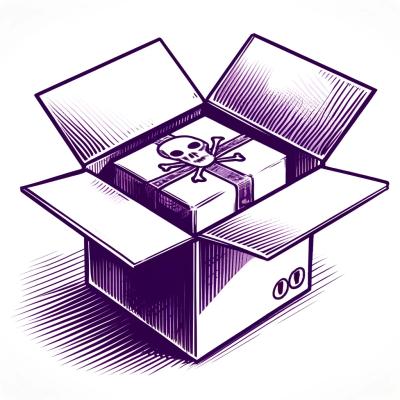
Security News
Research
Data Theft Repackaged: A Case Study in Malicious Wrapper Packages on npm
The Socket Research Team breaks down a malicious wrapper package that uses obfuscation to harvest credentials and exfiltrate sensitive data.
We'll be running distributed_optimizer.py
to start the optimization.
distributed_optimizer.py
can be run in 2 modes: host mode and client mode.
The host mode will call 2 processes: One that
starts the Optimizer
of choice and One that connect with other machines (the clients) to run distributed_optimizer.py
on client mode.
The client mode will run the user-specified MyWhateverTrainer.
Host mode: python3 distributed_optimizer.py
as host mode is the default.
Client mode: python3 distributed_optimizer.py --run_as=client
as client mode. You do not need to manually run client mode on each client machine as the host mode will do this. However, you can use it if you want to add client machines during optimization.
Essential parts of the script:
The COMMANDS: A constant dictionary. The keys are machine categories, and values are the necessary commands to run distributed_optimizer.py
on client mode on those different categories.
A MyWhateverTrainer that inherits the Trainer
abstract class from
src.trainer
, and implement the abstract method get_observation
, in which the set of hyperparameters (candidate) given will be plugged into the objective function.
A start_host()
function that will be used to call 2 processes: one that
start the Optimizer
and one that runs appropriate sequence of commands to run distributed_optimizer.py
on client mode, i.e. startTrainers
on respective machines using the following:
python3 distributed_optimizer.py --run_as=client
A start_client()
function that will run MyWhateverTrainer, i.e. run objective function.
A main()
that parse command line input and switch between host and client mode, and specify further information needed to run objective function on target machines.
Check list:
MyWhatever Trainer
that implements the get_observation
method.ssh [name]@[hostmachine] [YOUR COMMANDS]
COMMANDS
dictionary.distributed_optimizer.py
and related files on all of the machines you intend to useFAQs
Distributed Optimizer
We found that dopt demonstrated a healthy version release cadence and project activity because the last version was released less than a year ago. It has 1 open source maintainer collaborating on the project.
Did you know?
Socket for GitHub automatically highlights issues in each pull request and monitors the health of all your open source dependencies. Discover the contents of your packages and block harmful activity before you install or update your dependencies.
Security News
Research
The Socket Research Team breaks down a malicious wrapper package that uses obfuscation to harvest credentials and exfiltrate sensitive data.
Research
Security News
Attackers used a malicious npm package typosquatting a popular ESLint plugin to steal sensitive data, execute commands, and exploit developer systems.
Security News
The Ultralytics' PyPI Package was compromised four times in one weekend through GitHub Actions cache poisoning and failure to rotate previously compromised API tokens.