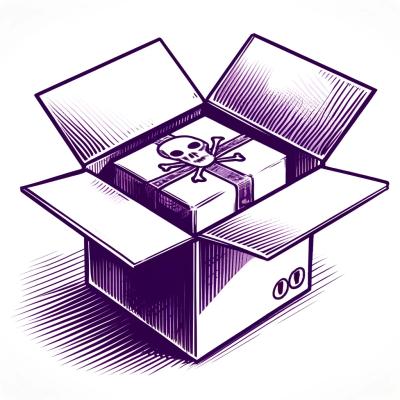
Security News
Research
Data Theft Repackaged: A Case Study in Malicious Wrapper Packages on npm
The Socket Research Team breaks down a malicious wrapper package that uses obfuscation to harvest credentials and exfiltrate sensitive data.
State-of-the art Automated Machine Learning python library for Tabular Data
Binary Classification
Regression
Multiclass Classification (in progress...)
The bigger, the better
From AutoML-Benchmark
pip install automl-alex
Classifier:
from automl_alex import AutoMLClassifier
model = AutoMLClassifier()
model.fit(X_train, y_train, timeout=600)
predicts = model.predict(X_test)
Regression:
from automl_alex import AutoMLRegressor
model = AutoMLRegressor()
model.fit(X_train, y_train, timeout=600)
predicts = model.predict(X_test)
DataPrepare:
from automl_alex import DataPrepare
de = DataPrepare()
X_train = de.fit_transform(X_train)
X_test = de.transform(X_test)
Simple Models Wrapper:
from automl_alex import LightGBMClassifier
model = LightGBMClassifier()
model.fit(X_train, y_train)
predicts = model.predict_proba(X_test)
model.opt(X_train, y_train,
timeout=600, # optimization time in seconds,
)
predicts = model.predict_proba(X_test)
More examples in the folder ./examples:
It integrates many popular frameworks:
Categorical Features
Numerical Features
Binary Features
Text
Datetime
Timeseries
Image
Works with optuna-dashboard
Run
$ optuna-dashboard sqlite:///db.sqlite3
Feature Generation
Save/Load and Predict on New Samples
Advanced Logging
Add opt Pruners
Docs Site
DL Encoders
Add More libs (NNs)
Multiclass Classification
Build pipelines
FAQs
State-of-the art Automated Machine Learning python library for Tabular Data
We found that automl-alex demonstrated a healthy version release cadence and project activity because the last version was released less than a year ago. It has 1 open source maintainer collaborating on the project.
Did you know?
Socket for GitHub automatically highlights issues in each pull request and monitors the health of all your open source dependencies. Discover the contents of your packages and block harmful activity before you install or update your dependencies.
Security News
Research
The Socket Research Team breaks down a malicious wrapper package that uses obfuscation to harvest credentials and exfiltrate sensitive data.
Research
Security News
Attackers used a malicious npm package typosquatting a popular ESLint plugin to steal sensitive data, execute commands, and exploit developer systems.
Security News
The Ultralytics' PyPI Package was compromised four times in one weekend through GitHub Actions cache poisoning and failure to rotate previously compromised API tokens.