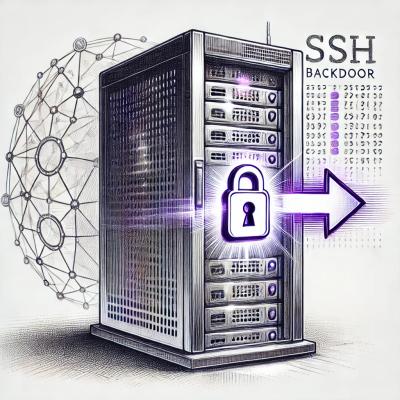
Research
Security News
Malicious npm Packages Inject SSH Backdoors via Typosquatted Libraries
Socket’s threat research team has detected six malicious npm packages typosquatting popular libraries to insert SSH backdoors.
Deep Learning Progress
A Python library for progress bars with the function of aggregating each iteration's value.
It helps manage the loss of each epoch in deep learning or machine learning training.
pip install dlprog
Setup
from dlprog import Progress
prog = Progress()
Example
import random
import time
n_epochs = 3
n_iter = 10
prog.start(n_epochs=n_epochs, n_iter=n_iter, label='value') # Initialize start time and epoch.
for _ in range(n_epochs):
for _ in range(n_iter):
time.sleep(0.1)
value = random.random()
prog.update(value) # Update progress bar and aggregate value.
1/3: ######################################## 100% [00:00:01.06] value: 0.64755
2/3: ######################################## 100% [00:00:01.05] value: 0.41097
3/3: ######################################## 100% [00:00:01.06] value: 0.26648
Get each epoch's value
>>> prog.values
[0.6475490908029968, 0.4109736504929395, 0.26648041702649705]
Call get_all_values()
method to get all values of each iteration.
And get_all_times()
method to get all times of each iteration.
Setup.
train_progress
function is a shortcut for Progress
class.
Return a progress bar that is suited for machine learning training.
from dlprog import train_progress
prog = train_progress()
Example. Case of training a deep learning model with PyTorch.
n_epochs = 3
n_iter = len(dataloader)
prog.start(n_epochs=n_epochs, n_iter=n_iter)
for _ in range(n_epochs):
for x, label in dataloader:
optimizer.zero_grad()
y = model(x)
loss = criterion(y, label)
loss.backward()
optimizer.step()
prog.update(loss.item())
Output
1/3: ######################################## 100% [00:00:03.08] loss: 0.34099
2/3: ######################################## 100% [00:00:03.12] loss: 0.15259
3/3: ######################################## 100% [00:00:03.14] loss: 0.10684
If you want to obtain weighted exact values considering batch size:
prog.update(loss.item(), weight=len(x))
Advanced arguments, functions, etc.
Also, see API Reference if you want to know more.
leave_freq
Argument that controls the frequency of leaving the progress bar.
n_epochs = 12
n_iter = 10
prog.start(n_epochs=n_epochs, n_iter=n_iter, leave_freq=4)
for _ in range(n_epochs):
for _ in range(n_iter):
time.sleep(0.1)
value = random.random()
prog.update(value)
Output
4/12: ######################################## 100% [00:00:01.06] loss: 0.34203
8/12: ######################################## 100% [00:00:01.05] loss: 0.47886
12/12: ######################################## 100% [00:00:01.05] loss: 0.40241
unit
Argument that multiple epochs as a unit.
n_epochs = 12
n_iter = 10
prog.start(n_epochs=n_epochs, n_iter=n_iter, unit=4)
for _ in range(n_epochs):
for _ in range(n_iter):
time.sleep(0.1)
value = random.random()
prog.update(value)
Output
1-4/12: ######################################## 100% [00:00:04.21] value: 0.49179
5-8/12: ######################################## 100% [00:00:04.20] value: 0.51518
9-12/12: ######################################## 100% [00:00:04.18] value: 0.54546
You can add a note to the progress bar.
n_iter = 10
prog.start(n_iter=n_iter, note='This is a note')
for _ in range(n_iter):
time.sleep(0.1)
value = random.random()
prog.update(value)
Output
1: ######################################## 100% [00:00:01.05] 0.58703, This is a note
You can also add a note when update()
as note
argument.
