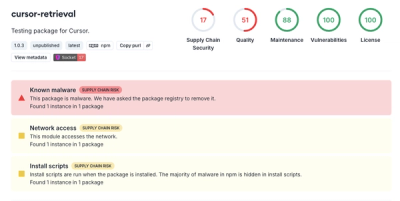
Security News
The Risks of Misguided Research in Supply Chain Security
Snyk's use of malicious npm packages for research raises ethical concerns, highlighting risks in public deployment, data exfiltration, and unauthorized testing.
@tensorscript/ts-deeplearning
Advanced tools
Deep Learning Classification, Clustering and Regression with Tensorflow
Deep Learning Classification and Regression with Tensorflow (Clustering coming soon)
$ npm i @tensorscript/ts-deeplearning
Test against the Iris Flower Data Set
import { DeepLearningClassification, } from '@tensorscript/ts-deeplearning';
import ms from 'modelscript';
async function main(){
const irisFlowerDataCSV = await ms.csv.loadCSV('./test/mock/data/iris_data.csv');
const DataSet = new ms.DataSet(irisFlowerDataCSV);
/**
* encodedData = [
* { sepal_length_cm: 5.1,
sepal_width_cm: 3.5,
petal_length_cm: 1.4,
petal_width_cm: 0.2,
plant: 'Iris-setosa',
'plant_Iris-setosa': 1,
'plant_Iris-versicolor': 0,
'plant_Iris-virginica': 0 },
...
{ sepal_length_cm: 5.9,
sepal_width_cm: 3,
petal_length_cm: 4.2,
petal_width_cm: 1.5,
plant: 'Iris-versicolor',
'plant_Iris-setosa': 0,
'plant_Iris-versicolor': 1,
'plant_Iris-virginica': 0 },
];
*/
const encodedData = DataSet.fitColumns({
columns: [
{
name: 'plant',
options: {
strategy: 'onehot',
},
},
],
returnData:true,
});
const independentVariables = [
'sepal_length_cm',
'sepal_width_cm',
'petal_length_cm',
'petal_width_cm',
];
const dependentVariables = [
'plant_Iris-setosa',
'plant_Iris-versicolor',
'plant_Iris-virginica',
];
const x_matrix = DataSet.columnMatrix(independentVariables);
const y_matrix = DataSet.columnMatrix(dependentVariables);
/*
x_matrix = [
[ 5.1, 3.5, 1.4, 0.2 ],
[ 4.9, 3, 1.4, 0.2 ],
[ 4.7, 3.2, 1.3, 0.2 ],
...
];
y_matrix = [
[ 1, 0, 0 ],
[ 1, 0, 0 ],
[ 1, 0, 0 ],
...
]
*/
const input_x = [
[5.1, 3.5, 1.4, 0.2, ],
[6.3, 3.3, 6.0, 2.5, ],
[5.6, 3.0, 4.5, 1.5, ],
[5.0, 3.2, 1.2, 0.2, ],
[4.5, 2.3, 1.3, 0.3, ],
];
const nnClassification = new DeepLearningClassification();
const nnClassificationModel = await nnClassification.train(x_matrix, y_matrix);
const predictions = await nnClassification.predict(input_x);
const answers = await nnClassification.predict(input_x, {
probability:false,
});
/*
predictions = [
[ 0.989512026309967, 0.010471616871654987, 0.00001649192017794121, ],
[ 0.0000016141033256644732, 0.054614484310150146, 0.9453839063644409, ],
[ 0.001930746017023921, 0.6456733345985413, 0.3523959517478943, ],
[ 0.9875779747962952, 0.01239941269159317, 0.00002274810685776174, ],
[ 0.9545140862464905, 0.04520365223288536, 0.0002823179238475859, ],
];
answers = [
[ 1, 0, 0, ],
[ 0, 0, 1, ],
[ 0, 1, 0, ],
[ 1, 0, 0, ],
[ 1, 0, 0, ],
];
*/
}
main();
Test against the Boston Housing Data Set
import { DeepLearningRegression, } from '@tensorscript/ts-deeplearning';
import ms from 'modelscript';
function scaleColumnMap(columnName) {
return {
name: columnName,
options: {
strategy: 'scale',
scaleOptions: {
strategy:'standard'
}
}
}
}
async function main(){
const housingdataCSV = await ms.csv.loadCSV('./test/mock/data/boston_housing_data.csv');
/*
housingdataCSV = [
{ CRIM: 0.00632, ZN: 18, INDUS: 2.31, CHAS: 0, NOX: 0.538, RM: 6.575, AGE: 65.2, DIS: 4.09, RAD: 1, TAX: 296, PTRATIO: 15.3, B: 396.9, LSTAT: 4.98, MEDV: 24 },
{ CRIM: 0.02731, ZN: 0, INDUS: 7.07, CHAS: 0, NOX: 0.469, RM: 6.421, AGE: 78.9, DIS: 4.9671, RAD: 2, TAX: 242, PTRATIO: 17.8, B: 396.9, LSTAT: 9.14, MEDV: 21.6 },
...
