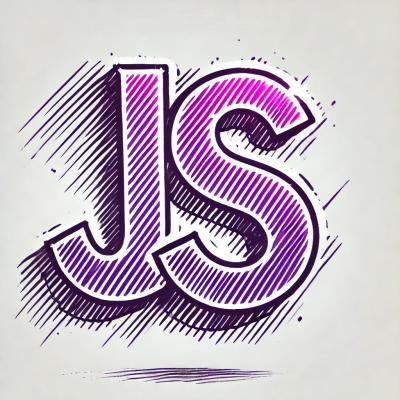
Security News
Oracle Drags Its Feet in the JavaScript Trademark Dispute
Oracle seeks to dismiss fraud claims in the JavaScript trademark dispute, delaying the case and avoiding questions about its right to the name.
A Python library for simplifying the evaluation of conformal predictors.
Installation of Conformal-eval is made by;
pip install conformal-eval
or including the extras report
:
pip install conformal-eval[report]
# or, depending on your shell you might need:
pip install "conformal-eval[report]"
Examples of using the package can be seen in our examples notebooks:
Package dependencies can be found in the pyproject.toml under [tool.poetry.dependencies]
, noting that the Jinja2
package is only required for for the report
extras (installing conformal-eval[report]
).
The code internally use numpy ndarrays for matrices and vectors, but tries to be agnostic about input being either list, arrays or Pandas equivalents. For performance reasons it is recommended that conversion to numpy format is done when using several of the methods in this library, as this is internally made in most functions.
For regression we require predictions to be the same as used in nonconformist, using 2D or 3D tensors in numpy ndarrays of shape (num_examples,2)
or (num_examples,2,num_significance_levels)
, where the second dimension contains the lower and upper limits of the prediction intervals.
Plotting is made based on the matplotlib library and Seaborn for the function plot_confusion_matrix_heatmap
. To easily set a nicer plotting style (in our opinion) you can use the function conf_eval.plot.update_plot_settings
which uses Seaborn to configure the default settings in matplotlib and e.g. easily scales font size.
conf_eval.cpsign
moduleThis module and submodules are intended for easily loading results from CPSign in the format required by Conformal-eval
report
This extras include the functionality to generate a "report" in HTML format for a model generated by CPSign. This report is at this point only in beta testing and contains rudimentary information - this could be altered in the future. Further note that this will install a CLI script called cpsign-report
once installing the conformal-eval[report]
package, to see the usage of this simply run cpsign-report --help
in your terminal environment.
All python-tests are located in the tests folder and are meant to be run using pytest. Test should be started from standing in the python
folder and can be run "all at once" (python -m pytest
), "per file" (python -m pytest tests/conf_eval/metrics/clf_metrics_test.py
), or a single test function (python -m pytest tests/conf_eval/metrics/clf_metrics_test.py::TestConfusionMatrix::test_with_custom_labels
).
python -m pytest [opt args]
is preferred here as the current directory is added to the python path and resolves the application code automatically. Simply running pytest
requires manual setup of the PYTHONPATH
instead.For the report
module there are CLI tests that require the package to be installed before running them (otherwise the cpsign-report
program is not available, or not updated). To do this you should use the following;
# Set up an venv to run in
poetry shell
# Install dependencies from the pyproject.toml
poetry install
# Run all (or subset) of tests
python -m pytest
Add/finish the following plots:
cpsign-report
utility (gitignore had removed them before).conf_eval.cpsign.report
module for generating model-reports in HTML format. The extras report
is needed to run this code.skip_inf
) when loading regression predictions and results, where a too high confidence level leads to infinitely large prediction intervals. This simply filters out the rows that have infinitely large outputs.cpsign.load_conf_independent_metrics
).pharmbio.cpsign
package with loading functionality for CPSign generated files, loading calibration statistics, efficiency statistics and predictions.plotting.plot_calibration
acting on pre-computed values or for classification using plotting.plot_calibration_clf
where true labels and p-values can be given.plotting.update_plot_settings
which updates the matplotlib global settings. Note this will have effect on all other plots generated in the same python session if those rely on matplotlib.FAQs
A package with utility functions for evaluating conformal predictors
We found that conformal-eval demonstrated a healthy version release cadence and project activity because the last version was released less than a year ago. It has 1 open source maintainer collaborating on the project.
Did you know?
Socket for GitHub automatically highlights issues in each pull request and monitors the health of all your open source dependencies. Discover the contents of your packages and block harmful activity before you install or update your dependencies.
Security News
Oracle seeks to dismiss fraud claims in the JavaScript trademark dispute, delaying the case and avoiding questions about its right to the name.
Security News
The Linux Foundation is warning open source developers that compliance with global sanctions is mandatory, highlighting legal risks and restrictions on contributions.
Security News
Maven Central now validates Sigstore signatures, making it easier for developers to verify the provenance of Java packages.