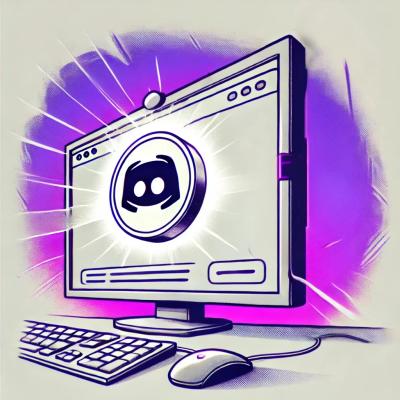
Research
Security News
Malicious PyPI Package ‘pycord-self’ Targets Discord Developers with Token Theft and Backdoor Exploit
Socket researchers uncover the risks of a malicious Python package targeting Discord developers.
semantic-chunking
Advanced tools
Semantically create chunks from large texts. Useful for workflows involving large language models (LLMs).
Semantically create chunks from large texts.
Useful for workflows involving large language models (LLMs).
npm install semantic-chunking
Basic usage:
import { chunkit } from 'semantic-chunking';
const text = "some long text...";
const chunkitOptions = {};
const myChunks = await chunkit(text, chunkitOptions);
NOTE 🚨 The Embedding model (onnxEmbeddingModel
) will be downloaded to this package's cache directory the first it is run (file size will depend on the specified model; see the model's table ).
chunkit
accepts a text string and an optional configuration object. Here are the details for each parameter:
text
: String to be split into chunks.
Chunkit Options Object:
logging
: Boolean (optional, default false
) - Enables logging of detailed processing steps.maxTokenSize
: Integer (optional, default 500
) - Maximum token size for each chunk.similarityThreshold
: Float (optional, default 0.456
) - Threshold to determine if sentences are similar enough to be in the same chunk. A higher value demands higher similarity.dynamicThresholdLowerBound
: Float (optional, default 0.2
) - Minimum possible dynamic similarity threshold.dynamicThresholdUpperBound
: Float (optional, default 0.8
) - Maximum possible dynamic similarity threshold.numSimilaritySentencesLookahead
: Integer (optional, default 2
) - Number of sentences to look ahead for calculating similarity.combineChunks
: Boolean (optional, default true
) - Determines whether to reblance and combine chunks into larger ones up to the max token limit.combineChunksSimilarityThreshold
: Float (optional, default 0.5
) - Threshold for combining chunks based on similarity during the rebalance and combining phase.onnxEmbeddingModel
: String (optional, default Xenova/all-MiniLM-L6-v2
) - ONNX model used for creating embeddings.onnxEmbeddingModelQuantized
: Boolean (optional, default true
) - Indicates whether to use a quantized version of the embedding model.localModelPath
: String (optional, default null
) - Local path to save and load models (example: ./models
).modelCacheDir
: String (optional, default null
) - Directory to cache downloaded models (example: ./models
).Example 1: Basic usage with custom similarity threshold:
import { chunkit } from 'semantic-chunking';
import fs from 'fs';
async function main() {
const text = await fs.promises.readFile('./test.txt', 'utf8');
let myChunks = await chunkit(text, { similarityThreshold: 0.3 });
myChunks.forEach((chunk, index) => {
console.log(`\n-- Chunk ${index + 1} --`);
console.log(chunk);
});
}
main();
Example 2: Chunking with a small max token size:
import { chunkit } from 'semantic-chunking';
let frogText = "A frog hops into a deli and croaks to the cashier, \"I'll have a sandwich, please.\" The cashier, surprised, quickly makes the sandwich and hands it over. The frog takes a big bite, looks around, and then asks, \"Do you have any flies to go with this?\" The cashier, taken aback, replies, \"Sorry, we're all out of flies today.\" The frog shrugs and continues munching on its sandwich, clearly unfazed by the lack of fly toppings. Just another day in the life of a sandwich-loving amphibian! 🐸🥪";
async function main() {
let myFrogChunks = await chunkit(frogText, { maxTokenSize: 65 });
console.log("myFrogChunks", myFrogChunks);
}
main();
Look at the example.js
file in the root of this project for a more complex example of using all the optional parameters.
