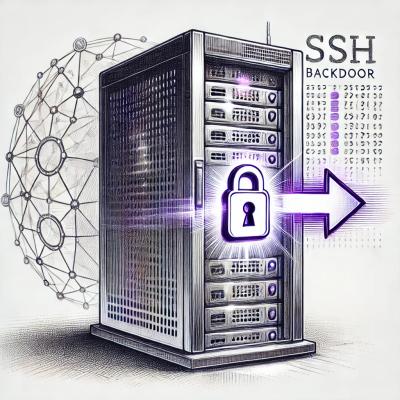
Research
Security News
Malicious npm Packages Inject SSH Backdoors via Typosquatted Libraries
Socket’s threat research team has detected six malicious npm packages typosquatting popular libraries to insert SSH backdoors.
Fast Run-Eval-Polish Loop for LLM Applications.
This project is still in the early development stage. Have questions? Let's chat!
Let's say we have this existing system:
import openai
context = """
The first step is to decide what to work on. The work you choose needs to have three qualities: it has to be something you have a natural aptitude for, that you have a deep interest in, and that offers scope to do great work.
In practice you don't have to worry much about the third criterion. Ambitious people are if anything already too conservative about it. So all you need to do is find something you have an aptitude for and great interest in.
"""
def run_qa(question: str) -> str:
return openai.ChatCompletion.create(
model="gpt-3.5-turbo",
messages=[
{
"role": "system",
"content": f"Answer in less than 30 words. Use the following context if needed: {context}",
},
{"role": "user", "content": question},
],
)["choices"][0]["message"]["content"]
We already have a fixed context. Now, let's ask some questions. local_runner
is used here to run it locally with threads and progress tracking. We will have remote_runner
to run the same in the cloud.
contexts = [[context]] * len(questions)
# https://huggingface.co/datasets/repllabs/questions_how_to_do_great_work
questions = [
"how to do great work?.",
"How can curiosity be nurtured and utilized to drive great work?",
"How does the author suggest finding something to work on?",
"How did Van Dyck's painting differ from Daniel Mytens' version and what message did it convey?",
]
runner = fastrepl.local_runner(fn=run_qa)
ds = runner.run(args_list=[(q,) for q in questions], output_feature="answer")
ds = ds.add_column("question", questions)
ds = ds.add_column("contexts", contexts)
# fastrepl.Dataset({
# features: ['answer', 'question', 'contexts'],
# num_rows: 4
# })
Now, let's use one of our evaluators to evaluate the dataset. Note that we are running it 5 times to ensure we obtain consistent results.
evaluator = fastrepl.RAGEvaluator(node=fastrepl.RAGAS(metric="Faithfulness"))
ds = fastrepl.local_runner(evaluator=evaluator, dataset=ds).run(num=5)
# ds["result"]
# [[0.25, 0.0, 0.25, 0.25, 0.5],
# [0.5, 0.5, 0.5, 0.75, 0.875],
# [0.66, 0.66, 0.66, 0.66, 0.66],
# [1.0, 1.0, 1.0, 1.0, 1.0]]
Seems like we are getting quite good results. If we increase the number of samples a bit, we can obtain a reliable evaluation of the entire system. We will keep working on bringing better evaluations.
Detailed documentation is here.
Any kind of contribution is welcome.
FAQs
Fast Run-Eval-Polish Loop for LLM App
We found that fastrepl demonstrated a healthy version release cadence and project activity because the last version was released less than a year ago. It has 1 open source maintainer collaborating on the project.
Did you know?
Socket for GitHub automatically highlights issues in each pull request and monitors the health of all your open source dependencies. Discover the contents of your packages and block harmful activity before you install or update your dependencies.
Research
Security News
Socket’s threat research team has detected six malicious npm packages typosquatting popular libraries to insert SSH backdoors.
Security News
MITRE's 2024 CWE Top 25 highlights critical software vulnerabilities like XSS, SQL Injection, and CSRF, reflecting shifts due to a refined ranking methodology.
Security News
In this segment of the Risky Business podcast, Feross Aboukhadijeh and Patrick Gray discuss the challenges of tracking malware discovered in open source softare.