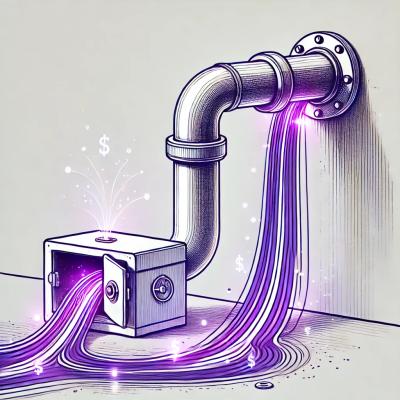
Research
Security News
Malicious npm Package Targets Solana Developers and Hijacks Funds
A malicious npm package targets Solana developers, rerouting funds in 2% of transactions to a hardcoded address.
Flatbread is a small library which extends the pivot table functionality in pandas. Flatbread is accessible through the DataFrame using the pita
accessor.
The library contains functions which will allow you to easily add totals/subtotals to one or more axes/levels of your pivot table. Furthermore, flatbread can calculate percentages from the totals/subtotals of each axis/level of your pivot table. You can transform the existing values in your table into percentages, but you can also add the percentages neatly next to your data. If the required (sub)totals are not present, then flatbread will add them automatically in order to perform the calculations. By default the (sub)totals are kept but you can drop them too. The library also contains some functionality built on top of matplotlib for plotting your data.
Initially I planned for this library to be called pita -- short for pivot tables. But as that name was already taken on pypi.org the choice fell on flatbread.
To install:
pip install flatbread
Let's create a df for testing:
from random import randint
import pandas as pd
import flatbread as fb
df = pd._testing.makeCustomDataframe(
nrows=8,
ncols=4,
data_gen_f=lambda r,c:randint(1,100),
c_idx_nlevels=2,
r_idx_nlevels=3,
c_ndupe_l=[2,1],
r_ndupe_l=[4,2,1],
)
Flatbread let's you easily add subtotals to your pivot tables. Here we add totals and subtotals to both axes at once:
df.pipe(fb.totals.add, axis=2, level=[0,1])
C0 | C_l0_g0 | C_l0_g1 | Total | ||||||
---|---|---|---|---|---|---|---|---|---|
C1 | C_l1_g0 | C_l1_g1 | Subtotal | C_l1_g2 | C_l1_g3 | Subtotal | |||
R0 | R1 | R2 | |||||||
R_l0_g0 | R_l1_g0 | R_l2_g0 | 8 | 76 | 84 | 32 | 55 | 87 | 171 |
R_l2_g1 | 21 | 75 | 96 | 15 | 67 | 82 | 178 | ||
R_l1_g1 | R_l2_g2 | 66 | 84 | 150 | 38 | 40 | 78 | 228 | |
R_l2_g3 | 83 | 94 | 177 | 57 | 31 | 88 | 265 | ||
Subtotal | 178 | 329 | 507 | 142 | 193 | 335 | 842 | ||
R_l0_g1 | R_l1_g2 | R_l2_g4 | 32 | 82 | 114 | 55 | 87 | 142 | 256 |
R_l2_g5 | 68 | 22 | 90 | 100 | 70 | 170 | 260 | ||
R_l1_g3 | R_l2_g6 | 55 | 25 | 80 | 40 | 24 | 64 | 144 | |
R_l2_g7 | 12 | 80 | 92 | 31 | 79 | 110 | 202 | ||
Subtotal | 167 | 209 | 376 | 226 | 260 | 486 | 862 | ||
Total | 345 | 538 | 883 | 368 | 453 | 821 | 1704 |
Flatbread let's you calculate the percentages of the totals or subtotals. You can either transform the data itself or add the percentages into your pivot table as separate columns. When rounding the percentages they will always add up to 100%:
df.pipe(fb.percs.add, level=1)
C0 | C_l0_g0 | C_l0_g1 | ||||||||
---|---|---|---|---|---|---|---|---|---|---|
C1 | C_l1_g0 | C_l1_g1 | C_l1_g2 | C_l1_g3 | ||||||
abs | % | abs | % | abs | % | abs | % | |||
R0 | R1 | R2 | ||||||||
R_l0_g0 | R_l1_g0 | R_l2_g0 | 8 | 4.5 | 76 | 23.1 | 32 | 22.5 | 55 | 28.5 |
R_l2_g1 | 21 | 11.8 | 75 | 22.8 | 15 | 10.6 | 67 | 34.7 | ||
R_l1_g1 | R_l2_g2 | 66 | 37.1 | 84 | 25.5 | 38 | 26.8 | 40 | 20.7 | |
R_l2_g3 | 83 | 46.6 | 94 | 28.6 | 57 | 40.1 | 31 | 16.1 | ||
Subtotal | 178 | 100.0 | 329 | 100.0 | 142 | 100.0 | 193 | 100.0 | ||
R_l0_g1 | R_l1_g2 | R_l2_g4 | 32 | 19.2 | 82 | 39.2 | 55 | 24.3 | 87 | 33.5 |
