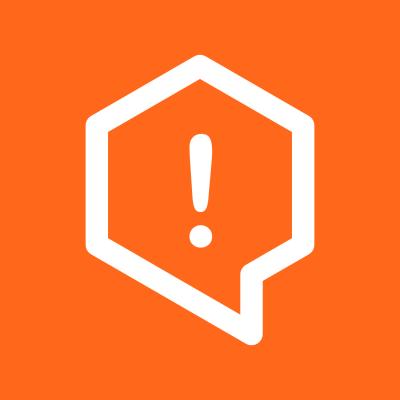
Security News
Fluent Assertions Faces Backlash After Abandoning Open Source Licensing
Fluent Assertions is facing backlash after dropping the Apache license for a commercial model, leaving users blindsided and questioning contributor rights.
Python bindings for the Transformer models implemented in C/C++ using GGML library.
Python bindings for the Transformer models implemented in C/C++ using GGML library.
Also see ChatDocs
Models | Model Type | CUDA | Metal |
---|---|---|---|
GPT-2 | gpt2 | ||
GPT-J, GPT4All-J | gptj | ||
GPT-NeoX, StableLM | gpt_neox | ||
Falcon | falcon | ✅ | |
LLaMA, LLaMA 2 | llama | ✅ | ✅ |
MPT | mpt | ✅ | |
StarCoder, StarChat | gpt_bigcode | ✅ | |
Dolly V2 | dolly-v2 | ||
Replit | replit |
pip install ctransformers
It provides a unified interface for all models:
from ctransformers import AutoModelForCausalLM
llm = AutoModelForCausalLM.from_pretrained("/path/to/ggml-model.bin", model_type="gpt2")
print(llm("AI is going to"))
To stream the output, set stream=True
:
for text in llm("AI is going to", stream=True):
print(text, end="", flush=True)
You can load models from Hugging Face Hub directly:
llm = AutoModelForCausalLM.from_pretrained("marella/gpt-2-ggml")
If a model repo has multiple model files (.bin
or .gguf
files), specify a model file using:
llm = AutoModelForCausalLM.from_pretrained("marella/gpt-2-ggml", model_file="ggml-model.bin")
Note: This is an experimental feature and may change in the future.
To use it with 🤗 Transformers, create model and tokenizer using:
from ctransformers import AutoModelForCausalLM, AutoTokenizer
model = AutoModelForCausalLM.from_pretrained("marella/gpt-2-ggml", hf=True)
tokenizer = AutoTokenizer.from_pretrained(model)
You can use 🤗 Transformers text generation pipeline:
from transformers import pipeline
pipe = pipeline("text-generation", model=model, tokenizer=tokenizer)
print(pipe("AI is going to", max_new_tokens=256))
You can use 🤗 Transformers generation parameters:
pipe("AI is going to", max_new_tokens=256, do_sample=True, temperature=0.8, repetition_penalty=1.1)
You can use 🤗 Transformers tokenizers:
from ctransformers import AutoModelForCausalLM
from transformers import AutoTokenizer
model = AutoModelForCausalLM.from_pretrained("marella/gpt-2-ggml", hf=True) # Load model from GGML model repo.
tokenizer = AutoTokenizer.from_pretrained("gpt2") # Load tokenizer from original model repo.
It is integrated into LangChain. See LangChain docs.
To run some of the model layers on GPU, set the gpu_layers
parameter:
llm = AutoModelForCausalLM.from_pretrained("TheBloke/Llama-2-7B-GGML", gpu_layers=50)
Install CUDA libraries using:
pip install ctransformers[cuda]
To enable ROCm support, install the ctransformers
package using:
CT_HIPBLAS=1 pip install ctransformers --no-binary ctransformers
To enable Metal support, install the ctransformers
package using:
CT_METAL=1 pip install ctransformers --no-binary ctransformers
Note: This is an experimental feature and only LLaMA models are supported using ExLlama.
Install additional dependencies using:
pip install ctransformers[gptq]
Load a GPTQ model using:
llm = AutoModelForCausalLM.from_pretrained("TheBloke/Llama-2-7B-GPTQ")
If model name or path doesn't contain the word
gptq
then specifymodel_type="gptq"
.
It can also be used with LangChain. Low-level APIs are not fully supported.
