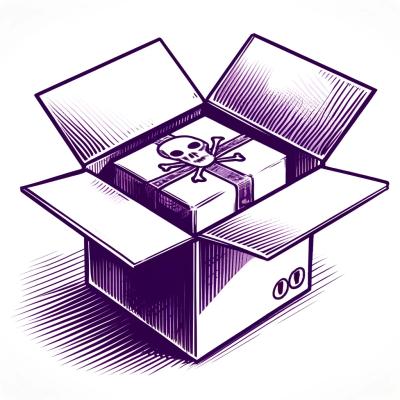
Security News
Research
Data Theft Repackaged: A Case Study in Malicious Wrapper Packages on npm
The Socket Research Team breaks down a malicious wrapper package that uses obfuscation to harvest credentials and exfiltrate sensitive data.
Flower Datasets (flwr-datasets
) is a library to quickly and easily create datasets for federated learning, federated evaluation, and federated analytics. It was created by the Flower Labs
team that also created Flower: A Friendly Federated Learning Framework.
[!TIP] For complete documentation that includes API docs, how-to guides and tutorials, please visit the Flower Datasets Documentation and for full FL example see the Flower Examples page.
For a complete installation guide visit the Flower Datasets Documentation
pip install flwr-datasets[vision]
Flower Datasets library supports:
datasets
,Thanks to using Hugging Face's datasets
used under the hood, Flower Datasets integrates with the following popular formats/frameworks:
Create custom partitioning schemes or choose from the implemented partitioning schemes:
Partitioner
IidPartitioner(num_partitions)
DirichletPartitioner(num_partitions, partition_by, alpha)
DistributionPartitioner(distribution_array, num_partitions, num_unique_labels_per_partition, partition_by, preassigned_num_samples_per_label, rescale)
InnerDirichletPartitioner(partition_sizes, partition_by, alpha)
PathologicalPartitioner(num_partitions, partition_by, num_classes_per_partition, class_assignment_mode)
NaturalIdPartitioner(partition_by)
SizePartitioner
LinearPartitioner(num_partitions)
SquarePartitioner(num_partitions)
ExponentialPartitioner(num_partitions)
Comparison of Partitioning Schemes on CIFAR10
PS: This plot was generated using a library function (see flwr_datasets.visualization package for more).
Flower Datasets exposes the FederatedDataset
abstraction to represent the dataset needed for federated learning/evaluation/analytics. It has two powerful methods that let you handle the dataset preprocessing: load_partition(partition_id, split)
and load_split(split)
.
Here's a basic quickstart example of how to partition the MNIST dataset:
from flwr_datasets import FederatedDataset
from flwr_datasets.partitioners import IidPartitioner
# The train split of the MNIST dataset will be partitioned into 100 partitions
partitioner = IidPartitioner(num_partitions=100)
fds = FederatedDataset("ylecun/mnist", partitioners={"train": partitioner})
partition = fds.load_partition(0)
centralized_data = fds.load_split("test")
For more details, please refer to the specific how-to guides or tutorial. They showcase customization and more advanced features.
Here are a few of the things that we will work on in future releases:
Partitioner
s.Partitioner
s.Partitioner
s via FederatedDataset
's partitioners
argument.FAQs
Flower Datasets
We found that flwr-datasets demonstrated a healthy version release cadence and project activity because the last version was released less than a year ago. It has 2 open source maintainers collaborating on the project.
Did you know?
Socket for GitHub automatically highlights issues in each pull request and monitors the health of all your open source dependencies. Discover the contents of your packages and block harmful activity before you install or update your dependencies.
Security News
Research
The Socket Research Team breaks down a malicious wrapper package that uses obfuscation to harvest credentials and exfiltrate sensitive data.
Research
Security News
Attackers used a malicious npm package typosquatting a popular ESLint plugin to steal sensitive data, execute commands, and exploit developer systems.
Security News
The Ultralytics' PyPI Package was compromised four times in one weekend through GitHub Actions cache poisoning and failure to rotate previously compromised API tokens.