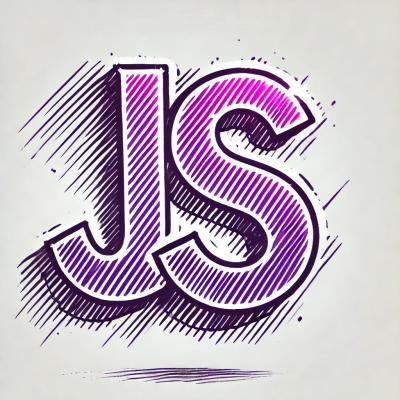
Security News
Oracle Drags Its Feet in the JavaScript Trademark Dispute
Oracle seeks to dismiss fraud claims in the JavaScript trademark dispute, delaying the case and avoiding questions about its right to the name.
Javascript implementation of Weng-Lin Rating, as described at https://www.csie.ntu.edu.tw/~cjlin/papers/online_ranking/online_journal.pdf
Up to 20x faster than TrueSkill!
Model | Speed (higher is better) | Variance | Samples |
---|---|---|---|
Openskill/bradleyTerryFull | 62,643 ops/sec | ±1.09% | 91 runs sampled |
Openskill/bradleyTerryPart | 40,152 ops/sec | ±0.73% | 91 runs sampled |
Openskill/thurstoneMostellerFull | 59,336 ops/sec | ±0.74% | 93 runs sampled |
Openskill/thurstoneMostellerPart | 38,666 ops/sec | ±1.21% | 92 runs sampled |
Openskill/plackettLuce | 23,492 ops/sec | ±0.26% | 91 runs sampled |
TrueSkill | 2,962 ops/sec | ±3.23% | 82 runs sampled |
See this post for more.
Add openskill
to your list of dependencies in package.json
:
npm install --save openskill
If you're writing ES6, you can import
, otherwise use CommonJS's require
import { rating, rate, ordinal } from 'openskill'
Ratings are kept as an object which represent a gaussian curve, with properties where mu
represents the mean, and sigma
represents the spread or standard deviation. Create these with:
> const { rating } = require('openskill')
> const a1 = rating()
{ mu: 25, sigma: 8.333333333333334 }
> const a2 = rating({ mu: 32.444, sigma: 5.123 })
{ mu: 32.444, sigma: 5.123 }
> const b1 = rating({ mu: 43.381, sigma: 2.421 })
{ mu: 43.381, sigma: 2.421 }
> const b2 = rating({ mu: 25.188, sigma: 6.211 })
{ mu: 25.188, sigma: 6.211 }
If a1
and a2
are on a team, and wins against a team of b1
and b2
, send this into rate
> const { rate } = require('openskill')
> const [[x1, x2], [y1, y2]] = rate([[a1, a2], [b1, b2]])
[
[
{ mu: 28.67..., sigma: 8.07...},
{ mu: 33.83..., sigma: 5.06...}
],
[
{ mu: 43.07..., sigma: 2.42...},
{ mu: 23.15..., sigma: 6.14...}
]
]
Teams can be asymmetric, too! For example, a game like Axis and Allies can be 3 vs 2, and this can be modeled here.
When displaying a rating, or sorting a list of ratings, you can use ordinal
> const { ordinal } = require('openskill')
> ordinal({ mu: 43.07, sigma: 2.42})
35.81
By default, this returns mu - 3*sigma
, showing a rating for which there's a 99.7% likelihood the player's true rating is higher, so with early games, a player's ordinal rating will usually go up and could go up even if that player loses.
If your teams are listed in one order but your ranking is in a different order, for convenience you can specify a ranks
option, such as
> const a1 = b1 = c1 = d1 = rating()
> const [[a2], [b2], [c2], [d2]] = rate([[a1], [b1], [c1], [d1]], {
rank: [4, 1, 3, 2] // 🐌 🥇 🥉 🥈
})
[
[{ mu: 20.963, sigma: 8.084 }], // 🐌
[{ mu: 27.795, sigma: 8.263 }], // 🥇
[{ mu: 24.689, sigma: 8.084 }], // 🥉
[{ mu: 26.553, sigma: 8.179 }], // 🥈
]
It's assumed that the lower ranks are better (wins), while higher ranks are worse (losses). You can provide a score
instead, where lower is worse and higher is better. These can just be raw scores from the game, if you want.
Ties should have either equivalent rank or score.
> const a1 = b1 = c1 = d1 = rating()
> const [[a2], [b2], [c2], [d2]] = rate([[a1], [b1], [c1], [d1]], {
score: [37, 19, 37, 42] // 🥈 🐌 🥈 🥇
})
[
[{ mu: 24.689, sigma: 8.179 }], // 🥈
[{ mu: 22.826, sigma: 8.179 }], // 🐌
[{ mu: 24.689, sigma: 8.179 }], // 🥈
[{ mu: 27.795, sigma: 8.263 }], // 🥇
]
For a given match of any number of teams, using predictWin
you can find a relative
odds that each of those teams will win.
> const { predictWin } = require('openskill')
> const a1 = rating()
> const a2 = rating({mu:33.564, sigma:1.123})
> const predictions = predictWin([[a1], [a2]])
[ 0.45110899943132493, 0.5488910005686751 ]
> predictions[0] + predictions[1]
1
Also for a given match, using predictDraw
you can get the relative chance that these
teams will draw. The number returned here should be treated as relative to other matches, but in reality the odds of an actual legal draw will be impacted by some meta-function based on the rules of the game.
> const { predictDraw } = require('openskill')
> const prediction = predictDraw([[a1], [a2]])
0.09025530533015186
This can be used in a similar way that you might use quality in TrueSkill if you were optimizing a matchmaking system, or optimizing an tournament tree structure for exciting finals and semi-finals such as in the NCAA.
By default, we use a Plackett-Luce model, which is probably good enough for most cases. When speed is an issue, the library runs faster with other models
import { bradleyTerryFull } from './models'
const [[a2], [b2]] = rate([[a1], [b1]], {
model: bradleyTerryFull,
})
FAQs
Weng-Lin Bayesian approximation method for online skill-ranking.
The npm package openskill receives a total of 291 weekly downloads. As such, openskill popularity was classified as not popular.
We found that openskill demonstrated a healthy version release cadence and project activity because the last version was released less than a year ago. It has 0 open source maintainers collaborating on the project.
Did you know?
Socket for GitHub automatically highlights issues in each pull request and monitors the health of all your open source dependencies. Discover the contents of your packages and block harmful activity before you install or update your dependencies.
Security News
Oracle seeks to dismiss fraud claims in the JavaScript trademark dispute, delaying the case and avoiding questions about its right to the name.
Security News
The Linux Foundation is warning open source developers that compliance with global sanctions is mandatory, highlighting legal risks and restrictions on contributions.
Security News
Maven Central now validates Sigstore signatures, making it easier for developers to verify the provenance of Java packages.