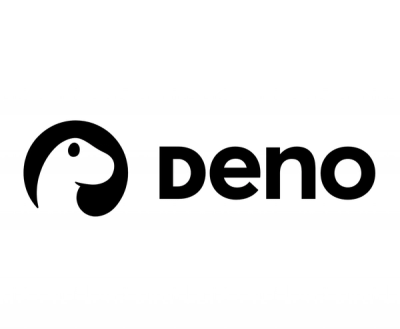
Security News
Deno 2.2 Improves Dependency Management and Expands Node.js Compatibility
Deno 2.2 enhances Node.js compatibility, improves dependency management, adds OpenTelemetry support, and expands linting and task automation for developers.
@google-cloud/vertexai
Advanced tools
The Vertex AI SDK for Node.js lets you use the Vertex AI Gemini API to build AI-powered features and applications. Both TypeScript and JavaScript are supported. The sample code in this document is written in JavaScript.
For detailed samples using the Vertex AI Node.js SDK, see the samples repository on GitHub.
For the latest list of available Gemini models on Vertex AI, see the Model information page in Vertex AI documentation.
Make sure your node.js version is 18 or above.
Create local authentication credentials for your user account:
gcloud auth application-default login
A list of accepted authentication options are listed in GoogleAuthOptions interface of google-auth-library-node.js GitHub repo.
Install the Vertex AI SDK for Node.js by running the following command:
npm install @google-cloud/vertexai
VertexAI
classTo use the Vertex AI SDK for Node.js, create an instance of VertexAI
by
passing it your Google Cloud project ID and location. Then create a reference to
a generative model.
const {
FunctionDeclarationSchemaType,
HarmBlockThreshold,
HarmCategory,
VertexAI
} = require('@google-cloud/vertexai');
const project = 'your-cloud-project';
const location = 'us-central1';
const textModel = 'gemini-1.0-pro';
const visionModel = 'gemini-1.0-pro-vision';
const vertexAI = new VertexAI({project: project, location: location});
// Instantiate Gemini models
const generativeModel = vertexAI.getGenerativeModel({
model: textModel,
// The following parameters are optional
// They can also be passed to individual content generation requests
safetySettings: [{category: HarmCategory.HARM_CATEGORY_DANGEROUS_CONTENT, threshold: HarmBlockThreshold.BLOCK_MEDIUM_AND_ABOVE}],
generationConfig: {maxOutputTokens: 256},
systemInstruction: {
role: 'system',
parts: [{"text": `For example, you are a helpful customer service agent.`}]
},
});
const generativeVisionModel = vertexAI.getGenerativeModel({
model: visionModel,
});
const generativeModelPreview = vertexAI.preview.getGenerativeModel({
model: textModel,
});
You can send text prompt requests by using generateContentStream
for streamed
responses, or generateContent
for nonstreamed responses.
The response is returned in chunks as it's being generated to reduce the perception of latency to a human reader.
async function streamGenerateContent() {
const request = {
contents: [{role: 'user', parts: [{text: 'How are you doing today?'}]}],
};
const streamingResult = await generativeModel.generateContentStream(request);
for await (const item of streamingResult.stream) {
console.log('stream chunk: ', JSON.stringify(item));
}
const aggregatedResponse = await streamingResult.response;
console.log('aggregated response: ', JSON.stringify(aggregatedResponse));
};
streamGenerateContent();
The response is returned all at once.
async function generateContent() {
const request = {
contents: [{role: 'user', parts: [{text: 'How are you doing today?'}]}],
};
const result = await generativeModel.generateContent(request);
const response = result.response;
console.log('Response: ', JSON.stringify(response));
};
generateContent();
Chat requests use previous messages as context when responding to new prompts.
To send multiturn chat requests, use sendMessageStream
for streamed responses,
or sendMessage
for nonstreamed responses.
The response is returned in chunks as it's being generated to reduce the perception of latency to a human reader.
async function streamChat() {
const chat = generativeModel.startChat();
const chatInput = "How can I learn more about Node.js?";
const result = await chat.sendMessageStream(chatInput);
for await (const item of result.stream) {
console.log("Stream chunk: ", item.candidates[0].content.parts[0].text);
}
const aggregatedResponse = await result.response;
console.log('Aggregated response: ', JSON.stringify(aggregatedResponse));
}
streamChat();
The response is returned all at once.
async function sendChat() {
const chat = generativeModel.startChat();
const chatInput = "How can I learn more about Node.js?";
const result = await chat.sendMessage(chatInput);
const response = result.response;
console.log('response: ', JSON.stringify(response));
}
sendChat();
Prompt requests can include either an image or video in addition to text. For more information, see Send multimodal prompt requests in the Vertex AI documentation.
