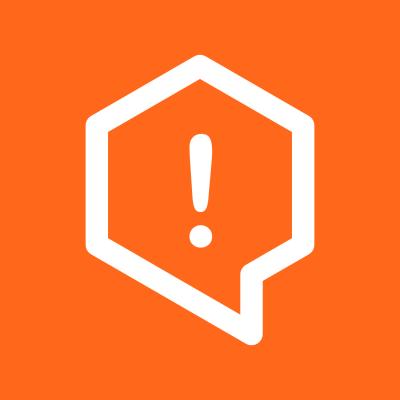
Security News
Fluent Assertions Faces Backlash After Abandoning Open Source Licensing
Fluent Assertions is facing backlash after dropping the Apache license for a commercial model, leaving users blindsided and questioning contributor rights.
.. image:: https://readthedocs.org/projects/embeddings/badge/?version=latest :target: http://embeddings.readthedocs.io/en/latest/?badge=latest :alt: Documentation Status .. image:: https://travis-ci.org/vzhong/embeddings.svg?branch=master :target: https://travis-ci.org/vzhong/embeddings
Embeddings is a python package that provides pretrained word embeddings for natural language processing and machine learning.
Instead of loading a large file to query for embeddings, embeddings
is backed by a database and fast to load and query:
.. code-block:: python
>>> %timeit GloveEmbedding('common_crawl_840', d_emb=300)
100 loops, best of 3: 12.7 ms per loop
>>> %timeit GloveEmbedding('common_crawl_840', d_emb=300).emb('canada')
100 loops, best of 3: 12.9 ms per loop
>>> g = GloveEmbedding('common_crawl_840', d_emb=300)
>>> %timeit -n1 g.emb('canada')
1 loop, best of 3: 38.2 µs per loop
.. code-block:: sh
pip install embeddings # from pypi
pip install git+https://github.com/vzhong/embeddings.git # from github
Upon first use, the embeddings are first downloaded to disk in the form of a SQLite database.
This may take a long time for large embeddings such as GloVe.
Further usage of the embeddings are directly queried against the database.
Embedding databases are stored in the $EMBEDDINGS_ROOT
directory (defaults to ~/.embeddings
). Note that this location is probably undesirable if your home directory is on NFS, as it would slow down database queries significantly.
.. code-block:: python
from embeddings import GloveEmbedding, FastTextEmbedding, KazumaCharEmbedding, ConcatEmbedding
g = GloveEmbedding('common_crawl_840', d_emb=300, show_progress=True)
f = FastTextEmbedding()
k = KazumaCharEmbedding()
c = ConcatEmbedding([g, f, k])
for w in ['canada', 'vancouver', 'toronto']:
print('embedding {}'.format(w))
print(g.emb(w))
print(f.emb(w))
print(k.emb(w))
print(c.emb(w))
If you use Docker, an image prepopulated with the Common Crawl 840 GloVe embeddings and Kazuma Hashimoto's character ngram embeddings is available at vzhong/embeddings <https://hub.docker.com/r/vzhong/embeddings>
_.
To mount volumes from this container, set $EMBEDDINGS_ROOT
in your container to /opt/embeddings
.
For example:
.. code-block:: bash
docker run --volumes-from vzhong/embeddings -e EMBEDDINGS_ROOT='/opt/embeddings' myimage python train.py
Pull requests welcome!
FAQs
Pretrained word embeddings in Python.
We found that embeddings demonstrated a healthy version release cadence and project activity because the last version was released less than a year ago. It has 1 open source maintainer collaborating on the project.
Did you know?
Socket for GitHub automatically highlights issues in each pull request and monitors the health of all your open source dependencies. Discover the contents of your packages and block harmful activity before you install or update your dependencies.
Security News
Fluent Assertions is facing backlash after dropping the Apache license for a commercial model, leaving users blindsided and questioning contributor rights.
Research
Security News
Socket researchers uncover the risks of a malicious Python package targeting Discord developers.
Security News
The UK is proposing a bold ban on ransomware payments by public entities to disrupt cybercrime, protect critical services, and lead global cybersecurity efforts.