R_l2_g5 | 68 | 40.7 | 22 | 10.6 | 100 | 44.3 | 70 | 26.9 | ||
R_l1_g3 | R_l2_g6 | 55 | 32.9 | 25 | 11.9 | 40 | 17.7 | 24 | 9.2 | |
R_l2_g7 | 12 | 7.2 | 80 | 38.3 | 31 | 13.7 | 79 | 30.4 | ||
Subtotal | 167 | 100.0 | 209 | 100.0 | 226 | 100.0 | 260 | 100.0 |
Flatbread provides the format
function for rendering your pivot table according to your preferred locale. Here we use nl-NL
as an example:
df = pd._testing.makeCustomDataframe(
nrows=5,
ncols=4,
data_gen_f=lambda r,c:randint(10,1000),
)
df.pipe(fb.percs.add).pipe(fb.format)
C0 | C_l0_g0 | C_l0_g1 | C_l0_g2 | C_l0_g3 | ||||
---|---|---|---|---|---|---|---|---|
abs | % | abs | % | abs | % | abs | % | |
R0 | ||||||||
R_l0_g0 | 702 | 23,8 | 57 | 1,7 | 579 | 23,2 | 908 | 39,6 |
R_l0_g1 | 791 | 26,8 | 839 | 25,6 | 687 | 27,6 | 333 | 14,5 |
R_l0_g2 | 579 | 19,6 | 777 | 23,7 | 633 | 25,4 | 553 | 24,2 |
R_l0_g3 | 571 | 19,3 | 699 | 21,3 | 108 | 4,4 | 439 | 19,1 |
R_l0_g4 | 310 | 10,5 | 908 | 27,7 | 484 | 19,4 | 59 | 2,6 |
Total | 2.953 | 100,0 | 3.280 | 100,0 | 2.491 | 100,0 | 2.292 | 100,0 |
Flatbread let's you control most of its behavior through key-word arguments, but it is also easy to store your settings and use them globally throughout a project:
from flatbread import CONFIG
# pick your preferred locale and set it (used with `format`)
CONFIG.format['locale'] = 'nl_NL'
CONFIG.set_locale()
# set your own labels
CONFIG.aggregation['totals_name'] = 'Totes'
CONFIG.aggregation['label_rel'] = 'pct'
# define the number of digits to round to (-1 is no rounding)
CONFIG.aggregation['ndigits] = 2
# store your configuration permanently (across sessions)
CONFIG.save()
# restore your settings to 'factory' defaults
CONFIG.factory_reset()
Use the Trendline object to create trendlines. Compare multiple years:
tl = fb.TrendLine.from_df(
sample,
offset_year = 2019,
datefield = 'Date of Application',
yearfield = 'Academic Year',
period = 'w',
end = '2019-09-01',
grouper = 'Academic Year',
focus = 2019,
)
fig = tl.plot()
Split your graphs in rows and columns:
tl = fb.TrendLine.from_df(
sample,
offset_year = 2019,
datefield = 'Date Processed',
yearfield = 'Academic Year',
period = 'w',
end = '2019-10-01',
grouper = 'Faculty',
focus = 'Humanities',
)
fig = tl.plot(
rows = 'Origin Country',
cols = 'Examination Type',
cum = True,
filter = "`Academic Year` == 2019"
)
FAQs
Pivot tables and graphs for pandas
We found that flatbread demonstrated a healthy version release cadence and project activity because the last version was released less than a year ago. It has 1 open source maintainer collaborating on the project.
Did you know?
Socket for GitHub automatically highlights issues in each pull request and monitors the health of all your open source dependencies. Discover the contents of your packages and block harmful activity before you install or update your dependencies.
Research
Security News
A malicious npm package targets Solana developers, rerouting funds in 2% of transactions to a hardcoded address.
Security News
Research
Socket researchers have discovered malicious npm packages targeting crypto developers, stealing credentials and wallet data using spyware delivered through typosquats of popular cryptographic libraries.
Security News
Socket's package search now displays weekly downloads for npm packages, helping developers quickly assess popularity and make more informed decisions.