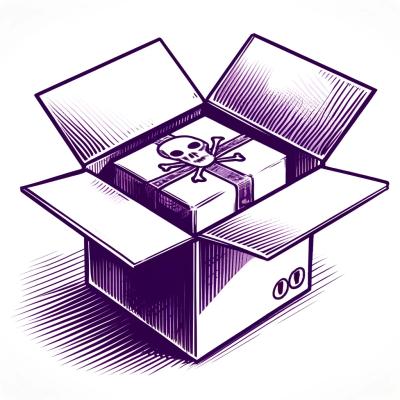
Security News
Research
Data Theft Repackaged: A Case Study in Malicious Wrapper Packages on npm
The Socket Research Team breaks down a malicious wrapper package that uses obfuscation to harvest credentials and exfiltrate sensitive data.
# normal install
pip install dslibrary
# to include a robust set of data connectors:
pip install dslibrary[all]
Data science code is supposed to focus on the data, but it frequently gets bogged down in repetitive tasks like juggling parameters, working out file formats, and connecting to cloud data sources. This library proposes some ways to make those parts of life a little easier, and to make the resulting code a little shorter and more readable.
Some of this project's goals:
If you use dslibrary with no configuration it will revert to very straightforward behaviors that a person would expect while doing local development. But it can be configured to operate in a wide range of environments.
Here's a simple data cleaning example. You can run it from the command line, or call it's clean() method and it will clip the values in a column of the supplied data. But so far it only works on local files, it only supports one file format (CSV), and it uses read_csv()'s default formatting arguments, which will not always work.
# clean_it.py
import pandas
def clean(upper=100, input="in.csv", output="out.csv"):
df = pandas.read_csv(input)
df.loc[df.x > upper, 'x'] = upper
df.to_csv(out)
if __name__ == "__main__":
# INSERT ARGUMENT PARSING CODE HERE
clean(...)
Here it is converted to use dslibrary. Now our code will work with any data format from any source. It still has a parameter 'upper' that can be set, it reads from a named input "in", and writes to a named output "out". And it is compatible with the prior version.
import dslibrary
def clean(upper=100, input="in", output="out"):
df = dslibrary.load_dataframe(input)
df.loc[df.x > upper, 'x'] = upper
dslibrary.write_resource(output, df)
if __name__ == "__main__":
clean(**dsl.get_parameters())
Now if we execute that code through dslibrary's ModelRunner class, we can point it to data in various places and set different file formatting options:
from dslibrary import ModelRunner
import clean_it
ModelRunner() \
.set_parameter("upper", 50) \
.set_input("in", "some_file.csv", format_options={"delimiter": "|") \
.set_output("out", "target.json", format_optons={"lines": True}) \
.run_method(clean_it.clean)
Or to the cloud:
from dslibrary import ModelRunner
import clean_it
ModelRunner() \
.set_parameter("upper", 50) \
.set_input("in", "s3://bucket/raw.csv", format_options={"delim_whitespace": True}, access_key=..., secret_key=...) \
.set_output("out", "s3://bucket/clipped.csv", format_options={"sep": "\t"}) \
.run_method(clean_it.clean)
Or I can invoke it as a subprocess:
.run_local("path/to/clean_it.py")
This will also work with notebooks:
.run_local("path/to/clean_it.ipynb")
Write code that can load files from a local folder, or an s3 bucket. Note that an input can be either a folder or a file. In this case we are pointing to a folder.
def my_code(dsl):
df = dsl.load_dataframe("data.csv")
msg = dsl.read_resource("msg.txt")
from dslibrary import ModelRunner
runner = ModelRunner()
# files from s3
runner.set_input("the_files", uri="s3://bucket", access_key=..., secret_key=...)
# or files from a local folder
runner.set_input("the_files", uri="/folder")
runner.run_method(my_code)
SQL can target a normal database engine, like MySQL, or it can target a folder containing (for instance) CSV files.
def my_model(dsl):
df = dsl.sql_select("select x from t1", engine="the_files")
runner = ModelRunner()
# tables in mysql
runner.set_input("the_files", uri="mysql://host/db", username=..., password=...)