The behavior of the chunkit
function can be finely tuned using several optional parameters in the options object. Understanding how each parameter affects the function can help you optimize the chunking process for your specific requirements.
logging
false
maxTokenSize
500
similarityThreshold
0.456
dynamicThresholdLowerBound
0.2
dynamicThresholdUpperBound
0.8
numSimilaritySentencesLookahead
2
combineChunks
true
maxTokenSize
. This can enhance the readability of the output by grouping closely related content more effectively.combineChunksSimilarityThreshold
0.4
similarityThreshold
, but specifically for rebalancing existing chunks. Adjusting this parameter can help in fine-tuning the granularity of the final chunks.onnxEmbeddingModel
Xenova/paraphrase-multilingual-MiniLM-L12-v2
onnxEmbeddingModelQuantized
true
Model | Quantized | Link | Size |
---|---|---|---|
Xenova/all-MiniLM-L6-v2 | true | https://huggingface.co/Xenova/all-MiniLM-L6-v2 | 23 MB |
Xenova/all-MiniLM-L6-v2 | false | https://huggingface.co/Xenova/all-MiniLM-L6-v2 | 90.4 MB |
Xenova/paraphrase-multilingual-MiniLM-L12-v2 | true | https://huggingface.co/Xenova/paraphrase-multilingual-MiniLM-L12-v2 | 118 MB |
Xenova/all-distilroberta-v1 | true | https://huggingface.co/Xenova/all-distilroberta-v1 | 82.1 MB |
Xenova/all-distilroberta-v1 | false | https://huggingface.co/Xenova/all-distilroberta-v1 | 326 MB |
BAAI/bge-base-en-v1.5 | false | https://huggingface.co/BAAI/bge-base-en-v1.5 | 436 MB |
BAAI/bge-small-en-v1.5 | false | https://huggingface.co/BAAI/bge-small-en-v1.5 | 133 MB |
Each of these parameters allows you to customize the chunkit
function to better fit the text size, content complexity, and performance requirements of your application.
cramit
- 🧼 The Quick & DirtyThere is an additional function you can import to just "cram" sentences together till they meet your target token size for when you just need quick, high desity chunks.
Basic usage:
import { cramit } from 'semantic-chunking';
let frogText = "A frog hops into a deli and croaks to the cashier, \"I'll have a sandwich, please.\" The cashier, surprised, quickly makes the sandwich and hands it over. The frog takes a big bite, looks around, and then asks, \"Do you have any flies to go with this?\" The cashier, taken aback, replies, \"Sorry, we're all out of flies today.\" The frog shrugs and continues munching on its sandwich, clearly unfazed by the lack of fly toppings. Just another day in the life of a sandwich-loving amphibian! 🐸🥪";
async function main() {
let myFrogChunks = await cramit(frogText, { maxTokenSize: 65 });
console.log("myFrogChunks", myFrogChunks);
}
main();
Look at the example2.js
file in the root of this project for a more complex example of using all the optional parameters.
The behavior of the chunkit
function can be finely tuned using several optional parameters in the options object. Understanding how each parameter affects the function can help you optimize the chunking process for your specific requirements.
logging
false
maxTokenSize
500
onnxEmbeddingModel
Xenova/paraphrase-multilingual-MiniLM-L12-v2
onnxEmbeddingModelQuantized
true
Fill out the tools/download-models.list.json
file with a list of models you want pre-downloaded, and if they are quantized or not (See the Curated ONNX Embedding Models section above for a list of models to try)
[1.5.0] - 2024-10-11
FAQs
Semantically create chunks from large texts. Useful for workflows involving large language models (LLMs).
The npm package semantic-chunking receives a total of 206 weekly downloads. As such, semantic-chunking popularity was classified as not popular.
We found that semantic-chunking demonstrated a healthy version release cadence and project activity because the last version was released less than a year ago. It has 0 open source maintainers collaborating on the project.
Did you know?
Socket for GitHub automatically highlights issues in each pull request and monitors the health of all your open source dependencies. Discover the contents of your packages and block harmful activity before you install or update your dependencies.
Research
Security News
Socket researchers uncover the risks of a malicious Python package targeting Discord developers.
Security News
The UK is proposing a bold ban on ransomware payments by public entities to disrupt cybercrime, protect critical services, and lead global cybersecurity efforts.
Security News
Snyk's use of malicious npm packages for research raises ethical concerns, highlighting risks in public deployment, data exfiltration, and unauthorized testing.