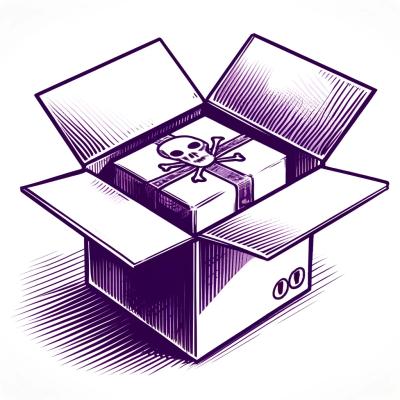
Security News
Research
Data Theft Repackaged: A Case Study in Malicious Wrapper Packages on npm
The Socket Research Team breaks down a malicious wrapper package that uses obfuscation to harvest credentials and exfiltrate sensitive data.
This project is an alternative independent asyncio implementation of Celery.
Quoting Celery documentation:
Celery is written in Python, but the protocol can be implemented in any language.
And aio-celery does exactly this, it (re)implements Celery Message Protocol (in Python) in order to unlock access to asyncio tasks and workers.
The most notable feature of aio-celery is that it does not depend on Celery codebase. It is written completely from scratch as a thin wrapper around aio-pika (which is an asyncronous RabbitMQ python driver) and it has no other dependencies (except for redis-py for result backend support, but this dependency is optional).
There have been attempts to create asyncio Celery Pools before, and celery-pool-asyncio is one such example, but its implementation, due to convoluted structure of the original Celery codebase, is (by necessity) full of monkeypatching and other fragile techniques. This fragility was apparently the reason why this library became incompatible with Celery version 5.
Celery project itself clearly struggles with implementing Asyncio Coroutine support, constantly delaying this feature due to apparent architectural difficulties.
This project was created in an attempt to solve the same problem but using the opposite approach. It implements only a limited (but still usable — that is the whole point) subset of Celery functionality without relying on Celery code at all — the goal is to mimic the basic wire protocol and to support a subset of Celery API minimally required for running and manipulating tasks.
What is supported:
@app.task
decorator, delay
and apply_async
task methods, AsyncResult
class etc.countdown
, eta
, queue
, priority
, etc.Important design decisions for aio-celery:
Install using pip:
pip install aio-celery
If you intend to use Redis result backend for storing task results, run this command:
pip install aio-celery[redis]
Define Celery
application instance and register a task:
# hello.py
import asyncio
from aio_celery import Celery
app = Celery()
@app.task(name="add-two-numbers")
async def add(a, b):
await asyncio.sleep(5)
return a + b
Then run worker:
$ aio_celery worker hello:app
Queue some tasks:
# publish.py
import asyncio
from hello import add, app
async def publish():
async with app.setup():
tasks = [add.delay(n, n) for n in range(50000)]
await asyncio.gather(*tasks)
asyncio.run(publish())
$ python3 publish.py
The last script concurrently publishes 50000 messages to RabbitMQ. It takes about 8 seconds to finish, with gives average publishing rate of about 6000 messages per second.
import asyncio
from aio_celery import Celery
from aio_celery.exceptions import TimeoutError
app = Celery()
app.conf.update(
result_backend="redis://localhost:6379",
)
@app.task(name="do-something")
async def foo(x, y, z):
await asyncio.sleep(5)
return x + y - z
async def main():
async with app.setup():
result = await foo.delay(1, 2, 3)
try:
value = await result.get(timeout=10)
except TimeoutError:
print("Result is not ready after 10 seconds")
else:
print("Result is", value)
if __name__ == "__main__":
asyncio.run(main())
import contextlib
import asyncpg
from aio_celery import Celery
app = Celery()
@app.define_app_context
@contextlib.asynccontextmanager
async def setup_context():
async with asyncpg.create_pool("postgresql://localhost:5432", max_size=10) as pool:
yield {"pool": pool}
@app.task
async def get_postgres_version():
async with app.context["pool"].acquire() as conn:
version = await conn.fetchval("SELECT version()")
return version
import random
from aio_celery import Celery
app = Celery()
@app.task(name="add-two-numbers", bind=True, max_retries=3)
async def add(self, a, b):
if random.random() > 0.25:
# Sends task to queue and raises `aio_celery.exception.Retry` exception.
await self.retry(countdown=2)
Support for RabbitMQ Message Priorities:
import asyncio
from aio_celery import Celery
app = Celery()
app.conf.update(
task_default_priority=5, # global default for all tasks
task_default_queue="queue-a", # global default for all tasks
task_queue_max_priority=10, # sets `x-max-priority` argument for RabbitMQ Queue
)
@app.task(
name="add-two-numbers",
priority=6, # per task default (overrides global default)
queue="queue-b", # per task default (overrider global default)
)
async def add(a, b):
await asyncio.sleep(3)
return a + b
async def main():
async with app.setup():
await add.apply_async(
args=(2, 3),
priority=7, # overrides all defaults
queue="queue-c", # overrides all defaults
)
if __name__ == "__main__":
asyncio.run(main())
See also RabbitMQ documentation on priorities.
import asyncio
from aio_celery import Celery
app = Celery()
app.conf.update(
result_backend="redis://localhost:6379",
)
async def main():
async with app.setup():
result = await app.send_task(
"add-two-numbers",
args=(3, 4),
queue="high-priority",
countdown=30,
)
print(await result.get(timeout=5))
if __name__ == "__main__":
asyncio.run(main())
@shared_task
decoratorAnalogous to original Celery feature,
the @shared_task
decorator lets you create tasks without having any concrete app instance:
from aio_celery import Celery, shared_task
@shared_task
async def add(a, b):
return a + b
app = Celery() # `add` task is already registered on `app` instance
https://github.com/cr0hn/aiotasks
https://github.com/the-wondersmith/celery-aio-pool
https://github.com/kai3341/celery-pool-asyncio
https://github.com/taskiq-python/taskiq
https://github.com/celery/celery/issues/3884
https://github.com/celery/celery/issues/7874
FAQs
Celery worker for running asyncio coroutine tasks
We found that aio-celery demonstrated a healthy version release cadence and project activity because the last version was released less than a year ago. It has 1 open source maintainer collaborating on the project.
Did you know?
Socket for GitHub automatically highlights issues in each pull request and monitors the health of all your open source dependencies. Discover the contents of your packages and block harmful activity before you install or update your dependencies.
Security News
Research
The Socket Research Team breaks down a malicious wrapper package that uses obfuscation to harvest credentials and exfiltrate sensitive data.
Research
Security News
Attackers used a malicious npm package typosquatting a popular ESLint plugin to steal sensitive data, execute commands, and exploit developer systems.
Security News
The Ultralytics' PyPI Package was compromised four times in one weekend through GitHub Actions cache poisoning and failure to rotate previously compromised API tokens.