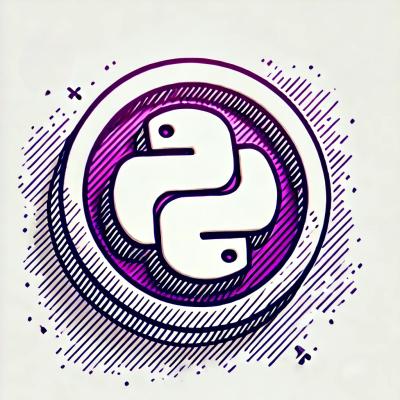
Security News
PyPI Now Supports iOS and Android Wheels for Mobile Python Development
PyPI now supports iOS and Android wheels, making it easier for Python developers to distribute mobile packages.
node-deepresearch
Advanced tools
Keep searching, reading webpages, reasoning until an answer is found (or the token budget is exceeded). Useful for deeply investigating a query.
---
config:
theme: mc
look: handDrawn
---
flowchart LR
subgraph Loop["until budget exceed"]
direction LR
Search["Search"]
Read["Read"]
Reason["Reason"]
end
Query(["Query"]) --> Loop
Search --> Read
Read --> Reason
Reason --> Search
Loop --> Answer(["Answer"])
Unlike OpenAI and Gemini's Deep Research capabilities, we focus solely on delivering accurate answers through our iterative process. We don't optimize for long-form articles – if you need quick, precise answers from deep search, you're in the right place. If you're looking for AI-generated reports like OpenAI/Gemini do, this isn't for you.
git clone https://github.com/jina-ai/node-DeepResearch.git
cd node-DeepResearch
npm install
It is also available on npm but not recommended for now, as the code is still under active development.
We use Gemini (latest gemini-2.0-flash
) / OpenAI / LocalLLM for reasoning, Jina Reader for searching and reading webpages, you can get a free API key with 1M tokens from jina.ai.
export GEMINI_API_KEY=... # for gemini
# export OPENAI_API_KEY=... # for openai
# export LLM_PROVIDER=openai # for openai
export JINA_API_KEY=jina_... # free jina api key, get from https://jina.ai/reader
npm run dev $QUERY
You can also use our official DeepSearch API, hosted and optimized by Jina AI:
https://deepsearch.jina.ai/v1/chat/completions
You can use it with any OpenAI-compatible client. For the authentication Bearer, get your Jina API key from https://jina.ai
If you are building a web/local/mobile client that uses Jina DeepSearch API
, here are some design guidelines:
jina-deepsearch-v1
.<think>...</think>
, <references>...</references>
. Please render them with care.was recorded with
gemini-1.5-flash
, the latestgemini-2.0-flash
leads to much better results!
Query: "what is the latest blog post's title from jina ai?"
3 steps; answer is correct!
Query: "what is the context length of readerlm-v2?"
2 steps; answer is correct!
Query: "list all employees from jina ai that u can find, as many as possible"
11 steps; partially correct! but im not in the list :(
Query: "who will be the biggest competitor of Jina AI"
42 steps; future prediction kind, so it's arguably correct! atm Im not seeing weaviate
as a competitor, but im open for the future "i told you so" moment.
More examples:
# example: no tool calling
npm run dev "1+1="
npm run dev "what is the capital of France?"
# example: 2-step
npm run dev "what is the latest news from Jina AI?"
# example: 3-step
npm run dev "what is the twitter account of jina ai's founder"
# example: 13-step, ambiguious question (no def of "big")
npm run dev "who is bigger? cohere, jina ai, voyage?"
# example: open question, research-like, long chain of thoughts
npm run dev "who will be president of US in 2028?"
npm run dev "what should be jina ai strategy for 2025?"
Note, not every LLM works with our reasoning flow, we need those who support structured output (sometimes called JSON Schema output, object output) well. Feel free to purpose a PR to add more open-source LLMs to the working list.
If you use Ollama or LMStudio, you can redirect the reasoning request to your local LLM by setting the following environment variables:
export LLM_PROVIDER=openai # yes, that's right - for local llm we still use openai client
export OPENAI_BASE_URL=http://127.0.0.1:1234/v1 # your local llm endpoint
export OPENAI_API_KEY=whatever # random string would do, as we don't use it (unless your local LLM has authentication)
export DEFAULT_MODEL_NAME=qwen2.5-7b # your local llm model name
If you have a GUI client that supports OpenAI API (e.g. CherryStudio, Chatbox) , you can simply config it to use this server.
Start the server:
# Without authentication
npm run serve
# With authentication (clients must provide this secret as Bearer token)
npm run serve --secret=your_secret_token
The server will start on http://localhost:3000 with the following endpoint:
# Without authentication
curl http://localhost:3000/v1/chat/completions \
-H "Content-Type: application/json" \
-d '{
"model": "jina-deepsearch-v1",
"messages": [
{
"role": "user",
"content": "Hello!"
}
]
}'
# With authentication (when server is started with --secret)
curl http://localhost:3000/v1/chat/completions \
-H "Content-Type: application/json" \
-H "Authorization: Bearer your_secret_token" \
-d '{
"model": "jina-deepsearch-v1",
"messages": [
{
"role": "user",
"content": "Hello!"
