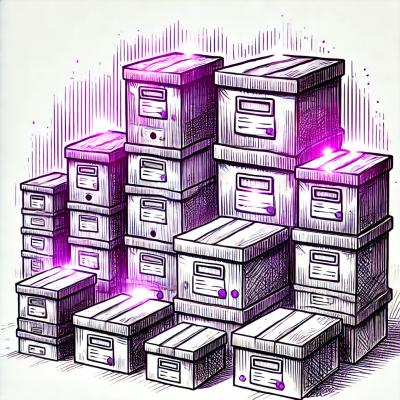
Security News
PyPI’s New Archival Feature Closes a Major Security Gap
PyPI now allows maintainers to archive projects, improving security and helping users make informed decisions about their dependencies.
Enchanter is a library for machine learning tasks for comet.ml users.
Getting Started • Docs • Tutorial • Licence
To get started, install PyTorch for your environment. Then install Enchanter in the following way:
To install the stable release.
pip install enchanter
or
To install the latest(unstable) release.
pip install git+https://github.com/khirotaka/enchanter.git
If you want to install with a specific branch, you can use the following.
# e.g.) Install enchanter from develop branch.
pip install git+https://github.com/khirotaka/enchanter.git@develop
Enchanter supports:
Try your first Enchanter Program. To train a neural network written in PyTorch on Enchanter, use the Runner
.
There are 2 ways to define a Runner
:
Runner
already implemented under enchanter.tasks
Runner
that inherit enchanter.engine.BaseRunner
.Let's see how to use the enchanter.tasks.ClassificationRunner
, which is the easiest way.
import comet_ml
import torch
import enchanter
model = torch.nn.Linear(6, 10)
optimizer = torch.optim.Adam(model.parameters())
runner = enchanter.tasks.ClassificationRunner(
model,
optimizer,
criterion=torch.nn.CrossEntropyLoss(),
experiment=comet_ml.Experiment()
)
runner.add_loader("train", train_loader)
runner.train_config(epochs=10)
runner.run()
Register a torch.utils.data.DataLoader
with the Runner
by using .add_loader()
.
Set up the number of epochs using .train_config()
, and execute Runner
with .run()
.
The wonderful algorithms for unsupervised time series representation learning, adopted at NeurIPS 2019, are now easily available.
Please prepare the following:
[N, F, L]
.2.
import comet_ml
import torch.nn as nn
import torch.optim as optim
import enchanter.tasks as tasks
import enchanter.addons.layers as L
class Encoder(nn.Module):
def __init__(self, in_features, mid_features, out_features):
super(Encoder, self).__init__()
self.conv = nn.Sequential(
L.CausalConv1d(in_features, mid_features, 3),
nn.LeakyReLU(),
L.CausalConv1d(mid_features, mid_features, 3),
nn.LeakyReLU(),
L.CausalConv1d(mid_features, mid_features, 3),
nn.LeakyReLU(),
nn.AdaptiveMaxPool1d(1)
)
self.fc = nn.Linear(mid_features, out_features)
def forward(self, x):
batch = x.shape[0]
out = self.conv(x).reshape(batch, -1)
return self.fc(out)
experiment = comet_ml.Experiment()
model = Encoder(...)
optimizer = optim.Adam(model.parameters())
runner = tasks.TimeSeriesUnsupervisedRunner(model, optimizer, experiment)
runner.add_loader("train", ...)
runner.run()
A teacher label is required for validation. Also, Use enchanter.callbacks.EarlyStoppingForTSUS
for early stopping.
from comet_ml import Optimizer
import torch
import torch.nn as nn
import torch.optim as optim
from sklearn.datasets import load_iris
import enchanter.tasks as tasks
import enchanter.addons as addons
import enchanter.addons.layers as layers
from enchanter.utils import comet
config = comet.TunerConfigGenerator(
algorithm="bayes",
metric="train_avg_loss",
objective="minimize",
seed=0,
trials=1,
max_combo=10
)
config.suggest_categorical("activation", ["addons.mish", "torch.relu", "torch.sigmoid"])
opt = Optimizer(config.generate())
x, y = load_iris(return_X_y=True)
x = x.astype("float32")
y = y.astype("int64")
for experiment in opt.get_experiments():
model = layers.MLP([4, 512, 128, 3], eval(experiment.get_parameter("activation")))
optimizer = optim.Adam(model.parameters())
runner = tasks.ClassificationRunner(
model, optimizer=optimizer, criterion=nn.CrossEntropyLoss(), experiment=experiment
)
runner.fit(x, y, epochs=1, batch_size=32)
runner.quite()
# or
# with runner:
# runner.fit(...)
# or
# runner.run()
Runners with defined in enchanter.tasks
are now support Auto Mixed Precision.
