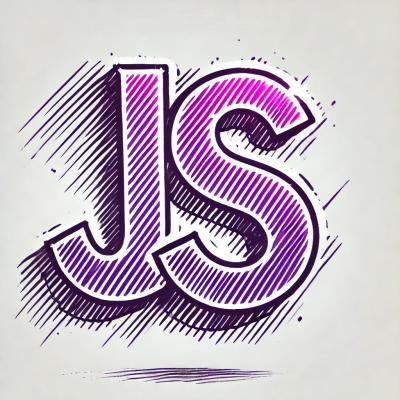
Security News
Oracle Drags Its Feet in the JavaScript Trademark Dispute
Oracle seeks to dismiss fraud claims in the JavaScript trademark dispute, delaying the case and avoiding questions about its right to the name.
A Python library for end-to-end A/B testing workflows, featuring:
import numpy as np
import pandas as pd
from cluster_experiments import PowerAnalysis, NormalPowerAnalysis
# Create sample data
N = 1_000
df = pd.DataFrame({
"target": np.random.normal(0, 1, size=N),
"date": pd.to_datetime(
np.random.randint(
pd.Timestamp("2024-01-01").value,
pd.Timestamp("2024-01-31").value,
size=N,
)
),
})
# Simulation-based power analysis with CUPED
config = {
"analysis": "ols",
"perturbator": "constant",
"splitter": "non_clustered",
"n_simulations": 50,
}
pw = PowerAnalysis.from_dict(config)
power = pw.power_analysis(df, average_effect=0.1)
# Normal approximation (faster)
npw = NormalPowerAnalysis.from_dict({
"analysis": "ols",
"splitter": "non_clustered",
"n_simulations": 5,
"time_col": "date",
})
power_normal = npw.power_analysis(df, average_effect=0.1)
power_line_normal = npw.power_line(df, average_effects=[0.1, 0.2, 0.3])
# MDE calculation
mde = npw.mde(df, power=0.8)
# MDE line with length
mde_timeline = npw.mde_time_line(
df,
powers=[0.8],
experiment_length=[7, 14, 21]
)
print(power, power_line_normal, power_normal, mde, mde_timeline)
import numpy as np
import pandas as pd
from cluster_experiments import AnalysisPlan
N = 1_000
experiment_data = pd.DataFrame({
"order_value": np.random.normal(100, 10, size=N),
"delivery_time": np.random.normal(10, 1, size=N),
"experiment_group": np.random.choice(["control", "treatment"], size=N),
"city": np.random.choice(["NYC", "LA"], size=N),
"customer_id": np.random.randint(1, 100, size=N),
"customer_age": np.random.randint(20, 60, size=N),
})
# Create analysis plan
plan = AnalysisPlan.from_metrics_dict({
"metrics": [
{"alias": "AOV", "name": "order_value"},
{"alias": "delivery_time", "name": "delivery_time"},
],
"variants": [
{"name": "control", "is_control": True},
{"name": "treatment", "is_control": False},
],
"variant_col": "experiment_group",
"alpha": 0.05,
"dimensions": [
{"name": "city", "values": ["NYC", "LA"]},
],
"analysis_type": "clustered_ols",
"analysis_config": {"cluster_cols": ["customer_id"]},
})
# Run analysis
print(plan.analyze(experiment_data).to_dataframe())
import numpy as np
import pandas as pd
from cluster_experiments import (
AnalysisPlan,
SimpleMetric,
Variant,
Dimension,
TargetAggregation,
HypothesisTest
)
N = 1000
experiment_data = pd.DataFrame({
"order_value": np.random.normal(100, 10, size=N),
"delivery_time": np.random.normal(10, 1, size=N),
"experiment_group": np.random.choice(["control", "treatment"], size=N),
"city": np.random.choice(["NYC", "LA"], size=N),
"customer_id": np.random.randint(1, 100, size=N),
"customer_age": np.random.randint(20, 60, size=N),
})
pre_experiment_data = pd.DataFrame({
"order_value": np.random.normal(100, 10, size=N),
"customer_id": np.random.randint(1, 100, size=N),
})
# Define test
cupac_model = TargetAggregation(
agg_col="customer_id",
target_col="order_value"
)
hypothesis_test = HypothesisTest(
metric=SimpleMetric(alias="AOV", name="order_value"),
analysis_type="clustered_ols",
analysis_config={
"cluster_cols": ["customer_id"],
"covariates": ["customer_age", "estimate_order_value"],
},
cupac_config={
"cupac_model": cupac_model,
"target_col": "order_value",
},
)
# Create analysis plan
plan = AnalysisPlan(
tests=[hypothesis_test],
variants=[
Variant("control", is_control=True),
Variant("treatment", is_control=False),
],
variant_col="experiment_group",
)
# Run analysis
results = plan.analyze(experiment_data, pre_experiment_data)
print(results.to_dataframe())
You can install this package via pip
.
pip install cluster-experiments
For detailed documentation and examples, visit our documentation site.
