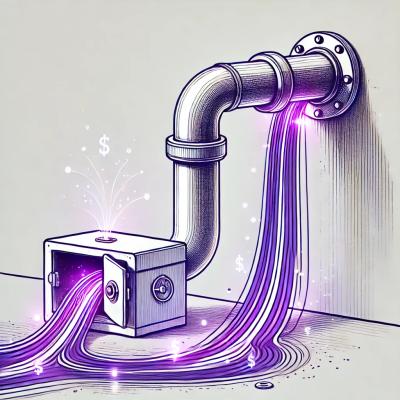
Research
Security News
Malicious npm Package Targets Solana Developers and Hijacks Funds
A malicious npm package targets Solana developers, rerouting funds in 2% of transactions to a hardcoded address.
English | 简体中文
⭐ If you like this project, please click the "Star" button at the top right to support us. Your support is our motivation to keep going!
EvalScope is the official model evaluation and performance benchmarking framework launched by the ModelScope community. It comes with built-in common benchmarks and evaluation metrics, such as MMLU, CMMLU, C-Eval, GSM8K, ARC, HellaSwag, TruthfulQA, MATH, and HumanEval. EvalScope supports various types of model evaluations, including LLMs, multimodal LLMs, embedding models, and reranker models. It is also applicable to multiple evaluation scenarios, such as end-to-end RAG evaluation, arena mode, and model inference performance stress testing. Moreover, with the seamless integration of the ms-swift training framework, evaluations can be initiated with a single click, providing full end-to-end support from model training to evaluation 🚀
EvalScope Framework.
The architecture includes the following modules:
llmuses
has been changed to evalscope
. Please update your code accordingly.We recommend using conda to manage your environment and installing dependencies with pip:
Create a conda environment (optional)
# It is recommended to use Python 3.10
conda create -n evalscope python=3.10
# Activate the conda environment
conda activate evalscope
Install dependencies using pip
pip install evalscope # Install Native backend (default)
# Additional options
pip install evalscope[opencompass] # Install OpenCompass backend
pip install evalscope[vlmeval] # Install VLMEvalKit backend
pip install evalscope[rag] # Install RAGEval backend
pip install evalscope[perf] # Install Perf dependencies
pip install evalscope[all] # Install all backends (Native, OpenCompass, VLMEvalKit, RAGEval)
[!WARNING] As the project has been renamed to
evalscope
, for versionsv0.4.3
or earlier, you can install using the following command:pip install llmuses<=0.4.3
To import relevant dependencies using
llmuses
:
from llmuses import ...
Download the source code
git clone https://github.com/modelscope/evalscope.git
Install dependencies
cd evalscope/
pip install -e . # Install Native backend
# Additional options
pip install -e '.[opencompass]' # Install OpenCompass backend
pip install -e '.[vlmeval]' # Install VLMEvalKit backend
pip install -e '.[rag]' # Install RAGEval backend
pip install -e '.[perf]' # Install Perf dependencies
pip install -e '.[all]' # Install all backends (Native, OpenCompass, VLMEvalKit, RAGEval)
To evaluate a model using default settings on specified datasets, follow the process below:
You can execute this in any directory:
python -m evalscope.run \
--model Qwen/Qwen2.5-0.5B-Instruct \
--template-type qwen \
--datasets gsm8k ceval \
--limit 10
You need to execute this in the evalscope
directory:
python evalscope/run.py \
--model Qwen/Qwen2.5-0.5B-Instruct \
--template-type qwen \
--datasets gsm8k ceval \
--limit 10
If prompted with
Do you wish to run the custom code? [y/N]
, please typey
.
Results (tested with only 10 samples)
Report table:
+-----------------------+--------------------+-----------------+
| Model | ceval | gsm8k |
+=======================+====================+=================+
| Qwen2.5-0.5B-Instruct | (ceval/acc) 0.5577 | (gsm8k/acc) 0.5 |
+-----------------------+--------------------+-----------------+
--model
: Specifies the model_id
of the model on ModelScope, allowing automatic download. For example, see the Qwen2-0.5B-Instruct model link; you can also use a local path, such as /path/to/model
.--template-type
: Specifies the template type corresponding to the model. Refer to the Default Template
field in the template table for filling in this field.--datasets
: The dataset name, allowing multiple datasets to be specified, separated by spaces; these datasets will be automatically downloaded. Refer to the supported datasets list for available options.--limit
: Maximum number of evaluation samples per dataset; if not specified, all will be evaluated, which is useful for quick validation.If you wish to conduct a more customized evaluation, such as modifying model parameters or dataset parameters, you can use the following commands:
Example 1:
python evalscope/run.py \
--model qwen/Qwen2-0.5B-Instruct \
--template-type qwen \
--model-args revision=master,precision=torch.float16,device_map=auto \
--datasets gsm8k ceval \
--use-cache true \
--limit 10
Example 2:
python evalscope/run.py \
--model qwen/Qwen2-0.5B-Instruct \
--template-type qwen \
--generation-config do_sample=false,temperature=0.0 \
--datasets ceval \
--dataset-args '{"ceval": {"few_shot_num": 0, "few_shot_random": false}}' \
--limit 10
In addition to the three basic parameters, the other parameters are as follows:
--model-args
: Model loading parameters, separated by commas, in key=value
format.--generation-config
: Generation parameters, separated by commas, in key=value
format.
