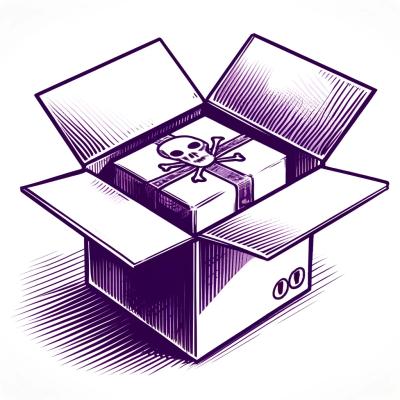
Security News
Research
Data Theft Repackaged: A Case Study in Malicious Wrapper Packages on npm
The Socket Research Team breaks down a malicious wrapper package that uses obfuscation to harvest credentials and exfiltrate sensitive data.
dataclasses-avroschema
Advanced tools
Generate Avro Schemas from Python classes. Serialize/Deserialize python instances with avro schemas
Generate avro schemas from python dataclasses, Pydantic models and Faust Records. Code generation from avro schemas. Serialize/Deserialize python instances with avro schemas
python 3.9+
with pip
or poetry
:
pip install dataclasses-avroschema
or poetry add dataclasses-avroschema
pip install 'dataclasses-avroschema[pydantic]'
or poetry add dataclasses-avroschema --extras "pydantic"
pip install 'dataclasses-avroschema[faust]'
or poetry add dataclasses-avroschema --extras "faust"
pip install 'dataclasses-avroschema[faker]'
or poetry add dataclasses-avroschema --extras "faker"
pip install 'dataclasses-avroschema[cli]'
or poetry add dataclasses-avroschema --with cli
Note: You can install all extra dependencies with pip install dataclasses-avroschema[faust,pydantic,faker,cli]
or poetry add dataclasses-avroschema --extras "pydantic faust faker cli"
https://marcosschroh.github.io/dataclasses-avroschema/
from dataclasses import dataclass
import enum
import typing
from dataclasses_avroschema import AvroModel
class FavoriteColor(str, enum.Enum):
BLUE = "BLUE"
YELLOW = "YELLOW"
GREEN = "GREEN"
@dataclass
class User(AvroModel):
"An User"
name: str
age: int
pets: typing.List[str]
accounts: typing.Dict[str, int]
favorite_colors: FavoriteColor
country: str = "Argentina"
address: typing.Optional[str] = None
class Meta:
namespace = "User.v1"
aliases = ["user-v1", "super user"]
print(User.avro_schema())
# {
# "type": "record",
# "name": "User",
# "fields": [
# {"name": "name", "type": "string"},
# {"name": "age", "type": "long"},
# {"name": "pets", "type": {"type": "array", "items": "string", "name": "pet"}},
# {"name": "accounts", "type": {"type": "map", "values": "long", "name": "account"}},
# {"name": "favorite_colors", "type": {"type": "enum", "name": "FavoriteColor", "symbols": ["BLUE", "YELLOW", "GREEN"]}},
# {"name": "country", "type": "string", "default": "Argentina"},
# {"name": "address", "type": ["null", "string"], "default": null}
# ],
# "doc": "An User",
# "namespace": "User.v1",
# "aliases": ["user-v1", "super user"]
# }
assert User.avro_schema_to_python() == {
"type": "record",
"name": "User",
"doc": "An User",
"namespace": "User.v1",
"aliases": ["user-v1", "super user"],
"fields": [
{"name": "name", "type": "string"},
{"name": "age", "type": "long"},
{"name": "pets", "type": {"type": "array", "items": "string", "name": "pet"}},
{"name": "accounts", "type": {"type": "map", "values": "long", "name": "account"}},
{"name": "favorite_colors", "type": {"type": "enum", "name": "FavoriteColor", "symbols": ["BLUE", "YELLOW", "GREEN"]}},
{"name": "country", "type": "string", "default": "Argentina"},
{"name": "address", "type": ["null", "string"], "default": None}
],
}
For serialization is neccesary to use python class/dataclasses instance
from dataclasses import dataclass
import typing
from dataclasses_avroschema import AvroModel
@dataclass
class Address(AvroModel):
"An Address"
street: str
street_number: int
@dataclass
class User(AvroModel):
"User with multiple Address"
name: str
age: int
addresses: typing.List[Address]
address_data = {
"street": "test",
"street_number": 10,
}
# create an Address instance
address = Address(**address_data)
data_user = {
"name": "john",
"age": 20,
"addresses": [address],
}
# create an User instance
user = User(**data_user)
# serialization
assert user.serialize() == b"\x08john(\x02\x08test\x14\x00"
assert user.serialize(
serialization_type="avro-json"
) == b'{"name": "john", "age": 20, "addresses": [{"street": "test", "street_number": 10}]}'
# # Get the json from the instance
assert user.to_json() == '{"name": "john", "age": 20, "addresses": [{"street": "test", "street_number": 10}]}'
# # Get a python dict
assert user.to_dict() == {
"name": "john",
"age": 20,
