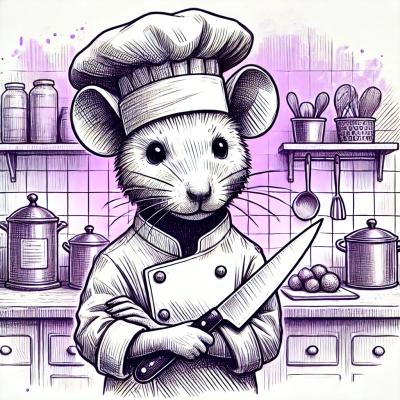
Research
Security News
Quasar RAT Disguised as an npm Package for Detecting Vulnerabilities in Ethereum Smart Contracts
Socket researchers uncover a malicious npm package posing as a tool for detecting vulnerabilities in Etherium smart contracts.
A set of data mining tool for javascript
npm install dmjs
bower install dmjs
Example:
var hc_cluster = new dm.HierachicalCluster()
.data([{
name : '1',
value : {
point : [0, 1]
}
},
{
name : '2',
value: {
point : [0, 2]
}
},
{
name : '3',
value: {
point : [0, 3]
}
},
{
name : '4',
value : {
point : [0, 3]
}
},
{
name : '5',
value : {
point : [0, -1]
}
},
{
name : '6',
value : {
point : [0, 10]
}
},
{
name : '7',
value : {
point : [0, 11]
}
}])
.dist_metric(dm.euclidean_distance)
.dist_fun('max')
.save_history(true)
.init()
.cluster();
console.log('root', hc_cluster.root());
Example:
var km_cluster = new dm.KMean()
.data([{
name : '1',
value : {
point : [1, 1]
}
},
{
name : '2',
value: {
point : [1.5, 2.0]
}
},
{
name : '3',
value: {
point : [3, 4]
}
},
{
name : '4',
value : {
point : [5, 7]
}
},
{
name : '5',
value : {
point : [3.5, 5]
}
},
{
name : '6',
value : {
point : [4.5, 5]
}
},
{
name : '7',
value : {
point : [3.5, 4.5]
}
}
])
.clusters([
{
'name' : 'C1',
'value' : {
'centroid' : [1, 1]
}
},
{
'name' : 'C2',
'value' : {
'centroid' : [5, 7]
}
}
])
.evaluate_sse(true)
.save_history(true)
.stopThreshold(0)
.accessor(function(d){return d.value.point;})
.centroid_fun('mean')
.numIteration(4)
.dist_metric(dm.euclidean_distance)
.cluster();
Example:
//create some points
var points = dm.array2points([
[0.4, 0.53],
[0.22, 0.38],
[0.35, 0.32],
[0.26, 0.19],
[0.08, 0.41],
[0.45, 0.30]
]);
//perform hierachical clustering
var cluster = new dm.HierachicalCluster()
.data(points)
.dist_metric(dm.euclidean_distance)
.dist_fun('centroid')
.save_history(true)
.init()
.cluster();
//Cut the hierachical clustering to 3 clusters
var clustering = cluster.cut_opt('K').cut(3);
//creating the clustering evaluation object
var cev = new dm.ClusterEvaluation().data(clustering);
var wss = cev.WSS();
var bss = cev.BSS();
var tss = cev.TSS();
var silhouette = cev
.silhouette_dist_metric(dm.euclidean_distance)
.silhouette_coefficient();
Example:
var v1 = new dm.SparseVector([0, 1, 5, 6, 10], [1, 1, 1, 1, 1]);
var v2 = new dm.SparseVector([0, 5, 10, 11], [1, 1, 1, 1]);
var v3 = new dm.SparseVector([1], [1]);
var d = v1.dotp(v2);
var s = v1.sum(v2);
console.log('d', d);
console.log('L2', v1.L2norm());
console.log('s', s);
var ind1 = v1.locationAtIndex(5);
console.log('index1', ind1);
var ind3 = v3.locationAtIndex(0);
console.log('index3', ind3);
console.log('v1', v1.toDenseVector());
console.log('v2', v2.toDenseVector());
console.log('v3', v3.toDenseVector());
console.log('s', s.toDenseVector());
v1.setValue(1, 2);
v1.setValue(4, 1);
v1.setValue(20, 1);
console.log('v1', v1.toDenseVector());
Example:
var nodes = [
{
id : 0,
name : 0
},
{
id: 1,
name : 1
},
{
id : 2,
name : 2
},
{
id : 3,
name : 3
},
{
id : 4,
name : 4
},
{
id : 5,
name : 5
},
{
id : 6,
name : 6
}
];
var edges = [
{
source : nodes[0],
target : nodes[1],
value : 2
},
{
source : nodes[0],
target : nodes[2],
value : 9
},
{
source : nodes[1],
target : nodes[2],
value : 4
},
{
source : nodes[1],
target : nodes[3],
value : 2
},
{
source : nodes[2],
target : nodes[3],
value : 1
},
{
source : nodes[2],
target : nodes[5],
value : 3
},
{
source : nodes[2],
target : nodes[6],
value : 11
},
{
source : nodes[3],
target : nodes[4],
value : 1
},
{
source : nodes[4],
target : nodes[6],
value : 7
},
{
source : nodes[5],
target : nodes[6],
value : 7
}
];
var G = dm.Graph().nodes(nodes).edges(edges).create();
var dk = dm.ShortestPathDijkstra()
.direction('out')
.init_metric(function(){return 0;})
.init_source_metric(function(){return Infinity;})
.comparator(function(a, b){
return b - a;
})
// .source(G.nodes()[0])
.graph(G);
var paths = dk();
var path;
var i;
for(i = 0; i < paths.length; i++){
path = paths[i];
console.log(i, path.map(function(d){
return d.id;
}));
}
var G = dm.Graph().nodes(nodes).edges(edges).create();
var ge = dm.GirvanNewman().graph(G);
var tree = ge();
console.log('tree', tree);
FAQs
A set of data mining tool for javascript
We found that dmjs demonstrated a not healthy version release cadence and project activity because the last version was released a year ago. It has 1 open source maintainer collaborating on the project.
Did you know?
Socket for GitHub automatically highlights issues in each pull request and monitors the health of all your open source dependencies. Discover the contents of your packages and block harmful activity before you install or update your dependencies.
Research
Security News
Socket researchers uncover a malicious npm package posing as a tool for detecting vulnerabilities in Etherium smart contracts.
Security News
Research
A supply chain attack on Rspack's npm packages injected cryptomining malware, potentially impacting thousands of developers.
Research
Security News
Socket researchers discovered a malware campaign on npm delivering the Skuld infostealer via typosquatted packages, exposing sensitive data.