# or tables in local files
runner.set_input("the_files", uri="/folder")
runner.run_method(my_model)
Report the average of some data:
import dslibrary as dsl
data = dsl.load_dataframe("input")
with dsl.start_run():
dsl.log_metric("avg_temp", data.temperature.mean())
Call it with some SQL data:
from dslibrary import ModelRunner
runner = ModelRunner()
runner.set_input(
"input",
uri="mysql://username:password@mysql-server/climate",
sql="select temperature from readings order by timestamp desc limit 100"
))
runner.run_local("avg_temp.py")
Change format & filename for metrics output (format is implied by filename):
runner.set_output(dslibrary.METRICS_ALIAS, "metrics.csv", format_optons={"sep": "\t"})
We could send the metrics to mlflow instead:
runner = ModelRunner(mlflow=True)
It can be annoying to have to switch between pandas and SQL depending on which type of data has been provided. So dslibrary provides reasonably robust SQL querying of data files.
Query files:
df = dslibrary.sql_select("SELECT x, y from `table1.csv` WHERE x < 100")
df.head()
Or data:
df = dslibrary.sql_select("SELECT * from my_table where x < 100", data={"table1": my_table})
Or connect to a named SQL engine:
runner = ModelRunner()
runner.set_input("sql1", uri="postgres://host/database", username="u", password="p")
...
df = dslibrary.sql_select("SELECT something", engine="sql1")
If all the essential connections to the outside from your code are 'abstracted' and can be repointed elsewhere, then your code will run everywhere.
The entire implementation of dslibrary can be changed through environment variables. In fact, all the ModelRunner class really does is set environment variables.
These are the main types of interface data science code has to the outside world. Dslibrary offers methods to manage all of these, and they can all be handled differently through configuration:
The normal way of accessing data in the cloud is to store CSP credentials in, say, "~/.aws/credentials", and then the intervening library is able to read and write to s3 buckets. You have to make sure this setup is done, that the right packages are in your environment, and write your code accordingly. Here are the main problems:
It can be time consuming to ensure that every system running the code has this credential configuration in place, and one system may need to access multiple accounts for the same CSP. And especially if you are on one CSP trying to access data in another CSP there is no automated setup you can count on.
The usual solution is to require that all the data science code add support for some particular cloud provider, and accept credentials as secrets. It's a lot of overhead.
The way dslibrary aims to help is by separating out all the information about a particular data source or target and providing ways to bundle and un-bundle it so that it can be sent where it is needed. The data science code itself should not have to worry about these settings or need to change just because the data moved or changed format.
The code often has access to those credentials. Maybe you trust the code not to "lift" those credentials and use them elsewhere, maybe you don't. Maybe you can ensure those credentials are locked down to no more than s3 bucket read access, or maybe you can't. Even secret management systems will still expose the credentials to the code.
The solution dslibrary facilitates is to have a different, trusted system perform the data access. In dslibrary there is an extensible/customizable way to "transport" data access to another system. By setting an environment variable or two (one for the remote URL, another for an access token), the data read and write operations can be managed by that other system. Before executing the code, one sends the URIs, credentials and file format information to the data access system.
The transport.to_rest
class will send dslibrary calls to a REST service.
The transport.to_volume
class will send dslibrary calls through a shared volume to a Kubernetes sidecar.
(c) Accenture 2021-2022
FAQs
Data Science Framework & Abstractions
We found that dslibrary demonstrated a healthy version release cadence and project activity because the last version was released less than a year ago. It has 1 open source maintainer collaborating on the project.
Did you know?
Socket for GitHub automatically highlights issues in each pull request and monitors the health of all your open source dependencies. Discover the contents of your packages and block harmful activity before you install or update your dependencies.
Security News
Research
The Socket Research Team breaks down a malicious wrapper package that uses obfuscation to harvest credentials and exfiltrate sensitive data.
Research
Security News
Attackers used a malicious npm package typosquatting a popular ESLint plugin to steal sensitive data, execute commands, and exploit developer systems.
Security News
The Ultralytics' PyPI Package was compromised four times in one weekend through GitHub Actions cache poisoning and failure to rotate previously compromised API tokens.