]
*/
const DataSet = new ms.DataSet(housingdataCSV);
const independentVariables = [
'CRIM',
'ZN',
'INDUS',
'CHAS',
'NOX',
'RM',
'AGE',
'DIS',
'RAD',
'TAX',
'PTRATIO',
'B',
'LSTAT',
];
const dependentVariables = [
'MEDV',
];
const columns = independentVariables.concat(dependentVariables);
DataSet.fitColumns({
columns: columns.map(scaleColumnMap),
returnData:false,
});
const x_matrix = DataSet.columnMatrix(independentVariables);
const y_matrix = DataSet.columnMatrix(dependentVariables);
/* x_matrix = [
[ -0.41936692921321594, 0.2845482693404666, -1.2866362317172035, -0.272329067679207, -0.1440748547324509, 0.4132629204530747, -0.119894767215809, 0.1400749839795629, -0.981871187861867, -0.6659491794887338, -1.457557967289609, 0.4406158949991029, -1.074498970343932 ],
[ -0.41692666996409716, -0.4872401872268264, -0.5927943782429392, -0.272329067679207, -0.7395303607434242, 0.1940823874370036, 0.3668034264326209, 0.5566090495704026, -0.8670244885881488, -0.9863533804386945, -0.3027944997494681, 0.4406158949991029, -0.49195252491856634 ]
...
];
y_matrix = [
[ 0.15952778852449556 ],
[ -0.1014239172731213 ],
...
];
const y_vector = ms.util.pivotVector(y_matrix)[ 0 ];// not used but just illustrative
y_vector = [ 0.15952778852449556, -0.1014239172731213, ... ]
*/
const input_x = [
[-0.41936692921321594, 0.2845482693404666, -1.2866362317172035, -0.272329067679207, -0.1440748547324509, 0.4132629204530747, -0.119894767215809, 0.1400749839795629, -0.981871187861867, -0.6659491794887338, -1.457557967289609, 0.4406158949991029, -1.074498970343932,],
[-0.41692666996409716, -0.4872401872268264, -0.5927943782429392, -0.272329067679207, -0.7395303607434242, 0.1940823874370036, 0.3668034264326209, 0.5566090495704026, -0.8670244885881488, -0.9863533804386945, -0.3027944997494681, 0.4406158949991029, -0.49195252491856634,],
];
const nnRegression = new DeepLearningRegression();
const model = await nnRegression.train(x_matrix, y_matrix);
const predictions = await nnRegressionWide.predict(input_x); // [ [ 0.43396109342575073 ], [ 0.12437985092401505 ] ]
const predictions_unscaled = predictions.map(pred=>DataSet.scalers.get('MEDV').descale(pred[0])); //[ 26.523991670220486, 23.67674075943165 ]
}
main();
Test against the Portland housing price dataset
import { MultipleLinearRegression, } from '@tensorscript/ts-deeplearning';
import ms from 'modelscript';
function scaleColumnMap(columnName) {
return {
name: columnName,
options: {
strategy: 'scale',
scaleOptions: {
strategy:'standard'
}
}
}
}
async function main(){
const housingdataCSV = await ms.csv.loadCSV('./test/mock/data/portland_housing_data.csv');
/*
housingdataCSV = [
{ sqft: 2104, bedrooms: 3, price: 399900 },
{ sqft: 1600, bedrooms: 3, price: 329900 },
...