"addresses": [
{"street": "test", "street_number": 10}
]
}
Deserialization could take place with an instance dataclass or the dataclass itself. Can return the dict representation or a new class instance
import typing
import dataclasses
from dataclasses_avroschema import AvroModel
@dataclasses.dataclass
class Address(AvroModel):
"An Address"
street: str
street_number: int
@dataclasses.dataclass
class User(AvroModel):
"User with multiple Address"
name: str
age: int
addresses: typing.List[Address]
avro_binary = b"\x08john(\x02\x08test\x14\x00"
avro_json_binary = b'{"name": "john", "age": 20, "addresses": [{"street": "test", "street_number": 10}]}'
# return a new class instance!!
assert User.deserialize(avro_binary) == User(
name='john',
age=20,
addresses=[Address(street='test', street_number=10)]
)
# return a python dict
assert User.deserialize(avro_binary, create_instance=False) == {
"name": "john",
"age": 20,
"addresses": [
{"street": "test", "street_number": 10}
]
}
# return a new class instance!!
assert User.deserialize(avro_json_binary, serialization_type="avro-json") == User(
name='john',
age=20,
addresses=[Address(street='test', street_number=10)]
)
# return a python dict
assert User.deserialize(
avro_json_binary,
serialization_type="avro-json",
create_instance=False
) == {"name": "john", "age": 20, "addresses": [{"street": "test", "street_number": 10}]}
To add dataclasses-avroschema
functionality to pydantic
you only need to replace BaseModel
by AvroBaseModel
:
import typing
import enum
from dataclasses_avroschema.pydantic import AvroBaseModel
from pydantic import Field, ValidationError
class FavoriteColor(str, enum.Enum):
BLUE = "BLUE"
YELLOW = "YELLOW"
GREEN = "GREEN"
class UserAdvance(AvroBaseModel):
name: str
age: int
pets: typing.List[str] = Field(default_factory=lambda: ["dog", "cat"])
accounts: typing.Dict[str, int] = Field(default_factory=lambda: {"key": 1})
has_car: bool = False
favorite_colors: FavoriteColor = FavoriteColor.BLUE
country: str = "Argentina"
address: typing.Optional[str] = None
class Meta:
schema_doc = False
assert UserAdvance.avro_schema_to_python() == {
"type": "record",
"name": "UserAdvance",
"fields": [
{"name": "name", "type": "string"},
{"name": "age", "type": "long"},
{"name": "pets", "type": {"type": "array", "items": "string", "name": "pet"}, "default": ["dog", "cat"]},
{"name": "accounts", "type": {"type": "map", "values": "long", "name": "account"}, "default": {"key": 1}},
{"name": "has_car", "type": "boolean", "default": False},{"name": "favorite_colors", "type": {"type": "enum", "name": "FavoriteColor", "symbols": ["BLUE", "YELLOW", "GREEN"]}, "default": "BLUE"},
{"name": "country", "type": "string", "default": "Argentina"}, {"name": "address", "type": ["null", "string"], "default": None}
]
}
print(UserAdvance.json_schema())
# {
# "$defs": {"FavoriteColor": {"enum": ["BLUE", "YELLOW", "GREEN"], "title": "FavoriteColor", "type": "string"}},
# "properties": {
# "name": {"title": "Name", "type": "string"},
# "age": {"title": "Age", "type": "integer"},
# "pets": {"items": {"type": "string"}, "title": "Pets", "type": "array"},
# "accounts": {"additionalProperties": {"type": "integer"}, "title": "Accounts", "type": "object"},
# "has_car": {"default": false, "title": "Has Car", "type": "boolean"},
# "favorite_colors": {"allOf": [{"$ref": "#/$defs/FavoriteColor"}], "default": "BLUE"},
# "country": {"default": "Argentina", "title": "Country", "type": "string"},
# "address": {"anyOf": [{"type": "string"}, {"type": "null"}], "default": null, "title": "Address"}
# },
# "required": ["name", "age"],
# "title": "UserAdvance",
# "type": "object"
# }"""
user = UserAdvance(name="bond", age=50)
# pydantic
assert user.dict() == {
'name': 'bond',
'age': 50,
'pets': ['dog', 'cat'],
'accounts': {'key': 1},
'has_car': False,
'favorite_colors': FavoriteColor.BLUE,
'country': 'Argentina',
'address': None
}
# pydantic
print(user.json())
assert user.json() == '{"name":"bond","age":50,"pets":["dog","cat"],"accounts":{"key":1},"has_car":false,"favorite_colors":"BLUE","country":"Argentina","address":null}'
# pydantic
try:
user = UserAdvance(name="bond")
except ValidationError as exc:
...