Parameter | Type | Description | Default |
---|---|---|---|
top_k | int | The top-k value to use for sampling. | 40 |
top_p | float | The top-p value to use for sampling. | 0.95 |
temperature | float | The temperature to use for sampling. | 0.8 |
repetition_penalty | float | The repetition penalty to use for sampling. | 1.1 |
last_n_tokens | int | The number of last tokens to use for repetition penalty. | 64 |
seed | int | The seed value to use for sampling tokens. | -1 |
max_new_tokens | int | The maximum number of new tokens to generate. | 256 |
stop | List[str] | A list of sequences to stop generation when encountered. | None |
stream | bool | Whether to stream the generated text. | False |
reset | bool | Whether to reset the model state before generating text. | True |
batch_size | int | The batch size to use for evaluating tokens in a single prompt. | 8 |
threads | int | The number of threads to use for evaluating tokens. | -1 |
context_length | int | The maximum context length to use. | -1 |
gpu_layers | int | The number of layers to run on GPU. | 0 |
Note: Currently only LLaMA, MPT and Falcon models support the
context_length
parameter.
AutoModelForCausalLM
AutoModelForCausalLM.from_pretrained
from_pretrained(
model_path_or_repo_id: str,
model_type: Optional[str] = None,
model_file: Optional[str] = None,
config: Optional[ctransformers.hub.AutoConfig] = None,
lib: Optional[str] = None,
local_files_only: bool = False,
revision: Optional[str] = None,
hf: bool = False,
**kwargs
) → LLM
Loads the language model from a local file or remote repo.
Args:
model_path_or_repo_id
: The path to a model file or directory or the name of a Hugging Face Hub model repo.model_type
: The model type.model_file
: The name of the model file in repo or directory.config
: AutoConfig
object.lib
: The path to a shared library or one of avx2
, avx
, basic
.local_files_only
: Whether or not to only look at local files (i.e., do not try to download the model).revision
: The specific model version to use. It can be a branch name, a tag name, or a commit id.hf
: Whether to create a Hugging Face Transformers model.Returns:
LLM
object.
LLM
LLM.__init__
__init__(
model_path: str,
model_type: Optional[str] = None,
config: Optional[ctransformers.llm.Config] = None,
lib: Optional[str] = None
)
Loads the language model from a local file.
Args:
model_path
: The path to a model file.model_type
: The model type.config
: Config
object.lib
: The path to a shared library or one of avx2
, avx
, basic
.The beginning-of-sequence token.
The config object.
The context length of model.
The input embeddings.
The end-of-sequence token.
The unnormalized log probabilities.
The path to the model file.
The model type.
The padding token.
The number of tokens in vocabulary.
LLM.detokenize
detokenize(tokens: Sequence[int], decode: bool = True) → Union[str, bytes]
Converts a list of tokens to text.
Args:
tokens
: The list of tokens.decode
: Whether to decode the text as UTF-8 string.Returns: The combined text of all tokens.
LLM.embed
embed(
input: Union[str, Sequence[int]],
batch_size: Optional[int] = None,
threads: Optional[int] = None
) → List[float]
Computes embeddings for a text or list of tokens.
Note: Currently only LLaMA and Falcon models support embeddings.
Args:
input
: The input text or list of tokens to get embeddings for.batch_size
: The batch size to use for evaluating tokens in a single prompt. Default: 8
threads
: The number of threads to use for evaluating tokens. Default: -1
Returns: The input embeddings.
LLM.eval
eval(
tokens: Sequence[int],
batch_size: Optional[int] = None,
threads: Optional[int] = None
) → None
Evaluates a list of tokens.
Args:
tokens
: The list of tokens to evaluate.batch_size
: The batch size to use for evaluating tokens in a single prompt. Default: 8
threads
: The number of threads to use for evaluating tokens. Default: -1
LLM.generate
generate(
tokens: Sequence[int],
top_k: Optional[int] = None,
top_p: Optional[float] = None,
temperature: Optional[float] = None,
repetition_penalty: Optional[float] = None,
last_n_tokens: Optional[int] = None,
seed: Optional[int] = None,
batch_size: Optional[int] = None,
threads: Optional[int] = None,
reset: Optional[bool] = None
) → Generator[int, NoneType, NoneType]
Generates new tokens from a list of tokens.