{ sqft: 1203, bedrooms: 3, price: 239500 }
]
*/
const DataSet = new ms.DataSet(housingdataCSV);
DataSet.fitColumns({
columns: [
'sqft',
'bedrooms',
'price',
].map(scaleColumnMap),
returnData:true,
});
const independentVariables = [ 'sqft', 'bedrooms',];
const dependentVariables = [ 'price', ];
const x_matrix = DataSet.columnMatrix(independentVariables);
const y_matrix = DataSet.columnMatrix(dependentVariables);
/* x_matrix = [
[2014, 3],
[1600, 3],
];
y_matrix = [
[399900],
[329900],
];
const y_vector = ms.util.pivotVector(y_matrix)[ 0 ];// not used but just illustrative
// y_vector = [ 399900, 329900]
*/
const testSqft = DataSet.scalers.get('sqft').scale(1650);
const testBedrooms = DataSet.scalers.get('bedrooms').scale(3);
const input_x = [
testSqft,
testBedrooms,
]; // input_x: [ -0.4412732005944351, -0.2236751871685913 ]
const tfMLR = new MultipleLinearRegression();
const model = await tfMLR.train(x_matrix, y_matrix);
const scaledPrediction = await tfMLR.predict(input_x); // [ -0.3785287367962629 ]
const prediction = DataSet.scalers.get('price').descale(scaledPrediction); // prediction: 293081.4643348962
}
main();
Test against the Social Media Ads
import { LogisticRegression, } from '@tensorscript/ts-deeplearning';
import ms from 'modelscript';
function scaleColumnMap(columnName) {
return {
name: columnName,
options: {
strategy: 'scale',
scaleOptions: {
strategy:'standard'
}
}
}
}
async function main(){
const CSVData = await ms.csv.loadCSV('./test/mock/data/social_network_ads.csv');
const DataSet = new ms.DataSet(CSVData);
const scaledData = DataSet.fitColumns({
columns: independentVariables.map(scaleColumnMap),
returnData:true,
});
/*
scaledData = [
{ 'User ID': 15624510,
Gender: 'Male',
Age: -1.7795687879022388,
EstimatedSalary: -1.4881825118632386,
Purchased: 0 },
{ 'User ID': 15810944,
Gender: 'Male',
Age: -0.253270175924977,
EstimatedSalary: -1.458854384319991,
Purchased: 0 },
...
];
*/
const independentVariables = [
'Age',
'EstimatedSalary',
];
const dependentVariables = [
'Purchased',
];
const x_matrix = DataSet.columnMatrix(independentVariables);
const y_matrix = DataSet.columnMatrix(dependentVariables);
/*
x_matrix = [
[ -1.7795687879022388, -1.4881825118632386 ],
[ -0.253270175924977, -1.458854384319991 ],
...
];
y_matrix = [
[ 0 ],
[ 0 ],
...
];
*/
const input_x = [
[-0.062482849427819266, 0.30083326827486173,], //0
[0.7960601198093905, -1.1069168538010206,], //1
[0.7960601198093905, 0.12486450301537644,], //0
[0.4144854668150751, -0.49102617539282206,], //0
[0.3190918035664962, 0.5061301610775946,], //1
];
const tfLR = new LogisticRegression();
const model = await tfLR.train(x_matrix, y_matrix);
const prediction = await tfLR.predict(input_x); // => [ [ 0 ], [ 0 ], [ 1 ], [ 0 ], [ 1 ] ],
}
main();
$ npm i
$ npm test
Fork, write tests and create a pull request!
As of Node 8, ES modules are still used behind a flag, when running natively as an ES module
$ node --experimental-modules my-machine-learning-script.mjs
# Also there are native bindings that require Python 2.x, make sure if you're using Andaconda, you build with your Python 2.x bin
$ npm i --python=/usr/bin/python
MIT
FAQs
Deep Learning Classification, Clustering and Regression with Tensorflow
We found that @tensorscript/ts-deeplearning demonstrated a not healthy version release cadence and project activity because the last version was released a year ago. It has 3 open source maintainers collaborating on the project.
Did you know?
Socket for GitHub automatically highlights issues in each pull request and monitors the health of all your open source dependencies. Discover the contents of your packages and block harmful activity before you install or update your dependencies.
Security News
Snyk's use of malicious npm packages for research raises ethical concerns, highlighting risks in public deployment, data exfiltration, and unauthorized testing.
Research
Security News
Socket researchers found several malicious npm packages typosquatting Chalk and Chokidar, targeting Node.js developers with kill switches and data theft.
Security News
pnpm 10 blocks lifecycle scripts by default to improve security, addressing supply chain attack risks but sparking debate over compatibility and workflow changes.