}
],
"stream": true
}'
Response format:
{
"id": "chatcmpl-123",
"object": "chat.completion",
"created": 1677652288,
"model": "jina-deepsearch-v1",
"system_fingerprint": "fp_44709d6fcb",
"choices": [{
"index": 0,
"message": {
"role": "assistant",
"content": "YOUR FINAL ANSWER"
},
"logprobs": null,
"finish_reason": "stop"
}],
"usage": {
"prompt_tokens": 9,
"completion_tokens": 12,
"total_tokens": 21
}
}
For streaming responses (stream: true), the server sends chunks in this format:
{
"id": "chatcmpl-123",
"object": "chat.completion.chunk",
"created": 1694268190,
"model": "jina-deepsearch-v1",
"system_fingerprint": "fp_44709d6fcb",
"choices": [{
"index": 0,
"delta": {
"content": "..."
},
"logprobs": null,
"finish_reason": null
}]
}
Note: The think content in streaming responses is wrapped in XML tags:
<think>
[thinking steps...]
</think>
[final answer]
To build the Docker image for the application, run the following command:
docker build -t deepresearch:latest .
To run the Docker container, use the following command:
docker run -p 3000:3000 --env GEMINI_API_KEY=your_gemini_api_key --env JINA_API_KEY=your_jina_api_key deepresearch:latest
You can also use Docker Compose to manage multi-container applications. To start the application with Docker Compose, run:
docker-compose up
Not sure a flowchart helps, but here it is:
flowchart TD
Start([Start]) --> Init[Initialize context & variables]
Init --> CheckBudget{Token budget<br/>exceeded?}
CheckBudget -->|No| GetQuestion[Get current question<br/>from gaps]
CheckBudget -->|Yes| BeastMode[Enter Beast Mode]
GetQuestion --> GenPrompt[Generate prompt]
GenPrompt --> ModelGen[Generate response<br/>using Gemini]
ModelGen --> ActionCheck{Check action<br/>type}
ActionCheck -->|answer| AnswerCheck{Is original<br/>question?}
AnswerCheck -->|Yes| EvalAnswer[Evaluate answer]
EvalAnswer --> IsGoodAnswer{Is answer<br/>definitive?}
IsGoodAnswer -->|Yes| HasRefs{Has<br/>references?}
HasRefs -->|Yes| End([End])
HasRefs -->|No| GetQuestion
IsGoodAnswer -->|No| StoreBad[Store bad attempt<br/>Reset context]
StoreBad --> GetQuestion
AnswerCheck -->|No| StoreKnowledge[Store as intermediate<br/>knowledge]
StoreKnowledge --> GetQuestion
ActionCheck -->|reflect| ProcessQuestions[Process new<br/>sub-questions]
ProcessQuestions --> DedupQuestions{New unique<br/>questions?}
DedupQuestions -->|Yes| AddGaps[Add to gaps queue]
DedupQuestions -->|No| DisableReflect[Disable reflect<br/>for next step]
AddGaps --> GetQuestion
DisableReflect --> GetQuestion
ActionCheck -->|search| SearchQuery[Execute search]
SearchQuery --> NewURLs{New URLs<br/>found?}
NewURLs -->|Yes| StoreURLs[Store URLs for<br/>future visits]
NewURLs -->|No| DisableSearch[Disable search<br/>for next step]
StoreURLs --> GetQuestion
DisableSearch --> GetQuestion
ActionCheck -->|visit| VisitURLs[Visit URLs]
VisitURLs --> NewContent{New content<br/>found?}
NewContent -->|Yes| StoreContent[Store content as<br/>knowledge]
NewContent -->|No| DisableVisit[Disable visit<br/>for next step]
StoreContent --> GetQuestion
DisableVisit --> GetQuestion
BeastMode --> FinalAnswer[Generate final answer] --> End
I kept the evaluation simple, LLM-as-a-judge and collect some ego questions for evaluation. These are the questions about Jina AI that I know 100% the answer but LLMs do not.
I mainly look at 3 things: total steps, total tokens, and the correctness of the final answer.
npm run eval ./src/evals/questions.json
Here's the table comparing plain gemini-2.0-flash
and gemini-2.0-flash + node-deepresearch
on the ego set.
Plain gemini-2.0-flash
can be run by setting tokenBudget
to zero, skipping the while-loop and directly answering the question.
It should not be surprised that plain gemini-2.0-flash
has a 0% pass rate, as I intentionally filtered out the questions that LLMs can answer.
Metric | gemini-2.0-flash | #188f1bb |
---|---|---|
Pass Rate | 0% | 75% |
Average Steps | 1 | 4 |
Maximum Steps | 1 | 13 |
Minimum Steps | 1 | 2 |
Median Steps | 1 | 3 |
Average Tokens | 428 | 68,574 |
Median Tokens | 434 | 31,541 |
Maximum Tokens | 463 | 363,655 |
Minimum Tokens | 374 | 7,963 |
FAQs
[API](#official-api) | [Evaluation](#evaluation)
The npm package node-deepresearch receives a total of 210 weekly downloads. As such, node-deepresearch popularity was classified as not popular.
We found that node-deepresearch demonstrated a healthy version release cadence and project activity because the last version was released less than a year ago. It has 1 open source maintainer collaborating on the project.
Did you know?
Socket for GitHub automatically highlights issues in each pull request and monitors the health of all your open source dependencies. Discover the contents of your packages and block harmful activity before you install or update your dependencies.
Security News
PyPI now supports iOS and Android wheels, making it easier for Python developers to distribute mobile packages.
Security News
Create React App is officially deprecated due to React 19 issues and lack of maintenance—developers should switch to Vite or other modern alternatives.
Security News
Oracle seeks to dismiss fraud claims in the JavaScript trademark dispute, delaying the case and avoiding questions about its right to the name.