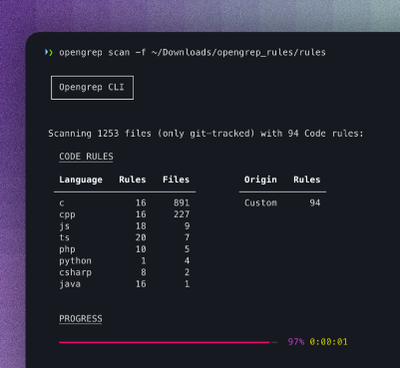
Security News
Opengrep Emerges as Open Source Alternative Amid Semgrep Licensing Controversy
Opengrep forks Semgrep to preserve open source SAST in response to controversial licensing changes.
@tensorflow/tfjs-layers
Advanced tools
A part of the TensorFlow.js ecosystem, TensorFlow.js Layers is a high-level API built on TensorFlow.js Core, enabling users to build, train and execute deep learning models in the browser. TensorFlow.js Layers is modeled after Keras and tf.keras and can load models saved from those libraries.
There are three ways to import TensorFlow.js Layers
tfjs-layers
has peer dependency on tfjs-core, so if you import
@tensorflow/tfjs-layers
, you also need to import
@tensorflow/tfjs-core
.Option 1 is the most convenient, but leads to a larger bundle size (we will be adding more packages to it in the future). Use option 2 if you care about bundle size.
The following example shows how to build a toy model with only one dense
layer
to perform linear regression.
import * as tf from '@tensorflow/tfjs';
// A sequential model is a container which you can add layers to.
const model = tf.sequential();
// Add a dense layer with 1 output unit.
model.add(tf.layers.dense({units: 1, inputShape: [1]}));
// Specify the loss type and optimizer for training.
model.compile({loss: 'meanSquaredError', optimizer: 'SGD'});
// Generate some synthetic data for training.
const xs = tf.tensor2d([[1], [2], [3], [4]], [4, 1]);
const ys = tf.tensor2d([[1], [3], [5], [7]], [4, 1]);
// Train the model.
await model.fit(xs, ys, {epochs: 500});
// Ater the training, perform inference.
const output = model.predict(tf.tensor2d([[5]], [1, 1]));
output.print();
You can also load a model previously trained and saved from elsewhere (e.g., from Python Keras) and use it for inference or transfer learning in the browser.
For example, in Python, save your Keras model using
tensorflowjs,
which can be installed using pip install tensorflowjs
.
import tensorflowjs as tfjs
# ... Create and train your Keras model.
# Save your Keras model in TensorFlow.js format.
tfjs.converters.save_keras_model(model, '/path/to/tfjs_artifacts/')
# Then use your favorite web server to serve the directory at a URL, say
# http://foo.bar/tfjs_artifacts/model.json
To load the model with TensorFlow.js Layers:
import * as tf from '@tensorflow/tfjs';
const model = await tf.loadLayersModel('http://foo.bar/tfjs_artifacts/model.json');
// Now the model is ready for inference, evaluation or re-training.
FAQs
TensorFlow layers API in JavaScript
The npm package @tensorflow/tfjs-layers receives a total of 78,466 weekly downloads. As such, @tensorflow/tfjs-layers popularity was classified as popular.
We found that @tensorflow/tfjs-layers demonstrated a healthy version release cadence and project activity because the last version was released less than a year ago. It has 10 open source maintainers collaborating on the project.
Did you know?
Socket for GitHub automatically highlights issues in each pull request and monitors the health of all your open source dependencies. Discover the contents of your packages and block harmful activity before you install or update your dependencies.
Security News
Opengrep forks Semgrep to preserve open source SAST in response to controversial licensing changes.
Security News
Critics call the Node.js EOL CVE a misuse of the system, sparking debate over CVE standards and the growing noise in vulnerability databases.
Security News
cURL and Go security teams are publicly rejecting CVSS as flawed for assessing vulnerabilities and are calling for more accurate, context-aware approaches.