# dataclasses-avroschema
event = user.serialize()
assert event == b'\x08bondd\x04\x06dog\x06cat\x00\x02\x06key\x02\x00\x00\x00\x12Argentina\x00'
assert UserAdvance.deserialize(data=event) == UserAdvance(
name='bond',
age=50,
pets=['dog', 'cat'],
accounts={'key': 1},
has_car=False,
favorite_colors=FavoriteColor.BLUE,
country='Argentina',
address=None
)
Under examples folder you can find 3 differents kafka examples, one with aiokafka (async
) showing the simplest use case when a AvroModel
instance is serialized and sent it thorught kafka, and the event is consumed.
The other two examples are sync
using the kafka-python driver, where the avro-json
serialization and schema evolution
(FULL
compatibility) is shown.
Also, there are two redis
examples using redis streams
with walrus and redisgears-py
Dataclasses Avro Schema also includes a factory
feature, so you can generate fast
python instances and use them, for example, to test your data streaming pipelines. Instances can be generated using the fake
method.
Note: This feature is not enabled by default and requires you have the faker
extra installed. You may install it with pip install 'dataclasses-avroschema[faker]'
import typing
import dataclasses
from dataclasses_avroschema import AvroModel
@dataclasses.dataclass
class Address(AvroModel):
"An Address"
street: str
street_number: int
@dataclasses.dataclass
class User(AvroModel):
"User with multiple Address"
name: str
age: int
addresses: typing.List[Address]
Address.fake()
# >>>> Address(street='PxZJILDRgbXyhWrrPWxQ', street_number=2067)
User.fake()
# >>>> User(name='VGSBbOGfSGjkMDnefHIZ', age=8974, addresses=[Address(street='vNpPYgesiHUwwzGcmMiS', street_number=4790)])
typing.Annotated
supportedtyping.Literal
supportedfaust.Record
avro schema
generatedavsc
fileskafka
drivers: aiokafka, kafka-pythonredis
drivers: walrus and redisgears-pyPoetry is needed to install the dependencies and develope locally
poetry install --all-extras
./scripts/format
./scripts/test
./scripts/test-documentation
For commit messages we use commitizen in order to standardize a way of committing rules
FAQs
Generate Avro Schemas from Python classes. Serialize/Deserialize python instances with avro schemas
We found that dataclasses-avroschema demonstrated a healthy version release cadence and project activity because the last version was released less than a year ago. It has 1 open source maintainer collaborating on the project.
Did you know?
Socket for GitHub automatically highlights issues in each pull request and monitors the health of all your open source dependencies. Discover the contents of your packages and block harmful activity before you install or update your dependencies.
Security News
Research
The Socket Research Team breaks down a malicious wrapper package that uses obfuscation to harvest credentials and exfiltrate sensitive data.
Research
Security News
Attackers used a malicious npm package typosquatting a popular ESLint plugin to steal sensitive data, execute commands, and exploit developer systems.
Security News
The Ultralytics' PyPI Package was compromised four times in one weekend through GitHub Actions cache poisoning and failure to rotate previously compromised API tokens.