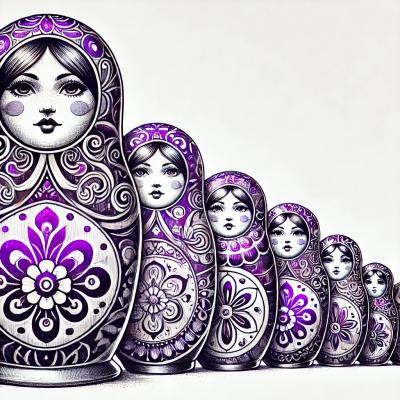
Research
Security News
Threat Actor Exposes Playbook for Exploiting npm to Build Blockchain-Powered Botnets
A threat actor's playbook for exploiting the npm ecosystem was exposed on the dark web, detailing how to build a blockchain-powered botnet.
@xenova/transformers
Advanced tools
State-of-the-art Machine Learning for the web. Run 🤗 Transformers directly in your browser, with no need for a server!
State-of-the-art Machine Learning for the web. Run 🤗 Transformers directly in your browser, with no need for a server!
Transformers.js is designed to be functionally equivalent to Hugging Face's transformers python library, meaning you can run the same pretrained models using a very similar API. These models support common tasks in different modalities, such as:
Transformers.js uses ONNX Runtime to run models in the browser. The best part about it, is that you can easily convert your pretrained PyTorch, TensorFlow, or JAX models to ONNX using 🤗 Optimum.
For more information, check out the full documentation.
It's super simple to translate from existing code! Just like the python library, we support the pipeline
API. Pipelines group together a pretrained model with preprocessing of inputs and postprocessing of outputs, making it the easiest way to run models with the library.
Python (original) | Javascript (ours) |
---|---|
|
|
You can also use a different model by specifying the model id or path as the second argument to the pipeline
function. For example:
// Use a different model for sentiment-analysis
let pipe = await pipeline('sentiment-analysis', 'nlptown/bert-base-multilingual-uncased-sentiment');
To install via NPM, run:
npm i @xenova/transformers
Alternatively, you can use it in vanilla JS, without any bundler, by using a CDN or static hosting. For example, using ES Modules, you can import the library with:
<script type="module">
import { pipeline } from 'https://cdn.jsdelivr.net/npm/@xenova/transformers@2.6.1';
</script>
Want to jump straight in? Get started with one of our sample applications/templates:
Name | Description | Source code |
---|---|---|
Whisper Web | Speech recognition w/ Whisper | link |
Doodle Dash | Real-time sketch-recognition game (see blog) | link |
Code Playground | In-browser code completion website | link |
Semantic Image Search | Search for images with text (Next.js + Supabase) | link |
Vanilla JavaScript | In-browser object detection | link |
React | Multilingual translation website | link |
Browser extension | Text classification extension | link |
Electron | Text classification application | link |
Next.js (client-side) | Sentiment analysis (in-browser inference) | link |
Next.js (server-side) | Sentiment analysis (Node.js inference) | link |
Node.js | Sentiment analysis API | link |
By default, Transformers.js uses hosted pretrained models and precompiled WASM binaries, which should work out-of-the-box. You can customize this as follows:
import { env } from '@xenova/transformers';
// Specify a custom location for models (defaults to '/models/').
env.localModelPath = '/path/to/models/';
// Disable the loading of remote models from the Hugging Face Hub:
env.allowRemoteModels = false;
// Set location of .wasm files. Defaults to use a CDN.
env.backends.onnx.wasm.wasmPaths = '/path/to/files/';
For a full list of available settings, check out the API Reference.
We recommend using our conversion script to convert your PyTorch, TensorFlow, or JAX models to ONNX in a single command. Behind the scenes, it uses 🤗 Optimum to perform conversion and quantization of your model.
python -m scripts.convert --quantize --model_id <model_name_or_path>
For example, convert and quantize bert-base-uncased using:
python -m scripts.convert --quantize --model_id bert-base-uncased
This will save the following files to ./models/
:
bert-base-uncased/
├── config.json
├── tokenizer.json
├── tokenizer_config.json
└── onnx/
├── model.onnx
└── model_quantized.onnx
Here is the list of all tasks and architectures currently supported by Transformers.js. If you don't see your task/model listed here or it is not yet supported, feel free to open up a feature request here.
To find compatible models on the Hub, select the "transformers.js" library tag in the filter menu (or visit this link). You can refine your search by selecting the task you're interested in (e.g., text-classification).