The library offers the following classes:
PowerAnalysis
: to run power analysis on any experiment design, using simulationPowerAnalysisWithPreExperimentData
: to run power analysis on a clustered/switchback design, but adding pre-experiment df during split and perturbation (especially useful for Synthetic Control)NormalPowerAnalysis
: to run power analysis on any experiment design using the central limit theorem for the distribution of the estimator. It can be used to compute the minimum detectable effect (MDE) for a given power level.ConstantPerturbator
: to artificially perturb treated group with constant perturbationsBinaryPerturbator
: to artificially perturb treated group for binary outcomesRelativePositivePerturbator
: to artificially perturb treated group with relative positive perturbationsRelativeMixedPerturbator
: to artificially perturb treated group with relative perturbations for positive and negative targetsNormalPerturbator
: to artificially perturb treated group with normal distribution perturbationsBetaRelativePositivePerturbator
: to artificially perturb treated group with relative positive beta distribution perturbationsBetaRelativePerturbator
: to artificially perturb treated group with relative beta distribution perturbations in a specified support intervalSegmentedBetaRelativePerturbator
: to artificially perturb treated group with relative beta distribution perturbations in a specified support interval, but using clustersClusteredSplitter
: to split data based on clustersFixedSizeClusteredSplitter
: to split data based on clusters with a fixed size (example: only 1 treatment cluster and the rest in control)BalancedClusteredSplitter
: to split data based on clusters in a balanced wayNonClusteredSplitter
: Regular data splitting, no clustersStratifiedClusteredSplitter
: to split based on clusters and strata, balancing the number of clusters in each stratusRepeatedSampler
: for backtests where we have access to counterfactuals, does not split the data, just duplicates the data for all groupsSwitchbackSplitter
: to split data based on clusters and dates, for switchback experimentsBalancedSwitchbackSplitter
: to split data based on clusters and dates, for switchback experiments, balancing treatment and control among all clustersStratifiedSwitchbackSplitter
: to split data based on clusters and dates, for switchback experiments, balancing the number of clusters in each stratusEmptyWashover
: no washover done at all.ConstantWashover
: accepts a timedelta parameter and removes the data when we switch from A to B for the timedelta interval.GeeExperimentAnalysis
: to run GEE analysis on the results of a clustered designMLMExperimentAnalysis
: to run Mixed Linear Model analysis on the results of a clustered designTTestClusteredAnalysis
: to run a t-test on aggregated data for clustersPairedTTestClusteredAnalysis
: to run a paired t-test on aggregated data for clustersClusteredOLSAnalysis
: to run OLS analysis on the results of a clustered designOLSAnalysis
: to run OLS analysis for non-clustered dataDeltaMethodAnalysis
: to run Delta Method Analysis for clustered designsTargetAggregation
: to add pre-experimental data of the outcome to reduce varianceSyntheticControlAnalysis
: to run synthetic control analysisMetric
: abstract class to define a metric to be used in the analysisSimpleMetric
: to create a metric defined at the same level of the data used for the analysisRatioMetric
: to create a metric defined at a lower level than the data used for the analysisVariant
: to define a variant of the experimentDimension
: to define a dimension to slice the results of the experimentHypothesisTest
: to define a Hypothesis Test with a metric, analysis method, optional analysis configuration, and optional dimensionsAnalysisPlan
: to define a plan of analysis with a list of Hypothesis Tests for a dataset and the experiment variants. The analyze()
method runs the analysis and returns the resultsAnalysisResults
: to store the results of the analysisPowerConfig
: to conveniently configure PowerAnalysis
classConfidenceInterval
: to store the data representation of a confidence intervalInferenceResults
: to store the structure of complete statistical analysis resultsFAQs
Unknown package
We found that cluster-experiments demonstrated a healthy version release cadence and project activity because the last version was released less than a year ago. It has 1 open source maintainer collaborating on the project.
Did you know?
Socket for GitHub automatically highlights issues in each pull request and monitors the health of all your open source dependencies. Discover the contents of your packages and block harmful activity before you install or update your dependencies.
Security News
Oracle seeks to dismiss fraud claims in the JavaScript trademark dispute, delaying the case and avoiding questions about its right to the name.
Security News
The Linux Foundation is warning open source developers that compliance with global sanctions is mandatory, highlighting legal risks and restrictions on contributions.
Security News
Maven Central now validates Sigstore signatures, making it easier for developers to verify the provenance of Java packages.