You can include images in the prompt either by specifying the Cloud Storage URI where the image is located or by including a base64 encoding of the image.
You can specify the Cloud Storage URI of the image in fileUri
.
async function multiPartContent() {
const filePart = {fileData: {fileUri: "gs://generativeai-downloads/images/scones.jpg", mimeType: "image/jpeg"}};
const textPart = {text: 'What is this picture about?'};
const request = {
contents: [{role: 'user', parts: [textPart, filePart]}],
};
const streamingResult = await generativeVisionModel.generateContentStream(request);
for await (const item of streamingResult.stream) {
console.log('stream chunk: ', JSON.stringify(item));
}
const aggregatedResponse = await streamingResult.response;
console.log(aggregatedResponse.candidates[0].content);
}
multiPartContent();
You can specify the base64 image encoding string in data
.
async function multiPartContentImageString() {
// Replace this with your own base64 image string
const base64Image = 'iVBORw0KGgoAAAANSUhEUgAAAAEAAAABCAYAAAAfFcSJAAAADUlEQVR42mP8z8BQDwAEhQGAhKmMIQAAAABJRU5ErkJggg==';
const filePart = {inline_data: {data: base64Image, mimeType: 'image/jpeg'}};
const textPart = {text: 'What is this picture about?'};
const request = {
contents: [{role: 'user', parts: [textPart, filePart]}],
};
const streamingResult = await generativeVisionModel.generateContentStream(request);
const contentResponse = await streamingResult.response;
console.log(contentResponse.candidates[0].content.parts[0].text);
}
multiPartContentImageString();
You can include videos in the prompt by specifying the Cloud Storage URI
where the video is located in fileUri
.
async function multiPartContentVideo() {
const filePart = {fileData: {fileUri: 'gs://cloud-samples-data/video/animals.mp4', mimeType: 'video/mp4'}};
const textPart = {text: 'What is in the video?'};
const request = {
contents: [{role: 'user', parts: [textPart, filePart]}],
};
const streamingResult = await generativeVisionModel.generateContentStream(request);
for await (const item of streamingResult.stream) {
console.log('stream chunk: ', JSON.stringify(item));
}
const aggregatedResponse = await streamingResult.response;
console.log(aggregatedResponse.candidates[0].content);
}
multiPartContentVideo();
The Vertex AI SDK for Node.js supports
function calling
in the sendMessage
, sendMessageStream
, generateContent
, and
generateContentStream
methods. We recommend using it through the chat methods
(sendMessage
or sendMessageStream
) but have included examples of both
approaches below.
The following examples show you how to declare a function.
const functionDeclarations = [
{
functionDeclarations: [
{
name: "get_current_weather",
description: 'get weather in a given location',
parameters: {
type: FunctionDeclarationSchemaType.OBJECT,
properties: {
location: {type: FunctionDeclarationSchemaType.STRING},
unit: {
type: FunctionDeclarationSchemaType.STRING,
enum: ['celsius', 'fahrenheit'],
},
},
required: ['location'],
},
},
],
},
];
const functionResponseParts = [
{
functionResponse: {
name: "get_current_weather",
response:
{name: "get_current_weather", content: {weather: "super nice"}},
},
},
];
sendMessageStream
After the function is declared, you can pass it to the model in the
tools
parameter of the prompt request.
async function functionCallingChat() {
// Create a chat session and pass your function declarations
const chat = generativeModel.startChat({
tools: functionDeclarations,
});
const chatInput1 = 'What is the weather in Boston?';
// This should include a functionCall response from the model
const streamingResult1 = await chat.sendMessageStream(chatInput1);
for await (const item of streamingResult1.stream) {
console.log(item.candidates[0]);
}
const response1 = await streamingResult1.response;
console.log("first aggregated response: ", JSON.stringify(response1));
// Send a follow up message with a FunctionResponse
const streamingResult2 = await chat.sendMessageStream(functionResponseParts);
for await (const item of streamingResult2.stream) {
console.log(item.candidates[0]);
}
// This should include a text response from the model using the response content
// provided above
const response2 = await streamingResult2.response;
console.log("second aggregated response: ", JSON.stringify(response2));
}
functionCallingChat();
generateContentStream
async function functionCallingGenerateContentStream() {
const request = {
contents: [
{role: 'user', parts: [{text: 'What is the weather in Boston?'}]},
{role: 'model', parts: [{functionCall: {name: 'get_current_weather', args: {'location': 'Boston'}}}]},
{role: 'user', parts: functionResponseParts}
],
tools: functionDeclarations,
};
const streamingResult =
await generativeModel.generateContentStream(request);
for await (const item of streamingResult.stream) {
console.log(item.candidates[0]);
}
}
functionCallingGenerateContentStream();
async function countTokens() {
const request = {
contents: [{role: 'user', parts: [{text: 'How are you doing today?'}]}],
};
const response = await generativeModel.countTokens(request);
console.log('count tokens response: ', JSON.stringify(response));
}
countTokens();
Grounding is preview only feature.
