 | 1 | 1 | 6 |
+----+---------------+-------------------+---------------+-------------------------------+-------------+------------+-------+-------+
| 2 | month | 1 | 1 | 1 | 1 | 1 | 1 | 6 |
+----+---------------+-------------------+---------------+-------------------------------+-------------+------------+-------+-------+
| 6 | job | 1 | 1 | 1 | 1 | 1 | 1 | 6 |
+----+---------------+-------------------+---------------+-------------------------------+-------------+------------+-------+-------+
| 0 | duration | 1 | 1 | 0 | 1 | 1 | 1 | 5 |
+----+---------------+-------------------+---------------+-------------------------------+-------------+------------+-------+-------+
| 3 | contact | 1 | 0 | 1 | 0 | 1 | 1 | 4 |
+----+---------------+-------------------+---------------+-------------------------------+-------------+------------+-------+-------+
| 4 | pdays | 1 | 1 | 0 | 0 | 1 | 0 | 3 |
+----+---------------+-------------------+---------------+-------------------------------+-------------+------------+-------+-------+
| 7 | housing | 1 | 0 | 1 | 0 | 1 | 0 | 3 |
+----+---------------+-------------------+---------------+-------------------------------+-------------+------------+-------+-------+
| 12 | age | 0 | 1 | 0 | 1 | 0 | 1 | 3 |
+----+---------------+-------------------+---------------+-------------------------------+-------------+------------+-------+-------+
| 14 | day | 0 | 1 | 0 | 1 | 0 | 1 | 3 |
+----+---------------+-------------------+---------------+-------------------------------+-------------+------------+-------+-------+
| 5 | previous | 1 | 0 | 0 | 0 | 1 | 0 | 2 |
+----+---------------+-------------------+---------------+-------------------------------+-------------+------------+-------+-------+
| 8 | balance | 0 | 1 | 0 | 1 | 0 | 0 | 2 |
+----+---------------+-------------------+---------------+-------------------------------+-------------+------------+-------+-------+
| 13 | campaign | 0 | 0 | 0 | 1 | 0 | 1 | 2 |
+----+---------------+-------------------+---------------+-------------------------------+-------------+------------+-------+-------+
| 9 | loan | 0 | 0 | 1 | 0 | 0 | 0 | 1 |
+----+---------------+-------------------+---------------+-------------------------------+-------------+------------+-------+-------+
| 10 | marital | 0 | 0 | 1 | 0 | 0 | 0 | 1 |
+----+---------------+-------------------+---------------+-------------------------------+-------------+------------+-------+-------+
| 11 | education | 0 | 0 | 1 | 0 | 0 | 0 | 1 |
+----+---------------+-------------------+---------------+-------------------------------+-------------+------------+-------+-------+
| 15 | default | 0 | 0 | 0 | 0 | 0 | 0 | 0 |
+----+---------------+-------------------+---------------+-------------------------------+-------------+------------+-------+-------+
XuniVerse is under active development, if you'd like to be involved, we'd love to have you. Check out the CONTRIBUTING.md file or open an issue on the github project to get started.
https://www.listendata.com/2015/03/weight-of-evidence-woe-and-information.html
https://medium.com/@sundarstyles89/variable-selection-using-python-vote-based-approach-faa42da960f0
Alessio Tamburro (https://github.com/alessiot)
FAQs
xverse short for X uniVerse is collection of transformers for feature engineering and feature selection
We found that xverse demonstrated a healthy version release cadence and project activity because the last version was released less than a year ago. It has 1 open source maintainer collaborating on the project.
Did you know?
Socket for GitHub automatically highlights issues in each pull request and monitors the health of all your open source dependencies. Discover the contents of your packages and block harmful activity before you install or update your dependencies.
Security News
PyPI now supports iOS and Android wheels, making it easier for Python developers to distribute mobile packages.
Security News
Create React App is officially deprecated due to React 19 issues and lack of maintenance—developers should switch to Vite or other modern alternatives.
Security News
Oracle seeks to dismiss fraud claims in the JavaScript trademark dispute, delaying the case and avoiding questions about its right to the name.