Write the following.
from torch.cuda import amp
from enchanter.tasks import ClassificationRunner
runner = ClassificationRunner(...)
runner.scaler = amp.GradScaler()
If you want to define a custom runner that supports mixed precision, do the following.
from torch.cuda import amp
import torch.nn.functional as F
from enchanter.engine import BaseRunner
class CustomRunner(BaseRunner):
# ...
def train_step(self, batch):
x, y = batch
with amp.autocast(): # REQUIRED
out = self.model(x)
loss = F.nll_loss(out, y)
return {"loss": loss}
runner = CustomRunner(...)
runner.scaler = amp.GradScaler()
That is, you can enable AMP by using torch.cuda.amp.autocast()
in .train_step()
, .val_step()
and .test_step()
.
from comet_ml import Experiment
import torch
import torch.nn as nn
import torch.optim as optim
from torch.utils.data import DataLoader
from sklearn.datasets import load_iris
from tqdm.auto import tqdm
import enchanter.tasks as tasks
import enchanter.engine.modules as modules
import enchanter.addons as addons
import enchanter.addons.layers as layers
experiment = Experiment()
model = layers.MLP([4, 512, 128, 3], addons.mish)
optimizer = optim.Adam(model.parameters())
x, y = load_iris(return_X_y=True)
x = x.astype("float32")
y = y.astype("int64")
train_ds = modules.get_dataset(x, y)
val_ds = modules.get_dataset(x, y)
test_ds = modules.get_dataset(x, y)
train_loader = DataLoader(train_ds, batch_size=32)
val_loader = DataLoader(val_ds, batch_size=32)
test_loader = DataLoader(test_ds, batch_size=32)
runner = tasks.ClassificationRunner(
model, optimizer, nn.CrossEntropyLoss(), experiment
)
with runner:
for epoch in tqdm(range(10)):
with runner.experiment.train():
for train_batch in train_loader:
runner.optimizer.zero_grad()
train_out = runner.train_step(train_batch)
runner.backward(train_out["loss"])
runner.update_optimizer()
with runner.experiment.validate(), torch.no_grad():
for val_batch in val_loader:
val_out = runner.val_step(val_batch)["loss"]
runner.experiment.log_metric("val_loss", val_out)
with runner.experiment.test(), torch.no_grad():
for test_batch in test_loader:
test_out = runner.test_step(test_batch)["loss"]
runner.experiment.log_metric("test_loss", test_out)
# The latest checkpoints (model_state & optim_state) are stored
# in comet.ml after the with statement.
import torch
from enchanter.utils import visualize
from enchanter.addons.layers import AutoEncoder
x = torch.randn(1, 32) # [N, in_features]
model = AutoEncoder([32, 16, 8, 2])
visualize.with_netron(model, (x, ))
FAQs
Enchanter is a library for machine learning tasks for comet.ml users.
We found that enchanter demonstrated a healthy version release cadence and project activity because the last version was released less than a year ago. It has 1 open source maintainer collaborating on the project.
Did you know?
Socket for GitHub automatically highlights issues in each pull request and monitors the health of all your open source dependencies. Discover the contents of your packages and block harmful activity before you install or update your dependencies.
Security News
PyPI now allows maintainers to archive projects, improving security and helping users make informed decisions about their dependencies.
Research
Security News
Malicious npm package postcss-optimizer delivers BeaverTail malware, targeting developer systems; similarities to past campaigns suggest a North Korean connection.
Security News
CISA's KEV data is now on GitHub, offering easier access, API integration, commit history tracking, and automated updates for security teams and researchers.