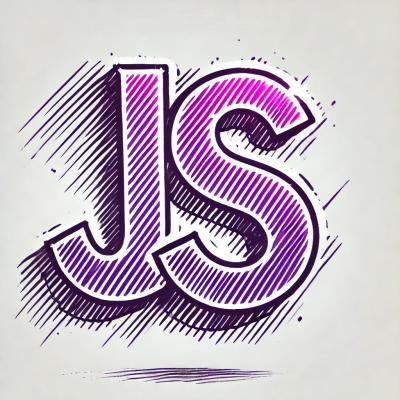
Security News
Oracle Drags Its Feet in the JavaScript Trademark Dispute
Oracle seeks to dismiss fraud claims in the JavaScript trademark dispute, delaying the case and avoiding questions about its right to the name.
Adas is an adaptive optimizer for scheduling the learning rate in training Convolutional Neural Networks (CNN)
This repository contains a PyTorch implementation of the Adas learning rate scheduler algorithm as well as the Knowledge Gain and Mapping Condition metrics.
Visit the paper
branch to see the paper-related code. You can use that code to replicate experiments from the paper.
Adas is released under the MIT License (refer to the LICENSE file for more information)
Permissions | Conditions | Limitations |
---|---|---|
@article{hosseini2020adas,
title={Adas: Adaptive Scheduling of Stochastic Gradients},
author={Hosseini, Mahdi S and Plataniotis, Konstantinos N},
journal={arXiv preprint arXiv:2006.06587},
year={2020}
}
Figure 1: Training performance using different optimizers across three datasets and two CNNs
Table 1: Image classification performance (test accuracy) with fixed budget epoch of ResNet34 training
Please refer to QC on Wiki for more information on two metrics of knowledge gain and mapping condition for monitoring training quality of CNNs
We use Python 3.7
.
Please refer to Requirements on Wiki for complete guideline.
Adas introduces no overhead (very minimal) over adaptive optimizers e.g. all mSGD+StepLR, mSGD+Adas, AdaM consume 40~43 sec/epoch to train ResNet34/CIFAR10 using the same PC/GPU platform
src/adas
into your local code base and use them directly. Note that you will probably need to modify the imports to be consistent with however you perform imports in your codebase.All source code can be found in src/adas
For more information, also refer to Installation on Wiki
The use Adas, simply import the Adas(torch.optim.optimier.Optimizer)
class and use it as follows:
from adas import Adas
optimizer = Adas(params=list(model.parameters()),
lr: float = ???,
beta: float = 0.8
step_size: int = None,
gamma: float = 1,
momentum: float = 0,
dampening: float = 0,
weight_decay: float = 0,
nesterov: bool = False):
...
for epoch in epochs:
for batch in train_dataset:
...
loss.backward()
optimizer.step()
optimizer.epoch_step(epoch)
Note, optipmizer.epoch_step()
is just to be called at the end of each epoch.
Note the following:
/tmp
, so if you don't have a /tmp
folder (i.e. you're on Windows), then correct this if you wish to run the tests yourselfFAQs
Python package for AdaS: Adaptive Scheduling of Stochastic Gradients
We found that adas demonstrated a healthy version release cadence and project activity because the last version was released less than a year ago. It has 1 open source maintainer collaborating on the project.
Did you know?
Socket for GitHub automatically highlights issues in each pull request and monitors the health of all your open source dependencies. Discover the contents of your packages and block harmful activity before you install or update your dependencies.
Security News
Oracle seeks to dismiss fraud claims in the JavaScript trademark dispute, delaying the case and avoiding questions about its right to the name.
Security News
The Linux Foundation is warning open source developers that compliance with global sanctions is mandatory, highlighting legal risks and restrictions on contributions.
Security News
Maven Central now validates Sigstore signatures, making it easier for developers to verify the provenance of Java packages.