Grounding lets you connect model output to verifiable sources of information to reduce hallucination. You can specify Google Search or Vertex AI search as the data source for grounding.
async function generateContentWithGoogleSearchGrounding() {
const generativeModelPreview = vertexAI.preview.getGenerativeModel({
model: textModel,
// The following parameters are optional
// They can also be passed to individual content generation requests
safetySettings: [{category: HarmCategory.HARM_CATEGORY_DANGEROUS_CONTENT, threshold: HarmBlockThreshold.BLOCK_MEDIUM_AND_ABOVE}],
generationConfig: {maxOutputTokens: 256},
});
const googleSearchRetrievalTool = {
googleSearchRetrieval: {
disableAttribution: false,
},
};
const result = await generativeModelPreview.generateContent({
contents: [{role: 'user', parts: [{text: 'Why is the sky blue?'}]}],
tools: [googleSearchRetrievalTool],
})
const response = result.response;
const groundingMetadata = response.candidates[0].groundingMetadata;
console.log("GroundingMetadata is: ", JSON.stringify(groundingMetadata));
}
generateContentWithGoogleSearchGrounding();
async function generateContentWithVertexAISearchGrounding() {
const generativeModelPreview = vertexAI.preview.getGenerativeModel({
model: textModel,
// The following parameters are optional
// They can also be passed to individual content generation requests
safetySettings: [{category: HarmCategory.HARM_CATEGORY_DANGEROUS_CONTENT, threshold: HarmBlockThreshold.BLOCK_MEDIUM_AND_ABOVE}],
generationConfig: {maxOutputTokens: 256},
});
const vertexAIRetrievalTool = {
retrieval: {
vertexAiSearch: {
datastore: 'projects/.../locations/.../collections/.../dataStores/...',
},
disableAttribution: false,
},
};
const result = await generativeModelPreview.generateContent({
contents: [{role: 'user', parts: [{text: 'Why is the sky blue?'}]}],
tools: [vertexAIRetrievalTool],
})
const response = result.response;
const groundingMetadata = response.candidates[0].groundingMetadata;
console.log("Grounding metadata is: ", JSON.stringify(groundingMetadata));
}
generateContentWithVertexAISearchGrounding();
You can include an optional system instruction when instantiating a generative model to provide additional context to the model.
The system instruction can also be passed to individual text prompt requests.
const generativeModel = vertexAI.getGenerativeModel({
model: textModel,
// The following parameter is optional.
systemInstruction: {
role: 'system',
parts: [{"text": `For example, you are a helpful customer service agent.`}]
},
});
async function generateContent() {
const request = {
contents: [{role: 'user', parts: [{text: 'How are you doing today?'}]}],
systemInstruction: { role: 'system', parts: [{ text: `For example, you are a helpful customer service agent.` }] },
};
const result = await generativeModel.generateContent(request);
const response = result.response;
console.log('Response: ', JSON.stringify(response));
};
generateContent();
Step1: Find a list of accepted authentication options in GoogleAuthOptions interface of google-auth-library-node.js GitHub repo.
Step2: Instantiate the VertexAI
class by passing in the GoogleAuthOptions
interface as follows:
const { VertexAI } = require('@google-cloud/vertexai');
const { GoogleAuthOptions } = require('google-auth-library');
const vertexAI = new VertexAI(
{
googleAuthOptions: {
// your GoogleAuthOptions interface
}
}
)
The contents of this repository are licensed under the Apache License, version 2.0.
FAQs
Vertex Generative AI client for Node.js
The npm package @google-cloud/vertexai receives a total of 164,877 weekly downloads. As such, @google-cloud/vertexai popularity was classified as popular.
We found that @google-cloud/vertexai demonstrated a healthy version release cadence and project activity because the last version was released less than a year ago. It has 2 open source maintainers collaborating on the project.
Did you know?
Socket for GitHub automatically highlights issues in each pull request and monitors the health of all your open source dependencies. Discover the contents of your packages and block harmful activity before you install or update your dependencies.
Security News
Deno 2.2 enhances Node.js compatibility, improves dependency management, adds OpenTelemetry support, and expands linting and task automation for developers.
Security News
React's CRA deprecation announcement sparked community criticism over framework recommendations, leading to quick updates acknowledging build tools like Vite as valid alternatives.
Security News
Ransomware payment rates hit an all-time low in 2024 as law enforcement crackdowns, stronger defenses, and shifting policies make attacks riskier and less profitable.