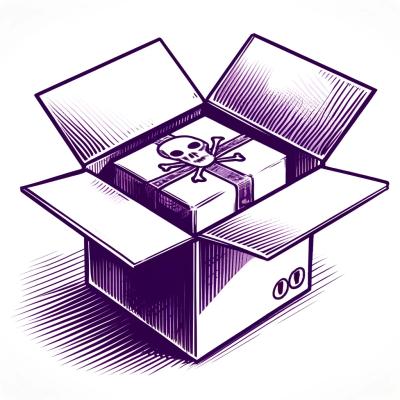
Security News
Research
Data Theft Repackaged: A Case Study in Malicious Wrapper Packages on npm
The Socket Research Team breaks down a malicious wrapper package that uses obfuscation to harvest credentials and exfiltrate sensitive data.
PyTorch Implementation of the linear methods and model from the paper "BitNet: Scaling 1-bit Transformers for Large Language Models"
BitLinear = tensor -> layernorm -> Binarize -> abs max quantization -> dequant
"The implementation of the BitNet architecture is quite simple, requiring only the replacement of linear projections (i.e., nn.Linear in PyTorch) in the Transformer. " -- BitNet is really easy to implement just swap out the linears with the BitLinear modules!
BitLinear
has been optimized and we now have a Bit Attention BitMGQA
That implements BitLinear into the attention mechanism. Multi Grouped Query Attention is also widely recognized as the best attention for its fast decoding and long context handling, thanks to Frank for his easy to use implementation!pip install bitnet
BitLinear
import torch
from bitnet import BitLinear
# Input
x = torch.randn(10, 1000, 512)
# BitLinear layer
layer = BitLinear(512, 400)
# Output
y = layer(x)
print(y)
import torch
from bitnet import BitLinearNew
# Create a random tensor of shape (16, 10)
x = torch.randn(16, 1000, 512)
# Create an instance of the BitLinearNew class with input size 10, output size 20, and 2 groups
layer = BitLinearNew(
512,
20,
)
# Perform a forward pass through the BitLinearNew layer with input x
output = layer(x)
# Print the output tensor
print(output)
print(output.shape)
BitNetTransformer
# Import the necessary libraries
import torch
from bitnet import BitNetTransformer
# Create a random tensor of integers
x = torch.randint(0, 20000, (1, 1024))
# Initialize the BitNetTransformer model
bitnet = BitNetTransformer(
num_tokens=20000, # Number of unique tokens in the input
dim=1024, # Dimension of the input and output embeddings
depth=6, # Number of transformer layers
heads=8, # Number of attention heads
ff_mult=4, # Multiplier for the hidden dimension in the feed-forward network
)
# Pass the tensor through the transformer model
logits = bitnet(x)
# Print the shape of the output
print(logits)
BitAttention
This Attention has been modified to use BitLinear instead of the default linear projection. It's also using Multi-Grouped Query Attention instead of regular multi-head attention for faster decoding and longer context handling.
import torch
from bitnet import BitMGQA
# Create a random tensor of shape (1, 10, 512)
x = torch.randn(1, 10, 512)
# Create an instance of the BitMGQA model with input size 512, 8 attention heads, and 4 layers
gqa = BitMGQA(512, 8, 4)
# Pass the input tensor through the BitMGQA model and get the output and attention weights
out, _ = gqa(x, x, x, need_weights=True)
# Print the shapes of the output tensor and attention tensor
print(out)
BitFeedForward
import torch
from bitnet import BitFeedForward
# Create a random input tensor of shape (10, 512)
x = torch.randn(10, 512)
# Create an instance of the BitFeedForward class with the following parameters:
# - input_dim: 512
# - hidden_dim: 512
# - num_layers: 4
# - swish: True (use Swish activation function)
# - post_act_ln: True (apply Layer Normalization after each activation)
# - dropout: 0.1 (apply dropout with a probability of 0.1)
ff = BitFeedForward(512, 512, 4, swish=True, post_act_ln=True, dropout=0.1)
# Apply the BitFeedForward network to the input tensor x
y = ff(x)
# Print the shape of the output tensor y
print(y) # torch.Size([10, 512])
from bitnet import BitNetInference
bitnet = BitNetInference()
bitnet.load_model("../model_checkpoint.pth") # Download model
output_str = bitnet.generate("The dog jumped over the ", 512)
print(output_str)
import torch
from transformers import AutoModelForSequenceClassification, AutoTokenizer
from bitnet import replace_linears_in_hf
# Load a model from Hugging Face's Transformers
model_name = "bert-base-uncased"
tokenizer = AutoTokenizer.from_pretrained(model_name)
model = AutoModelForSequenceClassification.from_pretrained(model_name)
# Replace Linear layers with BitLinear
replace_linears_in_hf(model)