Args:
tokens
: The list of tokens to generate tokens from.top_k
: The top-k value to use for sampling. Default: 40
top_p
: The top-p value to use for sampling. Default: 0.95
temperature
: The temperature to use for sampling. Default: 0.8
repetition_penalty
: The repetition penalty to use for sampling. Default: 1.1
last_n_tokens
: The number of last tokens to use for repetition penalty. Default: 64
seed
: The seed value to use for sampling tokens. Default: -1
batch_size
: The batch size to use for evaluating tokens in a single prompt. Default: 8
threads
: The number of threads to use for evaluating tokens. Default: -1
reset
: Whether to reset the model state before generating text. Default: True
Returns: The generated tokens.
LLM.is_eos_token
is_eos_token(token: int) → bool
Checks if a token is an end-of-sequence token.
Args:
token
: The token to check.Returns:
True
if the token is an end-of-sequence token else False
.
LLM.prepare_inputs_for_generation
prepare_inputs_for_generation(
tokens: Sequence[int],
reset: Optional[bool] = None
) → Sequence[int]
Removes input tokens that are evaluated in the past and updates the LLM context.
Args:
tokens
: The list of input tokens.reset
: Whether to reset the model state before generating text. Default: True
Returns: The list of tokens to evaluate.
LLM.reset
reset() → None
Deprecated since 0.2.27.
LLM.sample
sample(
top_k: Optional[int] = None,
top_p: Optional[float] = None,
temperature: Optional[float] = None,
repetition_penalty: Optional[float] = None,
last_n_tokens: Optional[int] = None,
seed: Optional[int] = None
) → int
Samples a token from the model.
Args:
top_k
: The top-k value to use for sampling. Default: 40
top_p
: The top-p value to use for sampling. Default: 0.95
temperature
: The temperature to use for sampling. Default: 0.8
repetition_penalty
: The repetition penalty to use for sampling. Default: 1.1
last_n_tokens
: The number of last tokens to use for repetition penalty. Default: 64
seed
: The seed value to use for sampling tokens. Default: -1
Returns: The sampled token.
LLM.tokenize
tokenize(text: str, add_bos_token: Optional[bool] = None) → List[int]
Converts a text into list of tokens.
Args:
text
: The text to tokenize.add_bos_token
: Whether to add the beginning-of-sequence token.Returns: The list of tokens.
LLM.__call__
__call__(
prompt: str,
max_new_tokens: Optional[int] = None,
top_k: Optional[int] = None,
top_p: Optional[float] = None,
temperature: Optional[float] = None,
repetition_penalty: Optional[float] = None,
last_n_tokens: Optional[int] = None,
seed: Optional[int] = None,
batch_size: Optional[int] = None,
threads: Optional[int] = None,
stop: Optional[Sequence[str]] = None,
stream: Optional[bool] = None,
reset: Optional[bool] = None
) → Union[str, Generator[str, NoneType, NoneType]]
Generates text from a prompt.
Args:
prompt
: The prompt to generate text from.max_new_tokens
: The maximum number of new tokens to generate. Default: 256
top_k
: The top-k value to use for sampling. Default: 40
top_p
: The top-p value to use for sampling. Default: 0.95
temperature
: The temperature to use for sampling. Default: 0.8
repetition_penalty
: The repetition penalty to use for sampling. Default: 1.1
last_n_tokens
: The number of last tokens to use for repetition penalty. Default: 64
seed
: The seed value to use for sampling tokens. Default: -1
batch_size
: The batch size to use for evaluating tokens in a single prompt. Default: 8
threads
: The number of threads to use for evaluating tokens. Default: -1
stop
: A list of sequences to stop generation when encountered. Default: None
stream
: Whether to stream the generated text. Default: False
reset
: Whether to reset the model state before generating text. Default: True
Returns: The generated text.
FAQs
Python bindings for the Transformer models implemented in C/C++ using GGML library.
We found that ctransformers demonstrated a healthy version release cadence and project activity because the last version was released less than a year ago. It has 1 open source maintainer collaborating on the project.
Did you know?
Socket for GitHub automatically highlights issues in each pull request and monitors the health of all your open source dependencies. Discover the contents of your packages and block harmful activity before you install or update your dependencies.
Security News
Fluent Assertions is facing backlash after dropping the Apache license for a commercial model, leaving users blindsided and questioning contributor rights.
Research
Security News
Socket researchers uncover the risks of a malicious Python package targeting Discord developers.
Security News
The UK is proposing a bold ban on ransomware payments by public entities to disrupt cybercrime, protect critical services, and lead global cybersecurity efforts.