do_sample
: Whether to use sampling, default is false
.max_new_tokens
: Maximum generation length, default is 1024.temperature
: Sampling temperature.top_p
: Sampling threshold.top_k
: Sampling threshold.--use-cache
: Whether to use local cache, default is false
. If set to true
, previously evaluated model and dataset combinations will not be evaluated again, and will be read directly from the local cache.--dataset-args
: Evaluation dataset configuration parameters, provided in JSON format, where the key is the dataset name and the value is the parameter; note that these must correspond one-to-one with the values in --datasets
.
--few_shot_num
: Number of few-shot examples.--few_shot_random
: Whether to randomly sample few-shot data; if not specified, defaults to true
.Using the run_task
function to submit an evaluation task requires the same parameters as the command line. You need to pass a dictionary as the parameter, which includes the following fields:
import torch
from evalscope.constants import DEFAULT_ROOT_CACHE_DIR
# Example
your_task_cfg = {
'model_args': {'revision': None, 'precision': torch.float16, 'device_map': 'auto'},
'generation_config': {'do_sample': False, 'repetition_penalty': 1.0, 'max_new_tokens': 512},
'dataset_args': {},
'dry_run': False,
'model': 'qwen/Qwen2-0.5B-Instruct',
'template_type': 'qwen',
'datasets': ['arc', 'hellaswag'],
'work_dir': DEFAULT_ROOT_CACHE_DIR,
'outputs': DEFAULT_ROOT_CACHE_DIR,
'mem_cache': False,
'dataset_hub': 'ModelScope',
'dataset_dir': DEFAULT_ROOT_CACHE_DIR,
'limit': 10,
'debug': False
}
Here, DEFAULT_ROOT_CACHE_DIR
is set to '~/.cache/evalscope'
.
from evalscope.run import run_task
run_task(task_cfg=your_task_cfg)
EvalScope supports using third-party evaluation frameworks to initiate evaluation tasks, which we call Evaluation Backend. Currently supported Evaluation Backend includes:
A stress testing tool focused on large language models, which can be customized to support various dataset formats and different API protocol formats.
Reference: Performance Testing 📖 User Guide
Supports wandb for recording results
Supports Speed Benchmark
It supports speed testing and provides speed benchmarks similar to those found in the official Qwen reports:
Speed Benchmark Results:
+---------------+-----------------+----------------+
| Prompt Tokens | Speed(tokens/s) | GPU Memory(GB) |
+---------------+-----------------+----------------+
| 1 | 50.69 | 0.97 |
| 6144 | 51.36 | 1.23 |
| 14336 | 49.93 | 1.59 |
| 30720 | 49.56 | 2.34 |
+---------------+-----------------+----------------+
EvalScope supports custom dataset evaluation. For detailed information, please refer to the Custom Dataset Evaluation 📖User Guide
You can use local dataset to evaluate the model without internet connection.
Refer to: Offline Evaluation 📖 User Guide
The Arena mode allows multiple candidate models to be evaluated through pairwise battles, and can choose to use the AI Enhanced Auto-Reviewer (AAR) automatic evaluation process or manual evaluation to obtain the evaluation report.
Refer to: Arena Mode 📖 User Guide
FAQs
EvalScope: Lightweight LLMs Evaluation Framework
We found that evalscope demonstrated a healthy version release cadence and project activity because the last version was released less than a year ago. It has 1 open source maintainer collaborating on the project.
Did you know?
Socket for GitHub automatically highlights issues in each pull request and monitors the health of all your open source dependencies. Discover the contents of your packages and block harmful activity before you install or update your dependencies.
Research
Security News
A malicious npm package targets Solana developers, rerouting funds in 2% of transactions to a hardcoded address.
Security News
Research
Socket researchers have discovered malicious npm packages targeting crypto developers, stealing credentials and wallet data using spyware delivered through typosquats of popular cryptographic libraries.
Security News
Socket's package search now displays weekly downloads for npm packages, helping developers quickly assess popularity and make more informed decisions.