Task | ID | Description | Supported? |
---|---|---|---|
Conversational | conversational | Generating conversational text that is relevant, coherent and knowledgable given a prompt. | ❌ |
Fill-Mask | fill-mask | Masking some of the words in a sentence and predicting which words should replace those masks. | ✅ (docs) (models) |
Question Answering | question-answering | Retrieve the answer to a question from a given text. | ✅ (docs) (models) |
Sentence Similarity | sentence-similarity | Determining how similar two texts are. | ✅ (docs) (models) |
Summarization | summarization | Producing a shorter version of a document while preserving its important information. | ✅ (docs) (models) |
Table Question Answering | table-question-answering | Answering a question about information from a given table. | ❌ |
Text Classification | text-classification or sentiment-analysis | Assigning a label or class to a given text. | ✅ (docs) (models) |
Text Generation | text-generation | Producing new text by predicting the next word in a sequence. | ✅ (docs) (models) |
Text-to-text Generation | text2text-generation | Converting one text sequence into another text sequence. | ✅ (docs) (models) |
Token Classification | token-classification or ner | Assigning a label to each token in a text. | ✅ (docs) (models) |
Translation | translation | Converting text from one language to another. | ✅ (docs) (models) |
Zero-Shot Classification | zero-shot-classification | Classifying text into classes that are unseen during training. | ✅ (docs) (models) |
Task | ID | Description | Supported? |
---|---|---|---|
Depth Estimation | depth-estimation | Predicting the depth of objects present in an image. | ❌ |
Image Classification | image-classification | Assigning a label or class to an entire image. | ✅ (docs) (models) |
Image Segmentation | image-segmentation | Divides an image into segments where each pixel is mapped to an object. This task has multiple variants such as instance segmentation, panoptic segmentation and semantic segmentation. | ✅ (docs) (models) |
Image-to-Image | image-to-image | Transforming a source image to match the characteristics of a target image or a target image domain. | ❌ |
Mask Generation | mask-generation | Generate masks for the objects in an image. | ❌ |
Object Detection | object-detection | Identify objects of certain defined classes within an image. | ✅ (docs) (models) |
Video Classification | n/a | Assigning a label or class to an entire video. | ❌ |
Unconditional Image Generation | n/a | Generating images with no condition in any context (like a prompt text or another image). | ❌ |
Task | ID | Description | Supported? |
---|---|---|---|
Audio Classification | audio-classification | Assigning a label or class to a given audio. | ✅ (docs) (models) |
Audio-to-Audio | n/a | Generating audio from an input audio source. | ❌ |
Automatic Speech Recognition | automatic-speech-recognition | Transcribing a given audio into text. | ✅ (docs) (models) |
Text-to-Speech | n/a | Generating natural-sounding speech given text input. | ❌ |
Task | ID | Description | Supported? |
---|---|---|---|
Tabular Classification | n/a | Classifying a target category (a group) based on set of attributes. | ❌ |
Tabular Regression | n/a | Predicting a numerical value given a set of attributes. | ❌ |
Task | ID | Description | Supported? |
---|---|---|---|
Document Question Answering | document-question-answering | Answering questions on document images. | ❌ |
Feature Extraction | feature-extraction | Transforming raw data into numerical features that can be processed while preserving the information in the original dataset. | ✅ (docs) (models) |
Image-to-Text | image-to-text | Output text from a given image. | ✅ (docs) (models) |
Text-to-Image | text-to-image | Generates images from input text. | ❌ |
Visual Question Answering | visual-question-answering | Answering open-ended questions based on an image. | ❌ |
Zero-Shot Image Classification | zero-shot-image-classification | Classifying images into classes that are unseen during training. | ✅ (docs) (models) |
Task | ID | Description | Supported? |
---|---|---|---|
Reinforcement Learning | n/a | Learning from actions by interacting with an environment through trial and error and receiving rewards (negative or positive) as feedback. | ❌ |
FAQs
State-of-the-art Machine Learning for the web. Run 🤗 Transformers directly in your browser, with no need for a server!
The npm package @xenova/transformers receives a total of 50,737 weekly downloads. As such, @xenova/transformers popularity was classified as popular.
We found that @xenova/transformers demonstrated a healthy version release cadence and project activity because the last version was released less than a year ago. It has 1 open source maintainer collaborating on the project.
Did you know?
Socket for GitHub automatically highlights issues in each pull request and monitors the health of all your open source dependencies. Discover the contents of your packages and block harmful activity before you install or update your dependencies.
Research
Security News
A threat actor's playbook for exploiting the npm ecosystem was exposed on the dark web, detailing how to build a blockchain-powered botnet.
Security News
NVD’s backlog surpasses 20,000 CVEs as analysis slows and NIST announces new system updates to address ongoing delays.
Security News
Research
A malicious npm package disguised as a WhatsApp client is exploiting authentication flows with a remote kill switch to exfiltrate data and destroy files.