# Example text to classify
text = "Replace this with your text"
inputs = tokenizer(
text, return_tensors="pt", padding=True, truncation=True, max_length=512
)
# Perform inference
model.eval() # Set the model to evaluation mode
with torch.no_grad():
outputs = model(**inputs)
predictions = torch.nn.functional.softmax(outputs.logits, dim=-1)
print(predictions)
# Process predictions
predicted_class_id = predictions.argmax().item()
print(f"Predicted class ID: {predicted_class_id}")
# Optionally, map the predicted class ID to a label, if you know the classification labels
# labels = ["Label 1", "Label 2", ...] # Define your labels corresponding to the model's classes
# print(f"Predicted label: {labels[predicted_class_id]}")
import torch
from torch import nn
from bitnet import replace_linears_in_pytorch_model
# Define a simple model
model = nn.Sequential(
nn.Linear(10, 20),
nn.ReLU(),
nn.Linear(20, 30),
)
print("Before replacement:")
print(model)
# Replace nn.Linear with BitLinear
replace_linears_in_pytorch_model(model)
print("After replacement:")
print(model)
# Now you can use the model for training or inference
# For example, pass a random input through the model
input = torch.randn(1, 10)
output = model(input)
python setup.py build_ext --inplace
import torch
import gemm_lowbit_ext # This imports the compiled module
# Example usage
a = torch.randn(10, 20, dtype=torch.half, device='cuda') # Example tensor
b = torch.randn(20, 30, dtype=torch.half, device='cuda') # Example tensor
c = torch.empty(10, 30, dtype=torch.half, device='cuda') # Output tensor
w_scale = 1.0 # Example scale factor
x_scale = 1.0 # Example scale factor
# Call the custom CUDA GEMM operation
gemm_lowbit_ext.gemm_lowbit(a, b, c, w_scale, x_scale)
print(c) # View the result
BitLora
Implementation of BitLora!
import torch
from bitnet import BitLora
# Random text tensor
x = torch.randn(1, 12, 200)
# Create an instance of the BitLora model
model = BitLora(in_features=200, out_features=200, rank=4, lora_alpha=1)
# Perform the forward pass
out = model(x)
# Print the shape of the output tensor
print(out.shape)
import torch
from bitnet import BitMamba
# Create a tensor of size (2, 10) with random values between 0 and 100
x = torch.randint(0, 100, (2, 10))
# Create an instance of the BitMamba model with input size 512, hidden size 100, output size 10, and depth size 6
model = BitMamba(512, 100, 10, 6, return_tokens=True)
# Pass the input tensor through the model and get the output
output = model(x)
# Print the output tensor
print(output)
# Print the shape of the output tensor
print(output.shape)
BitMoE
import torch
from bitnet.bit_moe import BitMoE
# Create input tensor
x = torch.randn(2, 4, 8)
# Create BitMoE model with specified input and output dimensions
model = BitMoE(8, 4, 2)
# Forward pass through the model
output = model(x)
# Print the output
print(output)
MIT
@misc{2310.11453,
Author = {Hongyu Wang and Shuming Ma and Li Dong and Shaohan Huang and Huaijie Wang and Lingxiao Ma and Fan Yang and Ruiping Wang and Yi Wu and Furu Wei},
Title = {BitNet: Scaling 1-bit Transformers for Large Language Models},
Year = {2023},
Eprint = {arXiv:2310.11453},
}
BitNetTransformer
FAQs
bitnet - Pytorch
We found that bitnet demonstrated a healthy version release cadence and project activity because the last version was released less than a year ago. It has 1 open source maintainer collaborating on the project.
Did you know?
Socket for GitHub automatically highlights issues in each pull request and monitors the health of all your open source dependencies. Discover the contents of your packages and block harmful activity before you install or update your dependencies.
Security News
Research
The Socket Research Team breaks down a malicious wrapper package that uses obfuscation to harvest credentials and exfiltrate sensitive data.
Research
Security News
Attackers used a malicious npm package typosquatting a popular ESLint plugin to steal sensitive data, execute commands, and exploit developer systems.
Security News
The Ultralytics' PyPI Package was compromised four times in one weekend through GitHub Actions cache poisoning and failure to rotate previously compromised API tokens.