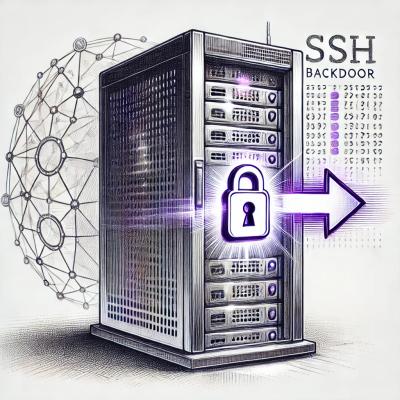
Research
Security News
Malicious npm Packages Inject SSH Backdoors via Typosquatted Libraries
Socket’s threat research team has detected six malicious npm packages typosquatting popular libraries to insert SSH backdoors.
differentiable-randaugment
Advanced tools
Optimize RandAugment with differentiable operations
Differentiable RandAugment is a differentiable version of RandAugment. The original paper proposed to find optimal parameters by using grid search. Instead, this library supports differentiable operations to calculate gradient of the magnitude parameter and optimize it. See getting started.
To install the latest version from PyPI:
$ pip install -U differentiable_randaugment
Or you can install from source by cloning the repository and running:
$ git clone https://github.com/affjljoo3581/Differentiable-RandAugment.git
$ cd Differentiable-RandAugment
$ python setup.py install
First, create RandAugmentModule
with your desired number of operations. This module is a differentiable and torch.Tensor
calculable version of RandAugment
policy. Using this module, you can train the policy as one of the neural-network model. Note that randomly selected num_ops
operations will be applied to the images.
from differentiable_randaugment import RandAugmentModule
augmentor = RandAugmentModule(num_ops=2)
Now you need to perform the module to the images. Usually augmentations are applied in Dataset
. That is, the operations use np.ndarray
images. However, it cannot calculate the gradients for image and magnitude parameter (because the entire optimization procedure is based on torch.Tensor
s). To resolve this, you should apply this module to torch.Tensor
images rather than np.ndarray
.
for inputs, labels in train_dataloader:
inputs = inputs.cuda()
logits = model(augmentor(inputs))
...
Of course, other augmentations should be removed from preprocessing:
transform = Compose([
Resize(...),
Normalize((0.5, 0.5, 0.5), (0.5, 0.5, 0.5)),
ToTensorV2(),
])
And lastly, create an optimizer with this module parameters. We recommend to use different learning rate for the model and the augmentor:
param_groups = [
{"params": augmentor.parameters(), "lr": 10 * learning_rate},
{"params": model.parameters(), "lr": learning_rate},
]
optimizer = optim.Adam(param_groups)
Now the RandAugment
policy will be trained with your prediction model.
After training RandAugmentModule
, get the trained optimal magnitude value by calling augmentor.get_magnitude()
and use the magnitude as follows:
from differentiable_randaugment import RandAugment
transform = Compose([
Resize(...),
RandAugment(num_ops=..., magnitude=...),
Normalize((0.5, 0.5, 0.5), (0.5, 0.5, 0.5)),
ToTensorV2(),
])
dataset = Dataset(..., transform=transform)
While RandAugment
is an extension of albumentations
, you can combine other augmentations in albumentations
with this class.
Differentiable RandAugment supports 14 operations described in the original paper. The below table shows the detailed differential specification of each operation.
Input Image | Magnitude | |
---|---|---|
Identity | ✔ | |
ShearX | ✔ | ✔ |
ShearY | ✔ | ✔ |
TranslateX | ✔ | ✔ |
TranslateY | ✔ | ✔ |
Rotate | ✔ | ✔ |
Cutout | ✔ | ✔ |
AutoContrast | ✔ | |
Equalize | ✔ | |
Solarize | ✔ | |
SolarizeAdd | ✔ | ✔ |
Posterize | ✔ | |
Contrast | ✔ | ✔ |
Color | ✔ | ✔ |
Brightness | ✔ | ✔ |
Sharpness | ✔ | ✔ |
Differentiable RandAugment is Apache-2.0 Licensed.
FAQs
Optimize RandAugment with differentiable operations
We found that differentiable-randaugment demonstrated a healthy version release cadence and project activity because the last version was released less than a year ago. It has 1 open source maintainer collaborating on the project.
Did you know?
Socket for GitHub automatically highlights issues in each pull request and monitors the health of all your open source dependencies. Discover the contents of your packages and block harmful activity before you install or update your dependencies.
Research
Security News
Socket’s threat research team has detected six malicious npm packages typosquatting popular libraries to insert SSH backdoors.
Security News
MITRE's 2024 CWE Top 25 highlights critical software vulnerabilities like XSS, SQL Injection, and CSRF, reflecting shifts due to a refined ranking methodology.
Security News
In this segment of the Risky Business podcast, Feross Aboukhadijeh and Patrick Gray discuss the challenges of tracking malware discovered in open source softare.