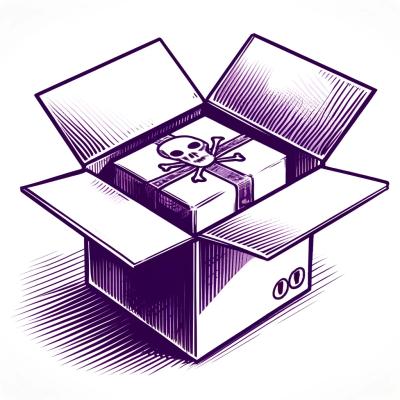
Security News
Research
Data Theft Repackaged: A Case Study in Malicious Wrapper Packages on npm
The Socket Research Team breaks down a malicious wrapper package that uses obfuscation to harvest credentials and exfiltrate sensitive data.
Change point detection aims to identify structural breaks in the probability distribution of a time series. Existing methods either assume a parametric model for within-segment distributions or are based on ranks or distances and thus fail in scenarios with a reasonably large dimensionality.
changeforest
implements a classifier-based algorithm that consistently estimates
change points without any parametric assumptions, even in high-dimensional scenarios.
It uses the out-of-bag probability predictions of a random forest to construct a
classifier log-likelihood ratio that gets optimized using a computationally feasible two-step
method.
See [1] for details.
To install from conda-forge
(recommended), run
conda install -c conda-forge changeforest
To install from PyPI
, run
pip install changeforest
In the following example, we perform random forest-based change point detection on
a simulated dataset with n=600
observations and covariance shifts at t=200, 400
.
In [1]: import numpy as np
...:
...: Sigma = np.full((5, 5), 0.7)
...: np.fill_diagonal(Sigma, 1)
...:
...: rng = np.random.default_rng(12)
...: X = np.concatenate(
...: (
...: rng.normal(0, 1, (200, 5)),
...: rng.multivariate_normal(np.zeros(5), Sigma, 200, method="cholesky"),
...: rng.normal(0, 1, (200, 5)),
...: ),
...: axis=0,
...: )
The simulated dataset X
coincides with the change in covariance (CIC) setup
described in [1]. Observations in the first and last segments are independently drawn
from a standard multivariate Gaussian distribution. Observations in the second segment
are i.i.d. normal with mean zero and unit variance, but with a covariance of ρ = 0.7
between coordinates. This is a challenging scenario.
In [2]: from changeforest import changeforest
...:
...: result = changeforest(X, "random_forest", "bs")
...: result
Out[2]:
best_split max_gain p_value
(0, 600] 400 14.814 0.005
¦--(0, 400] 200 59.314 0.005
¦ ¦--(0, 200] 6 -1.95 0.67
¦ °--(200, 400] 393 -8.668 0.81
°--(400, 600] 412 -9.047 0.66
In [3]: result.split_points()
Out[3]: [200, 400]
changeforest
correctly identifies the change points at t=200
and t=400
. The
changeforest
function returns a BinarySegmentationResult
. We use its plot
method
to investigate the gain curves maximized by the change point estimates:
In [4]: result.plot().show()
For method="random_forest"
and method="knn"
, the changeforest
algorithm uses a two-step approach to
find an optimizer of the gain. This fits a classifier for three split candidates
at the segment's 1/4, 1/2 and 3/4 quantiles, computes approximate gain curves using
the resulting classifier log-likelihood ratios and selects the overall optimizer as a second guess.
We can investigate the gain curves from the optimizer using the plot
method of OptimizerResult
.
The initial guesses are marked in blue.
In [5]: result.optimizer_result.plot().show()
One can observe that the approximate gain curves are piecewise linear, with maxima around the true underlying change points.
The BinarySegmentationResult
returned by changeforest
is a tree-like object with attributes
start
, stop
, best_split
, max_gain
, p_value
, is_significant
, optimizer_result
, model_selection_result
, left
, right
and segments
.
These can be interesting to investigate the output of the algorithm further.
The changeforest
algorithm can be tuned with hyperparameters. See
here
for their descriptions and default values. In Python, the parameters can
be specified with the Control
class,
which can be passed to changeforest
. The following will build random forests with
50 trees:
In [6]: from changeforest import Control
...: changeforest(X, "random_forest", "bs", Control(random_forest_n_estimators=50))
Out[6]:
best_split max_gain p_value
(0, 600] 416 7.463 0.01
¦--(0, 416] 200 43.935 0.005
¦ ¦--(0, 200] 193 -14.993 0.945
¦ °--(200, 416] 217 -9.13 0.085
°--(416, 600] 591 -12.07 1
The changeforest
algorithm still detects change points at t=200
, but is slightly off
with t=416
.
Due to the nature of the change, method="change_in_mean"
is unable to detect any
change points at all:
In [7]: changeforest(X, "change_in_mean", "bs")
Out[7]:
best_split max_gain p_value
(0, 600] 589 8.625
[1] M. Londschien, P. Bühlmann and S. Kovács (2023). "Random Forests for Change Point Detection" Journal of Machine Learning Research
FAQs
Random Forests for Change Point Detection
We found that changeforest demonstrated a healthy version release cadence and project activity because the last version was released less than a year ago. It has 1 open source maintainer collaborating on the project.
Did you know?
Socket for GitHub automatically highlights issues in each pull request and monitors the health of all your open source dependencies. Discover the contents of your packages and block harmful activity before you install or update your dependencies.
Security News
Research
The Socket Research Team breaks down a malicious wrapper package that uses obfuscation to harvest credentials and exfiltrate sensitive data.
Research
Security News
Attackers used a malicious npm package typosquatting a popular ESLint plugin to steal sensitive data, execute commands, and exploit developer systems.
Security News
The Ultralytics' PyPI Package was compromised four times in one weekend through GitHub Actions cache poisoning and failure to rotate previously compromised API tokens.