Also, you can add a note when end of epoch usin memo() if defer=True
.
n_epochs = 3
prog.start(
n_epochs=n_epochs,
n_iter=len(trainloader),
label='train_loss',
defer=True,
width=20,
)
for _ in range(n_epochs):
for x, label in trainloader:
optimizer.zero_grad()
y = model(x)
loss = criterion(y, label)
loss.backward()
optimizer.step()
prog.update(loss.item())
test_loss = eval_model(model)
prog.memo(f'test_loss: {test_loss:.5f}')
Output
1/3: #################### 100% [00:00:02.83] train_loss: 0.34094, test_loss: 0.18194
2/3: #################### 100% [00:00:02.70] train_loss: 0.15433, test_loss: 0.12987
3/3: #################### 100% [00:00:02.79] train_loss: 0.10651, test_loss: 0.09783
If you want to aggregate multiple values, set n_values
and input values as a list.
n_epochs = 3
n_iter = 10
prog.start(n_epochs=n_epochs, n_iter=n_iter, n_values=2)
for _ in range(n_epochs):
for _ in range(n_iter):
time.sleep(0.1)
value1 = random.random()
value2 = random.random() * 10
prog.update([value1, value2])
Output
1/3: ######################################## 100% [00:00:01.05] 0.47956, 4.96049
2/3: ######################################## 100% [00:00:01.05] 0.30275, 4.86003
3/3: ######################################## 100% [00:00:01.05] 0.43296, 3.31025
You can input multiple labels as a list instead of n_values
.
prog.start(n_iter=n_iter, label=['value1', 'value2'])
Progress
object keeps constructor arguments as default attributes.
These attributes are used when not specified in start()
.
Attributes specified in start()
is used preferentially while this running (until next start()
or reset()
).
If a required attribute (n_iter
) has already been specified, start()
can be skipped.
momentum
Update values by exponential moving average.
now_values = []
prog.start(n_iter=10, momentum=0.9, defer=True)
for i in range(10):
prog.update(i)
now_values.append(prog.now_values())
now_values
Output
1: ######################################## 100% [00:00:00.01] 3.48678
[0.0,
0.09999999999999998,
0.2899999999999999,
0.5609999999999999,
0.9048999999999999,
1.3144099999999999,
1.7829689999999998,
2.3046721,
2.8742048899999997,
3.4867844009999995]
Progress
class.train_progress
function.values
attribute.leave_freq
argument.unit
argument.note
argument, memo()
method, and defer
argument.round
argument.start()
.note=None
in memo()
.note=None
defaultly in memo()
.label
is not available when with_test=True
in train_progress()
.width
is not available when with_test=True
in train_progress()
.get_all_values()
method.get_all_times()
method.store_all_values
and store_all_times
arguments.momentum
argument.now_values()
method.FAQs
A progress bar that aggregates the values of each iteration.
We found that dlprog demonstrated a healthy version release cadence and project activity because the last version was released less than a year ago. It has 1 open source maintainer collaborating on the project.
Did you know?
Socket for GitHub automatically highlights issues in each pull request and monitors the health of all your open source dependencies. Discover the contents of your packages and block harmful activity before you install or update your dependencies.
Research
Security News
Socket’s threat research team has detected six malicious npm packages typosquatting popular libraries to insert SSH backdoors.
Security News
MITRE's 2024 CWE Top 25 highlights critical software vulnerabilities like XSS, SQL Injection, and CSRF, reflecting shifts due to a refined ranking methodology.
Security News
In this segment of the Risky Business podcast, Feross Aboukhadijeh and Patrick Gray discuss the challenges of tracking malware discovered in open source softare.